CONNECTOME-BASED PREDICTIVE MODELLING WITH MISSING CONNECTIVITY DATA USING ROBUST MATRIX COMPLETION
2021 IEEE 18TH INTERNATIONAL SYMPOSIUM ON BIOMEDICAL IMAGING (ISBI)(2021)
Abstract
Combining connectivity data from multiple tasks incorporates complementary information and increases the predictive power of machine learning models. However, as the number of tasks increases, the chances of missing a portion of data also increases, hindering downstream analyses. The most common strategy for handling missing data is to remove participants with missing data and run the analysis only using the complete cases. Though convenient, this approach discards a huge amount of useful information and leaves a smaller subset of data for predictive modelling. To address this issue, we embed Robust Matrix Completion after the feature selection step in the Connectome-based Predictive Modelling (CPM) pipeline so that the participants with missing data can be included. The experimental results on the HCP dataset show that our proposed method significantly improves the predictive performance and outperforms other comparison methods.
MoreTranslated text
Key words
missing data, matrix completion, data imputation, functional connectivity
AI Read Science
Must-Reading Tree
Example
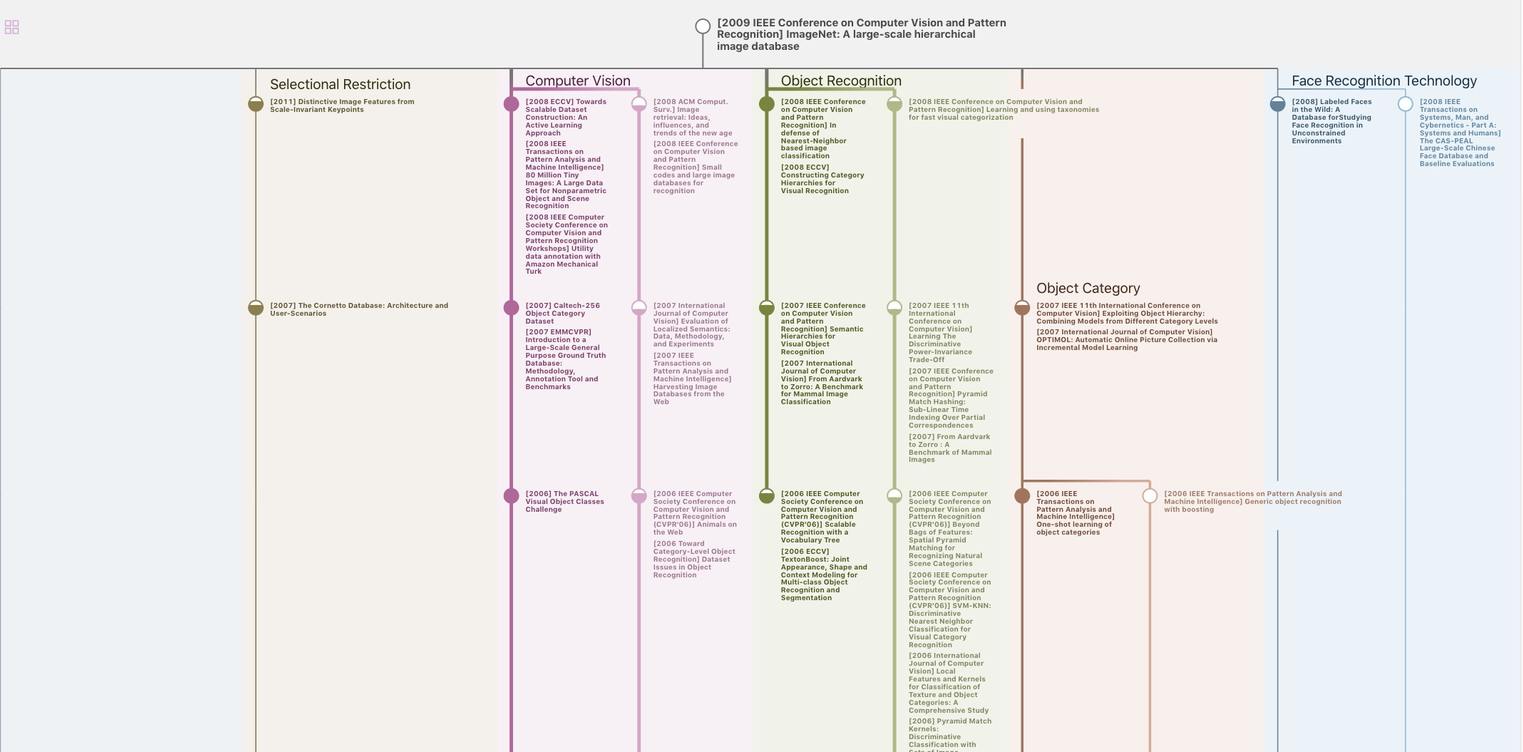
Generate MRT to find the research sequence of this paper
Chat Paper
Summary is being generated by the instructions you defined