HIGHLY ACCURATE AUTOMATED PATIENT-SPECIFIC 3D BONE POSE AND SCALE ESTIMATION USING BI-PLANAR POSE-INVARIANT PATCHES IN A CNN-BASED 3D/2D REGISTRATION FRAMEWORK
2021 IEEE 18TH INTERNATIONAL SYMPOSIUM ON BIOMEDICAL IMAGING (ISBI)(2021)
摘要
This paper proposes an automatic CNN-based 3D/2D registration method to achieve highly accurate and robust seven degrees of freedom (7DOF) pose and isotropic scale of a generic 3D model. This step is a key enabler for reconstructing a patient-specific 3D bone surface model from a wide range of EOS (R) 2D bi-planar X-rays acquired with various fields of view and patients' orientations. Based on a coarse-to-fine strategy. first a CNN-based semantic segmentation followed by a PCA-based registration are used to roughly locate the bone. Similarity in pose-invariant local patches using CNN regression models is used to refine the 3D pose. The accuracy of the method is validated on 60 bi-planar X-rays. The mean of Mean Absolute pose Errors (MAE) of 3D translations, 3D rotations, and isotropic scaling are 0.19 mm, 0.33 degrees, and 0.05(%), respectively. The success rate is of 100 % at MAE lower than 1 mm. 1 degrees. and 0.1 (%).
更多查看译文
关键词
7DOF registration, convolutional neural networks (CNN), 3D/2D similarity registration, 2D bi-planar X-rays
AI 理解论文
溯源树
样例
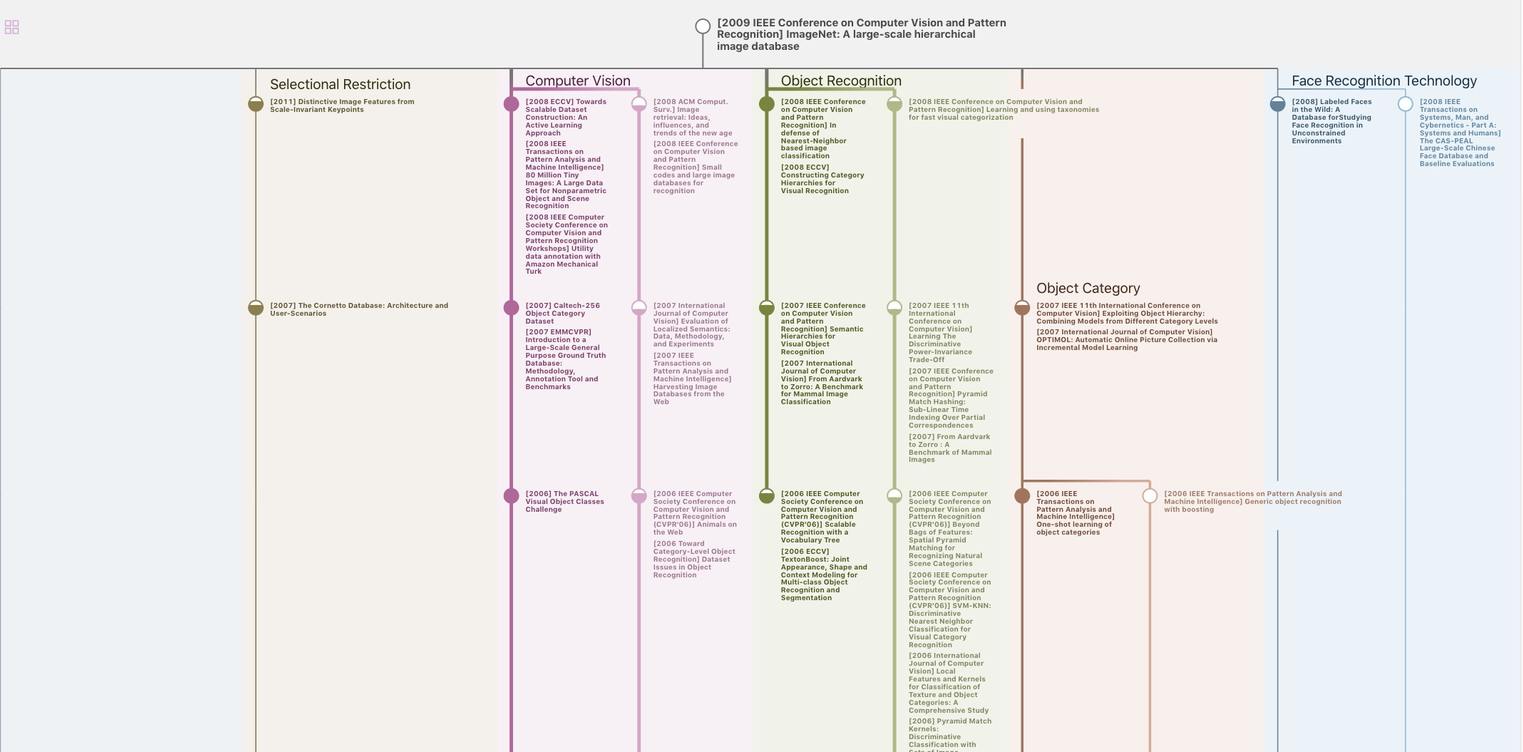
生成溯源树,研究论文发展脉络
Chat Paper
正在生成论文摘要