Radar Odometry Combining Probabilistic Estimation And Unsupervised Feature Learning
ROBOTICS: SCIENCE AND SYSTEM XVII(2021)
摘要
This paper presents a radar odometry method that combines probabilistic trajectory estimation and deep learned features without needing groundtruth pose information. The feature network is trained unsupervised, using only the on-board radar data. With its theoretical foundation based on a data likelihood objective, our method leverages a deep network for processing rich radar data, and a non-differentiable classic estimator for probabilistic inference. We provide extensive experimental results on both the publicly available Oxford Radar RobotCar Dataset and an additional 100 km of driving collected in an urban setting. Our sliding-window implementation of radar odometry outperforms most hand-crafted methods and approaches the current state of the art without requiring a groundtruth trajectory for training. We also demonstrate the effectiveness of radar odometry under adverse weather conditions. Code for this project can be found at: https://github.com/utiasASRL/hero_radar_odometry
更多查看译文
关键词
unsupervised feature learning,probabilistic estimation
AI 理解论文
溯源树
样例
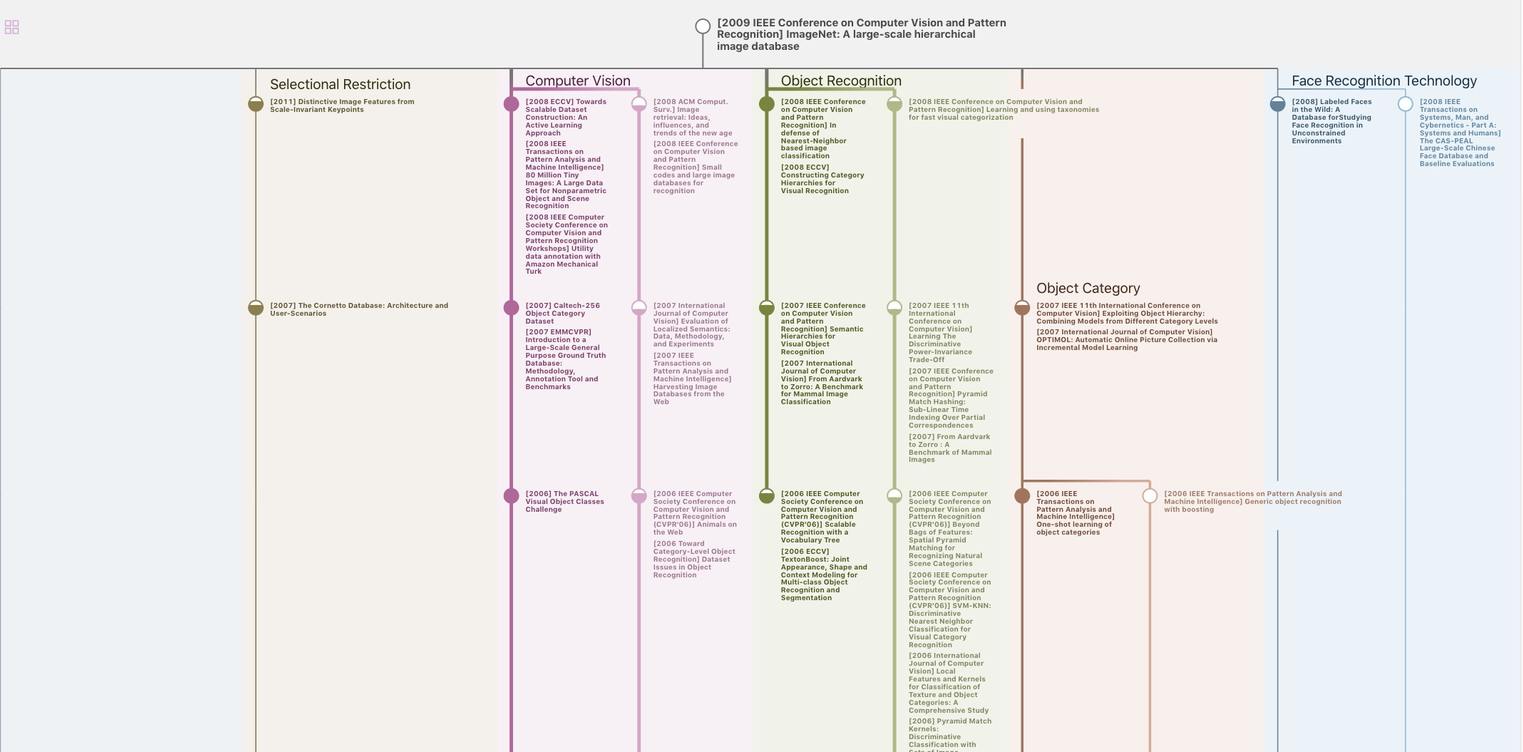
生成溯源树,研究论文发展脉络
Chat Paper
正在生成论文摘要