Stability and Super-Resolution of MUSIC and ESPRIT for Multi-Snapshot Spectral Estimation
IEEE TRANSACTIONS ON SIGNAL PROCESSING(2022)
摘要
This paper studies the spectral estimation problem of estimating the locations of a fixed number of point sources given multiple snapshots of Fourier measurements collected by a uniform array of sensors. We prove novel stability bounds for MUSIC and ESPRIT as a function of the noise standard deviation, number of snapshots, source amplitudes, and support. Our most general result is a perturbation bound of the signal space in terms of the minimum singular value of Fourier matrices. When the point sources are located in several separated clumps, we provide an explicit upper bound of the noise-space correlation perturbation error in MUSIC and the support error in ESPRIT in terms of a Super-Resolution Factor (SRF). The upper bound for ESPRIT is then compared with a new Cramer-Rao lower bound for the clumps model. As a result, we show that ESPRIT is comparable to that of the optimal unbiased estimator(s) in terms of the dependence on noise, number of snapshots and SRF. As a byproduct of our analysis, we discover several fundamental differences between the single-snapshot and multi-snapshot problems. Our theory is validated by numerical experiments.
更多查看译文
关键词
Multiple signal classification, Estimation, Superresolution, Sensor arrays, Upper bound, Perturbation methods, Standards, Multi-snapshot spectral estimation, stability, super-resolution, MUSIC algorithm, optimality of ESPRIT algorithm, Cramer-Rao lower bound, array imaging
AI 理解论文
溯源树
样例
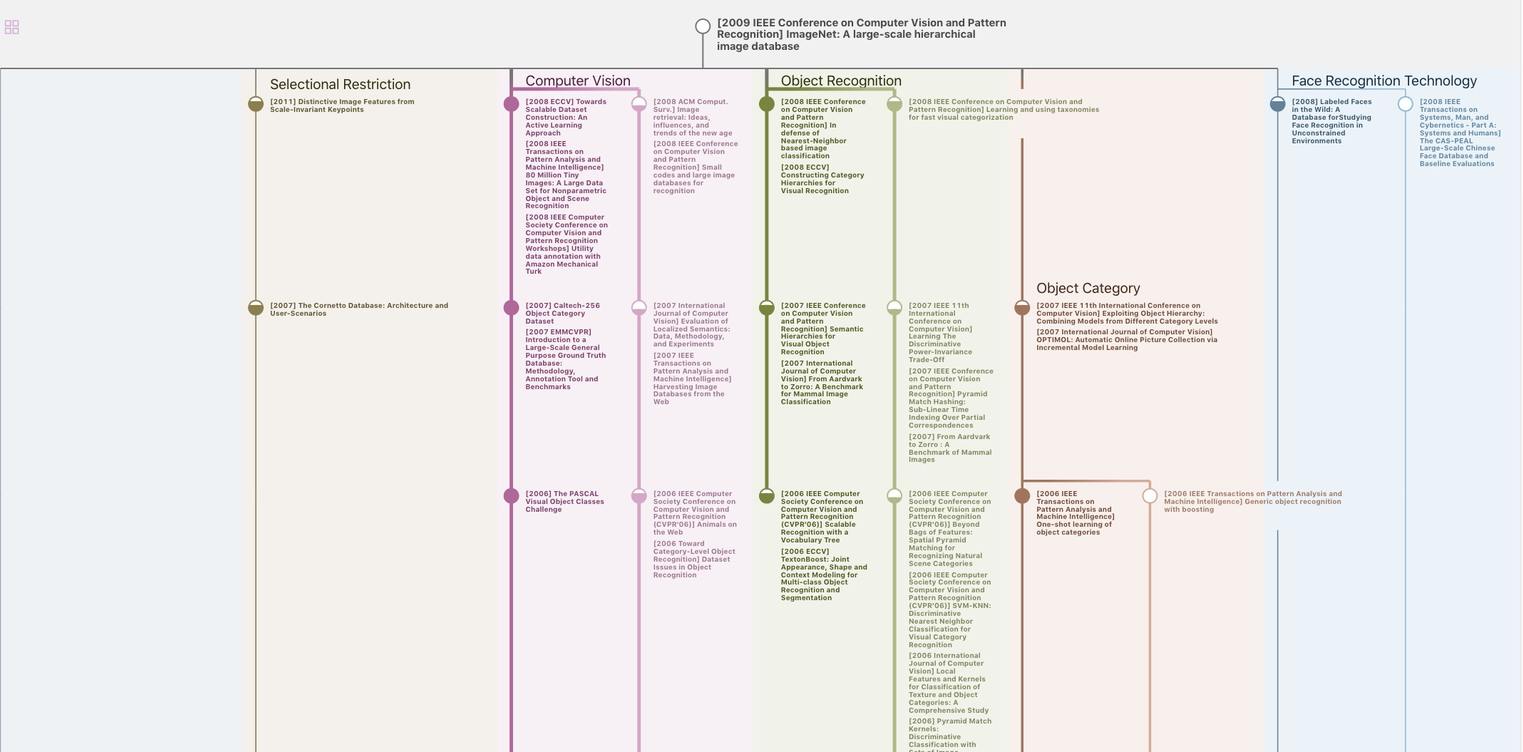
生成溯源树,研究论文发展脉络
Chat Paper
正在生成论文摘要