GHOST-LIGHT-3DNET: EFFICIENT NETWORK FOR HEART SEGMENTATION
2021 IEEE 18TH INTERNATIONAL SYMPOSIUM ON BIOMEDICAL IMAGING (ISBI)(2021)
摘要
Accurate 3D whole heart segmentation provides more details of the morphological and pathological information that could help doctors with more effective patient-specific treatments. 3D CNN network has been recognized as an important role in accurate volumetric segmentation. Typically, 3D CNN network has a large number of parameters as well as the floating point operations (FLOPs), which leads to heavy and complex computation. In this paper, we introduce an efficient 3D network (Ghost-Light-3DNet) for heart segmentation. Our solution is characterized by two key components: First, inspired by Ghos tNet in 2D, we extend the Ghost module to 3D which can generate more feature maps from cheap operations. Second, a sequential separable cony with residual module is applied as a light plug-and-play component to further reduce network parameters and FLOPs. For evaluation, the proposed method is validated on the MM-WHS heart segmentation Challenge 2017 datasets. Compared to state-of-the-art solution using 3D UNet-like architecture, our Ghost-Light-3DNet achieves comparable segmentation accuracy with the 2.18x fewer parameters and 4.48x less FLOPs, respectively.
更多查看译文
关键词
Heart segmentation, 3D efficient CNN network, net work architecture
AI 理解论文
溯源树
样例
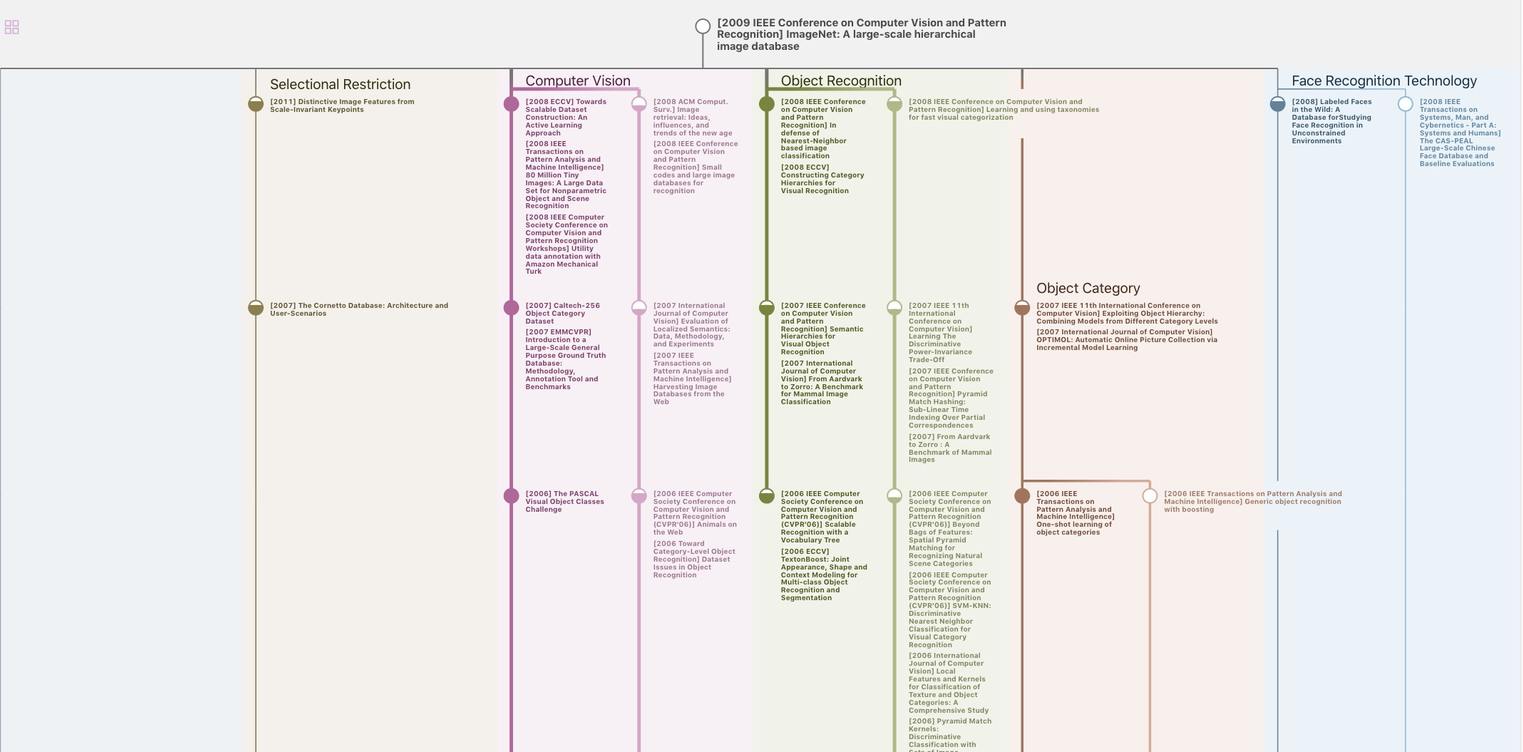
生成溯源树,研究论文发展脉络
Chat Paper
正在生成论文摘要