$\ell_2$-norm Flow Diffusion in Near-Linear Time
arxiv(2021)
摘要
Diffusion is a fundamental graph process and has been a basic building block in the study of graph clustering and graph learning tasks such as node classification. In this paper, we initiate the study of computationally efficient diffusion primitives beyond random walk. We provide an $\widetilde{O}(m)$-time randomized algorithm for the $\ell_2$-norm flow diffusion problem, obtaining the approximation factor of $1+1/\mathrm{poly}(n)$. Using the connection between its dual solution and local cut structure, we give an alternative approach for finding locally-biased low conductance cuts. It is done simply by sweeping over the dual solution vector. This algorithm demonstrates a novel way of dealing with inequality constraints in graph optimization problems. It adapts the high-level algorithmic framework of Laplacian system solvers, but requires several new tools: vertex elimination under constraints, a new family of graph ultra-sparsifiers, and accelerated proximal gradient methods with inexact proximal mapping computation.
更多查看译文
AI 理解论文
溯源树
样例
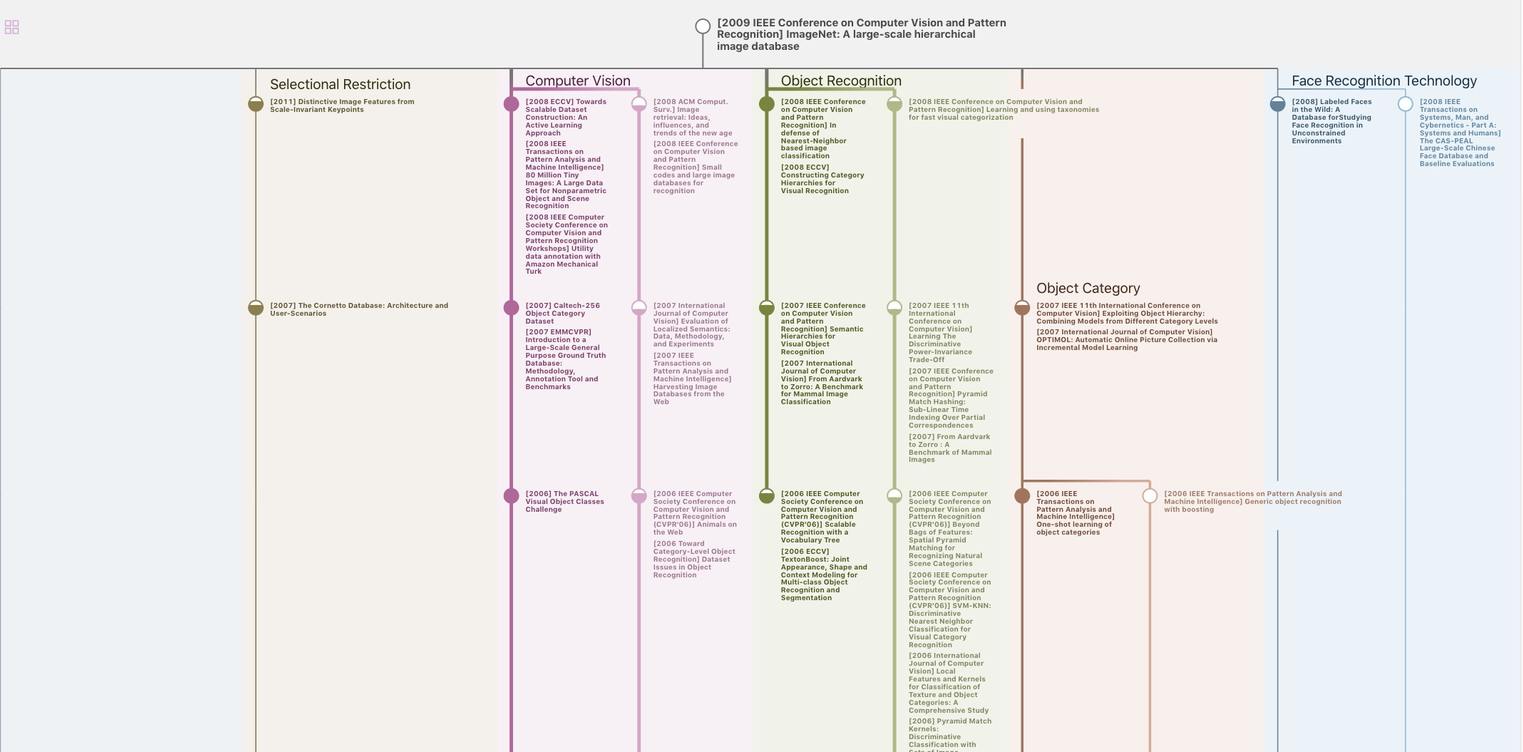
生成溯源树,研究论文发展脉络
Chat Paper
正在生成论文摘要