Polygonal Unadjusted Langevin Algorithms: Creating stable and efficient adaptive algorithms for neural networks
ICML 2024(2024)
Abstract
We present a new class of Langevin-based algorithms, which overcomes many of the known shortcomings of popular adaptive optimizers that are currently used for the fine tuning of deep learning models. Its underpinning theory relies on recent advances of Euler-Krylov polygonal approximations for stochastic differential equations (SDEs) with monotone coefficients. As a result, it inherits the stability properties of tamed algorithms, while it addresses other known issues, e.g. vanishing gradients in deep learning. In particular, we provide a nonasymptotic analysis and full theoretical guarantees for the convergence properties of an algorithm of this novel class, which we named TH$\varepsilon$O POULA (or, simply, TheoPouLa). Finally, several experiments are presented with different types of deep learning models, which show the superior performance of TheoPouLa over many popular adaptive optimization algorithms.
MoreTranslated text
Key words
Stochastic optimization,nonconvex optimization,non-asymptotic estimates,taming technique,Euler-Krylov polygonal approximation
AI Read Science
Must-Reading Tree
Example
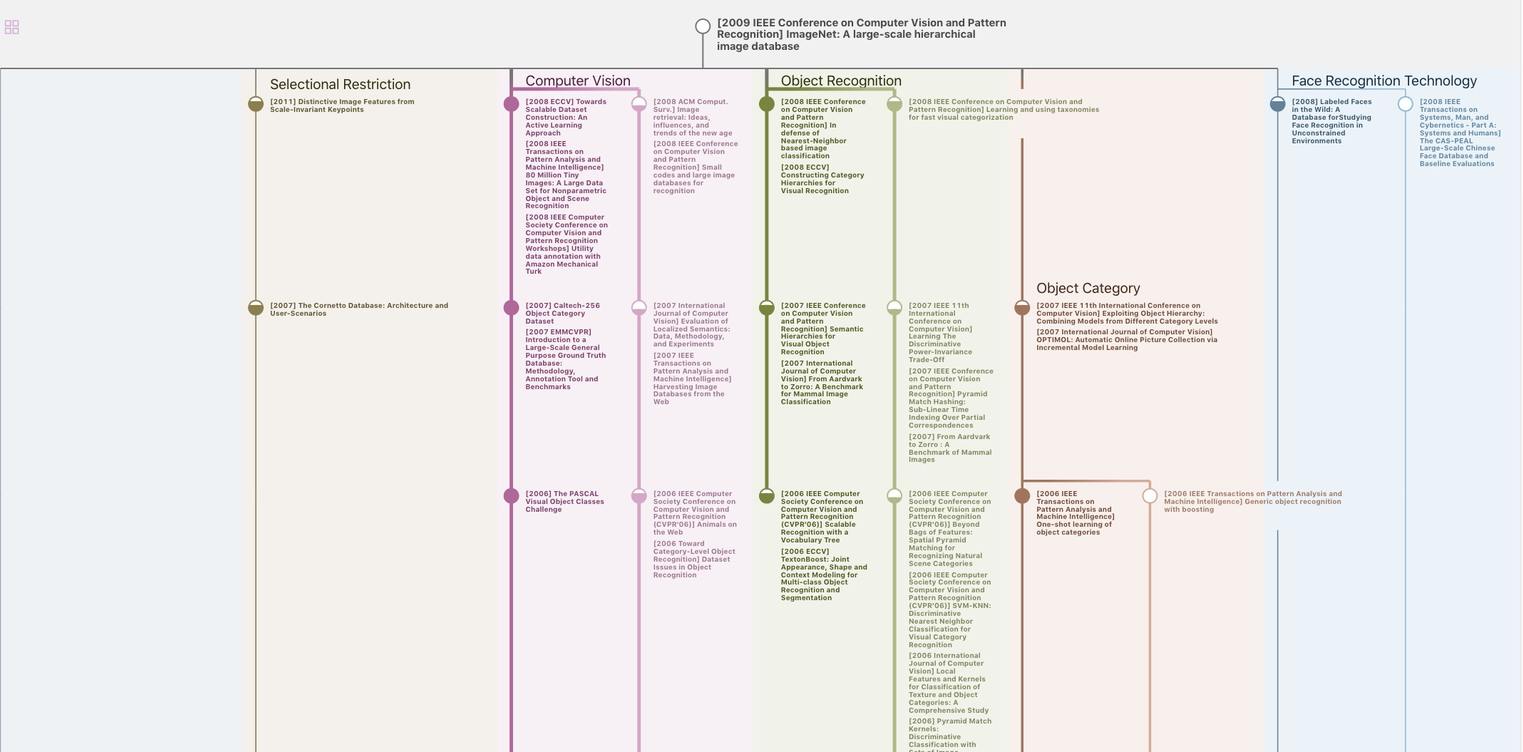
Generate MRT to find the research sequence of this paper
Chat Paper
Summary is being generated by the instructions you defined