Efficient unsupervised drift detector for fast and high-dimensional data streams
KNOWLEDGE AND INFORMATION SYSTEMS(2021)
摘要
Stream mining considers the online arrival of examples at high speed and the possibility of changes in its descriptive features or class definitions compared with past knowledge (i.e., concept drifts). The fast detection of drifts is essential to keep the predictive model updated and stable in changing environments. For many applications, such as those related to smart sensors, the high number of features is an additional challenge in terms of memory and time for stream processing. This paper presents an unsupervised and model-independent concept drift detector suitable for high-speed and high-dimensional data streams. We propose a straightforward two-dimensional data representation that allows the faster processing of datasets with a large number of examples and dimensions. We developed an adaptive drift detector on this visual representation that is efficient for fast streams with thousands of features and is accurate as existing costly methods that perform various statistical tests considering each feature individually. Our method achieves better performance measured by execution time and accuracy in classification problems for different types of drifts. The experimental evaluation considering synthetic and real data demonstrates the method’s versatility in several domains, including entomology, medicine, and transportation systems.
更多查看译文
关键词
Data stream,Concept drift,Unsupervised drift detector
AI 理解论文
溯源树
样例
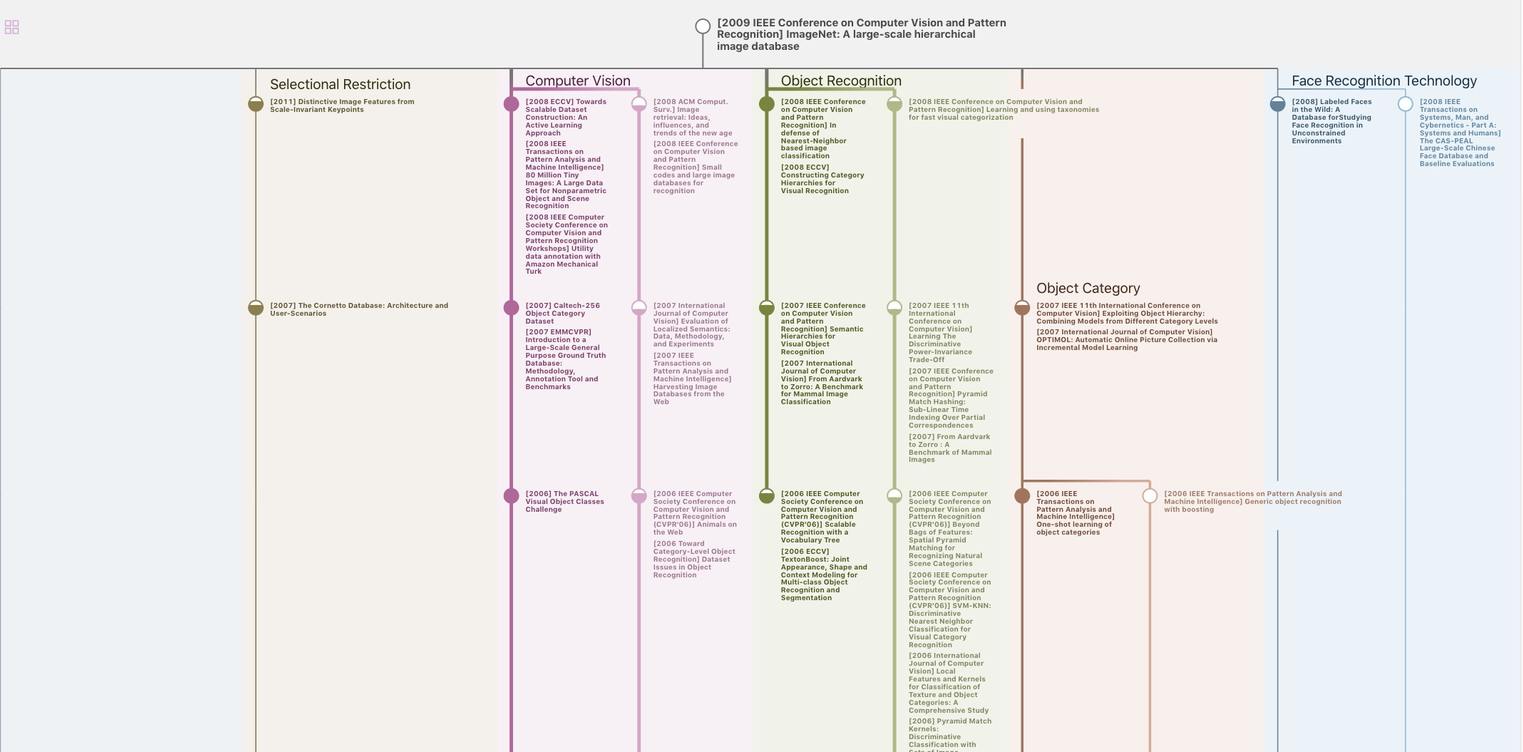
生成溯源树,研究论文发展脉络
Chat Paper
正在生成论文摘要