INTERPRETABLE DEEP MODEL FOR PREDICTING GENE-ADDICTED NON-SMALL-CELL LUNG CANCER IN CT SCANS
2021 IEEE 18TH INTERNATIONAL SYMPOSIUM ON BIOMEDICAL IMAGING (ISBI)(2021)
摘要
Genetic profiling and characterization of lung cancers have recently emerged as a new technique for targeted therapeutic treatment based on inununotherapy or molecular drugs. However, the most effective way to discover specific gene mutations through tissue biopsy has several limitations, from invasiveness to being a risky procedure. Recently, quantitative assessment of visual features from CT data has been demonstrated to be a valid alternative to biopsy for the diagnosis of gene-addicted tumors. In this paper, we present a deep model for automated lesion segmentation and classification as gene-addicted or not. The segmentation approach extends the 2D Tiramisu architecture for 3D segmentation through dense blocks and squeeze-and-excitation layers, while a multi-scale 3D CNN is used for lesion classification. We also train our model with adversarial samples, and show that this approach acts as a gradient regularizer and enhances model interpretability. We also built a dataset, the first of its nature, consisting of 73 CT scans annotated with the presence of a specific genomics profile. We test our approach on this dataset achieving a segmentation accuracy of 93.11% (Dice score) and a classification accuracy in identifying oncogene-addicted lung tumors of 82.00%.
更多查看译文
关键词
XAI, 3D Tiramisu, Lesion Classification
AI 理解论文
溯源树
样例
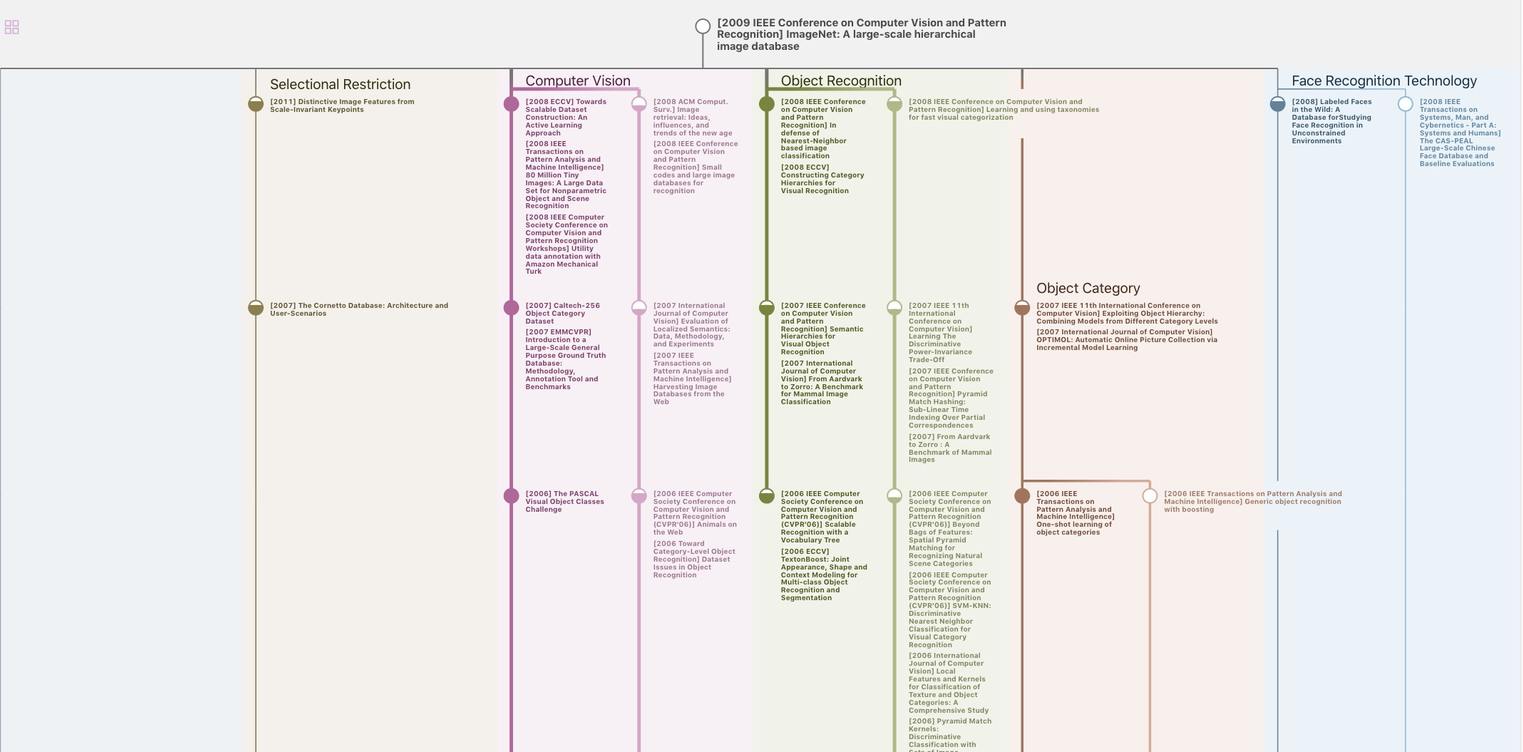
生成溯源树,研究论文发展脉络
Chat Paper
正在生成论文摘要