DEEP BAYESIAN IMAGE SEGMENTATION FOR A MORE ROBUST EJECTION FRACTION ESTIMATION
2021 IEEE 18TH INTERNATIONAL SYMPOSIUM ON BIOMEDICAL IMAGING (ISBI)(2021)
Abstract
The left ventricular ejection fraction (LVEF) is one of the most commonly measured cardiac parameters in echocardiography (echo). While the clinical guidelines suggest that apical views are used for LVEF assessment, these views are difficult to obtain for less experienced operators. Specifically, in point-of-care imaging, parasternal views are commonly used for rapid assessment of LVEF, since those views can be easier to obtain. However, robust LVEF estimation is challenging due to high variability of echo quality and cardiovascular structures across different patients. In this paper, we formulate a Bayesian deep learning approach for fully automatic LVEF estimation based on segmentation of the left ventricle (LV) in parasternal short-axis papillary muscles (PSAX-PM) level. The proposed approach exploits the LV segmentation uncertainty to improve the robustness of the reported LVEF. The experiments using a dataset of 2,680 patients show that the proposed approach could increase the LVEF estimation's R2 score with a noticeable relative margin of 17.9%, while automatically detecting and discarding around 6% of cases with the highest predictive uncertainty.
MoreTranslated text
Key words
Uncertainty Estimation, Bayesian Deep Learning, Image Segmentation, Left Ventricular Ejection Fraction, Echocardiography
AI Read Science
Must-Reading Tree
Example
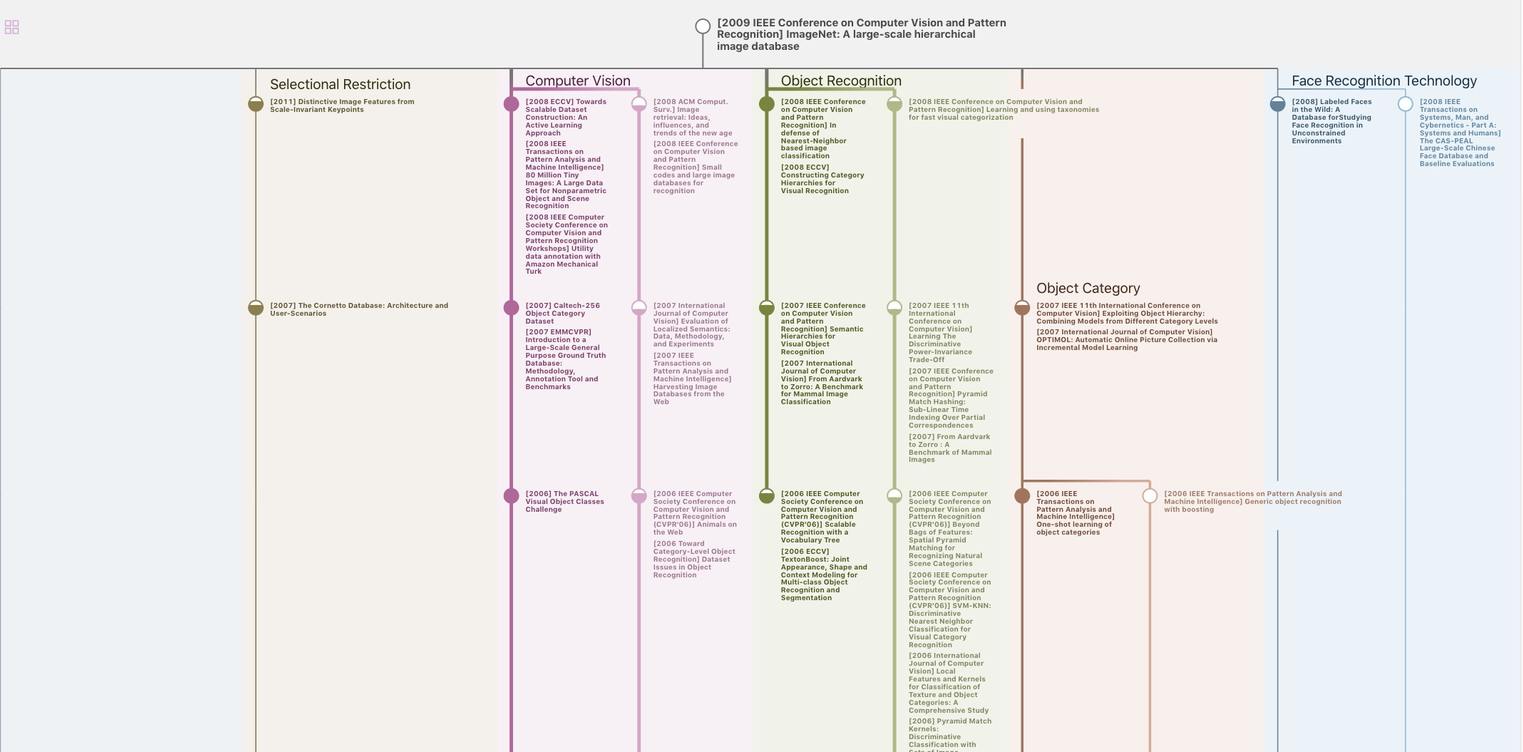
Generate MRT to find the research sequence of this paper
Chat Paper
Summary is being generated by the instructions you defined