EDLm 6 APred: ensemble deep learning approach for mRNA m 6 A site prediction
BMC BIOINFORMATICS(2021)
摘要
Background As a common and abundant RNA methylation modification, N6-methyladenosine (m 6 A) is widely spread in various species' transcriptomes, and it is closely related to the occurrence and development of various life processes and diseases. Thus, accurate identification of m 6 A methylation sites has become a hot topic. Most biological methods rely on high-throughput sequencing technology, which places great demands on the sequencing library preparation and data analysis. Thus, various machine learning methods have been proposed to extract various types of features based on sequences, then occupied conventional classifiers, such as SVM, RF, etc., for m 6 A methylation site identification. However, the identification performance relies heavily on the extracted features, which still need to be improved. Results This paper mainly studies feature extraction and classification of m 6 A methylation sites in a natural language processing way, which manages to organically integrate the feature extraction and classification simultaneously, with consideration of upstream and downstream information of m 6 A sites. One-hot, RNA word embedding, and Word2vec are adopted to depict sites from the perspectives of the base as well as its upstream and downstream sequence. The BiLSTM model, a well-known sequence model, was then constructed to discriminate the sequences with potential m 6 A sites. Since the above-mentioned three feature extraction methods focus on different perspectives of m 6 A sites, an ensemble deep learning predictor (EDLm 6 APred) was finally constructed for m 6 A site prediction. Experimental results on human and mouse data sets show that EDLm 6 APred outperforms the other single ones, indicating that base, upstream, and downstream information are all essential for m 6 A site detection. Compared with the existing m 6 A methylation site prediction models without genomic features, EDLm 6 APred obtains 86.6% of the area under receiver operating curve on the human data sets, indicating the effectiveness of sequential modeling on RNA. To maximize user convenience, a webserver was developed as an implementation of EDLm 6 APred and made publicly available at www.xjtlu.edu.cn/biologicalsciences/EDLm6APred . Conclusions Our proposed EDLm 6 APred method is a reliable predictor for m 6 A methylation sites.
更多查看译文
关键词
m(6)A methylation modification, Word embedding, Deep learning, Predictor
AI 理解论文
溯源树
样例
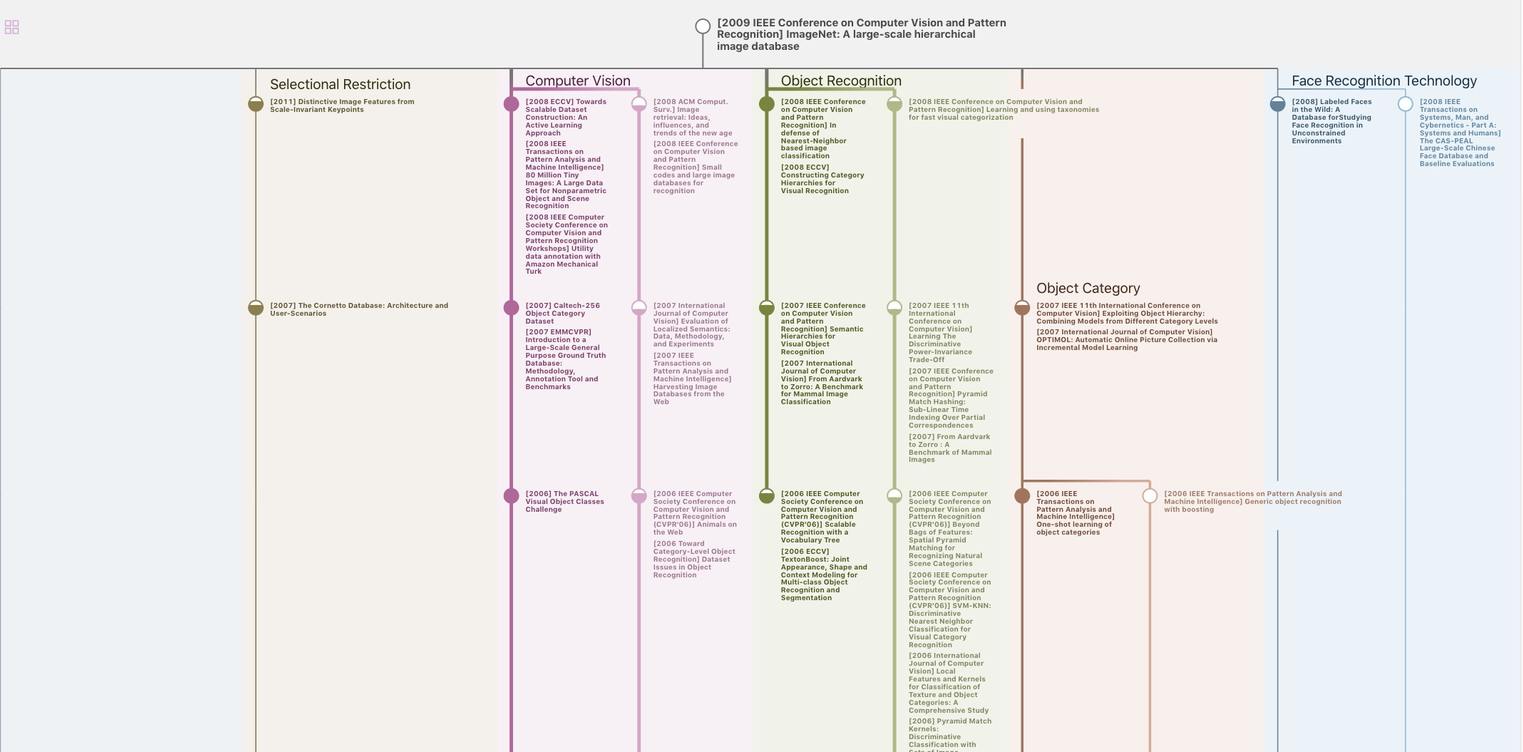
生成溯源树,研究论文发展脉络
Chat Paper
正在生成论文摘要