Knowledge Inheritance for Pre-trained Language Models
NAACL 2022: THE 2022 CONFERENCE OF THE NORTH AMERICAN CHAPTER OF THE ASSOCIATION FOR COMPUTATIONAL LINGUISTICS: HUMAN LANGUAGE TECHNOLOGIES(2021)
摘要
Recent explorations of large-scale pre-trained language models (PLMs) such as GPT-3 have revealed the power of PLMs with huge amounts of parameters, setting off a wave of training ever-larger PLMs. However, training a large-scale PLM requires tremendous amounts of computational resources, which is time-consuming and expensive. In addition, existing large-scale PLMs are mainly trained from scratch individually, ignoring the availability of many existing well-trained PLMs. To this end, we explore the question that how can previously trained PLMs benefit training larger PLMs in future. Specifically, we introduce a novel pre-training framework named "knowledge inheritance" (KI), which combines both self-learning and teacher-guided learning to efficiently train larger PLMs. Sufficient experimental results demonstrate the feasibility of our KI framework. We also conduct empirical analyses to explore the effects of teacher PLMs' pre-training settings, including model architecture, pre-training data, etc. Finally, we show that KI can well support lifelong learning and knowledge transfer.
更多查看译文
关键词
knowledge,language,models,inheritance,pre-trained
AI 理解论文
溯源树
样例
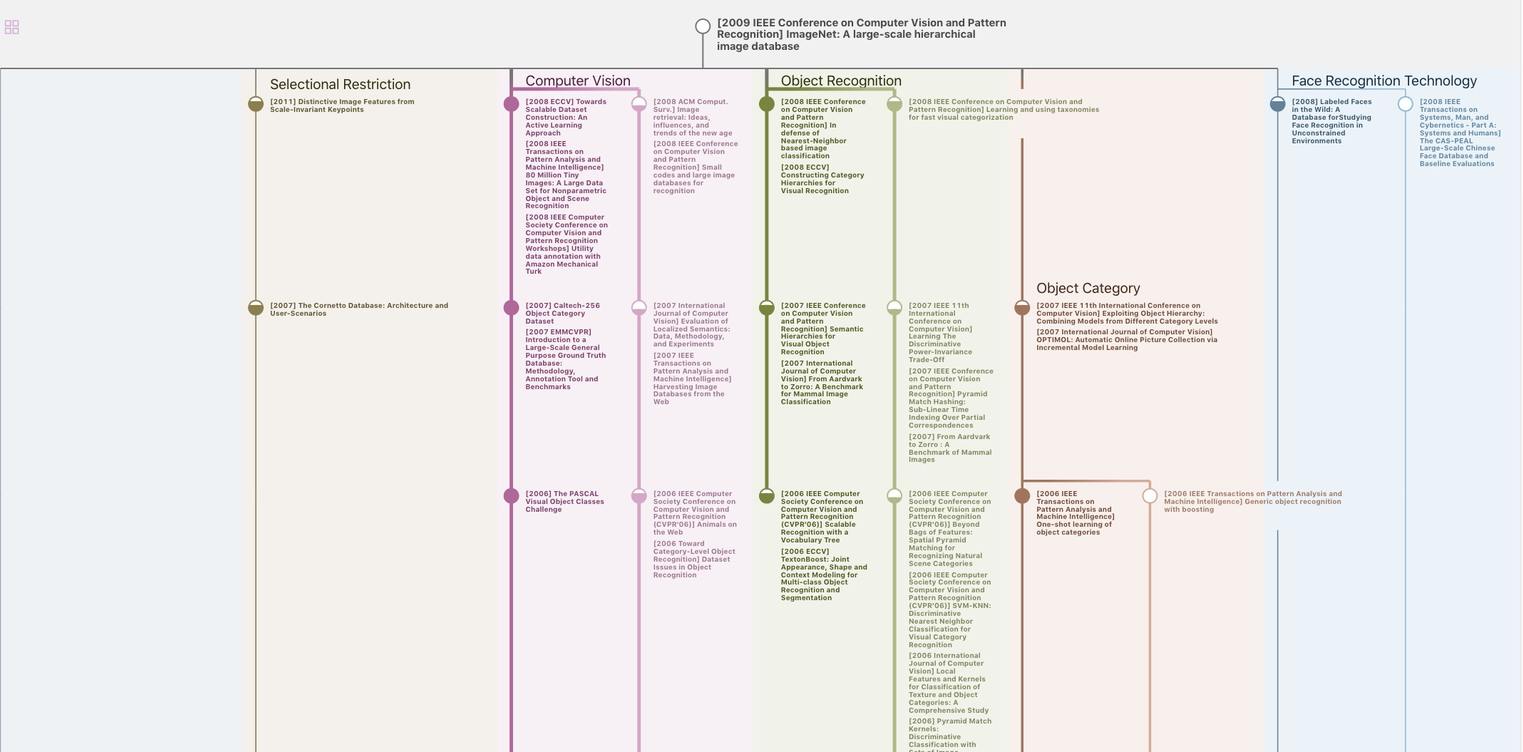
生成溯源树,研究论文发展脉络
Chat Paper
正在生成论文摘要