Reinforcement learning reveals fundamental limits on the mixing of active particles
SOFT MATTER(2022)
摘要
The control of far-from-equilibrium physical systems, including active materials, requires advanced control strategies due to the non-linear dynamics and long-range interactions between particles, preventing explicit solutions to optimal control problems. In such situations, Reinforcement Learning (RL) has emerged as an approach to derive suitable control strategies. However, for active matter systems, it is an important open question how the mathematical structure and the physical properties determine the tractability of RL. In this paper, we demonstrate that RL can only find good mixing strategies for active matter systems that combine attractive and repulsive interactions. Using analytic results from dynamical systems theory, we show that combining both interaction types is indeed necessary for the existence of mixing-inducing hyperbolic dynamics and therefore the ability of RL to find homogeneous mixing strategies. In particular, we show that for drag-dominated translational-invariant particle systems, mixing relies on combined attractive and repulsive interactions. Therefore, our work demonstrates which experimental developments need to be made to make protein-based active matter applicable, and it provides some classification of microscopic interactions based on macroscopic behavior.
更多查看译文
AI 理解论文
溯源树
样例
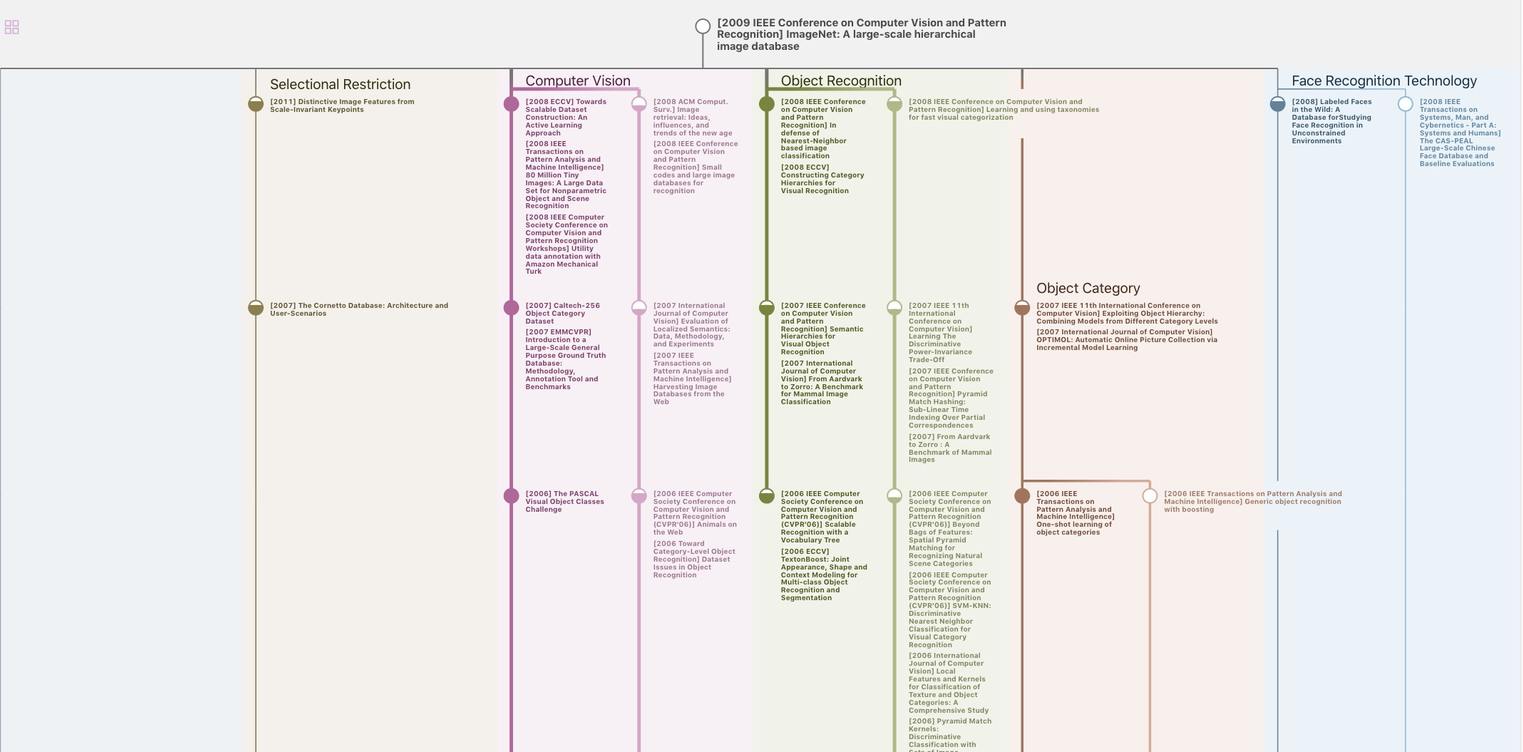
生成溯源树,研究论文发展脉络
Chat Paper
正在生成论文摘要