Deep Learning on Monocular Object Pose Detection and Tracking: A Comprehensive Overview
ACM Computing Surveys(2023)
摘要
AbstractObject pose detection and tracking has recently attracted increasing attention due to its wide applications in many areas, such as autonomous driving, robotics, and augmented reality. Among methods for object pose detection and tracking, deep learning is the most promising one that has shown better performance than others. However, survey study about the latest development of deep learning-based methods is lacking. Therefore, this study presents a comprehensive review of recent progress in object pose detection and tracking that belongs to the deep learning technical route. To achieve a more thorough introduction, the scope of this study is limited to methods taking monocular RGB/RGBD data as input and covering three kinds of major tasks: instance-level monocular object pose detection, category-level monocular object pose detection, and monocular object pose tracking. In our work, metrics, datasets, and methods of both detection and tracking are presented in detail. Comparative results of current state-of-the-art methods on several publicly available datasets are also presented, together with insightful observations and inspiring future research directions.
更多查看译文
关键词
Object pose detection,object pose tracking,instance-level,category-level,monocular
AI 理解论文
溯源树
样例
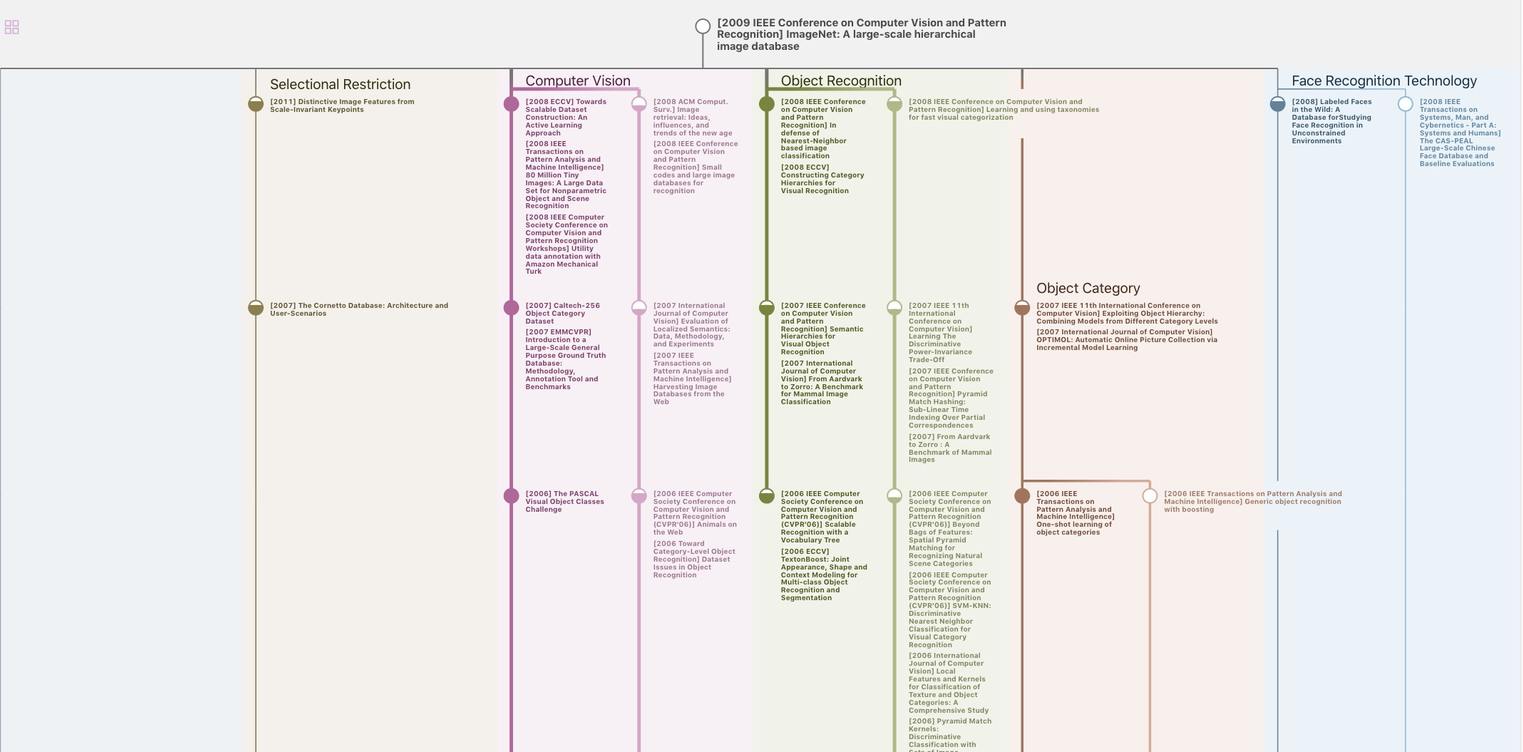
生成溯源树,研究论文发展脉络
Chat Paper
正在生成论文摘要