Implicit Regularization in Matrix Sensing via Mirror Descent.
Annual Conference on Neural Information Processing Systems(2021)
摘要
We study discrete-time mirror descent applied to the unregularized empirical risk in matrix sensing. In both the general case of rectangular matrices and the particular case of positive semidefinite matrices, a simple potential-based analysis in terms of the Bregman divergence allows us to establish convergence of mirror descent---with different choices of the mirror maps---to a matrix that, among all global minimizers of the empirical risk, minimizes a quantity explicitly related to the nuclear norm, the Frobenius norm, and the von Neumann entropy. In both cases, this characterization implies that mirror descent, a first-order algorithm minimizing the unregularized empirical risk, recovers low-rank matrices under the same set of assumptions that are sufficient to guarantee recovery for nuclear-norm minimization. When the sensing matrices are symmetric and commute, we show that gradient descent with full-rank factorized parametrization is a first-order approximation to mirror descent, in which case we obtain an explicit characterization of the implicit bias of gradient flow as a by-product.
更多查看译文
关键词
matrix sensing,implicit regularization
AI 理解论文
溯源树
样例
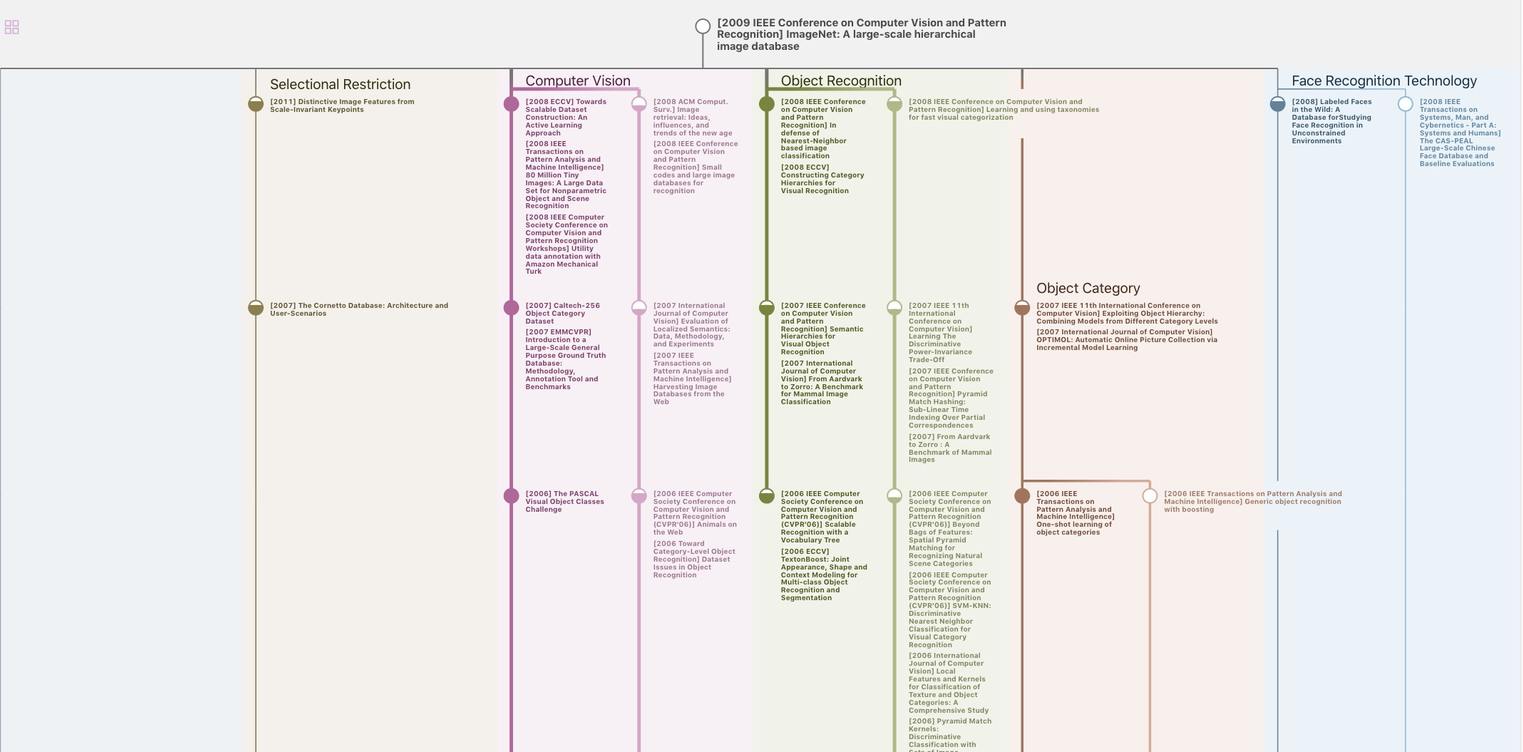
生成溯源树,研究论文发展脉络
Chat Paper
正在生成论文摘要