Exploring the Diagnostic Potential of Adding T2 Dependence in Diffusion-Weighted MR Imaging of the Prostate
wos(2021)
AI Read Science
Must-Reading Tree
Example
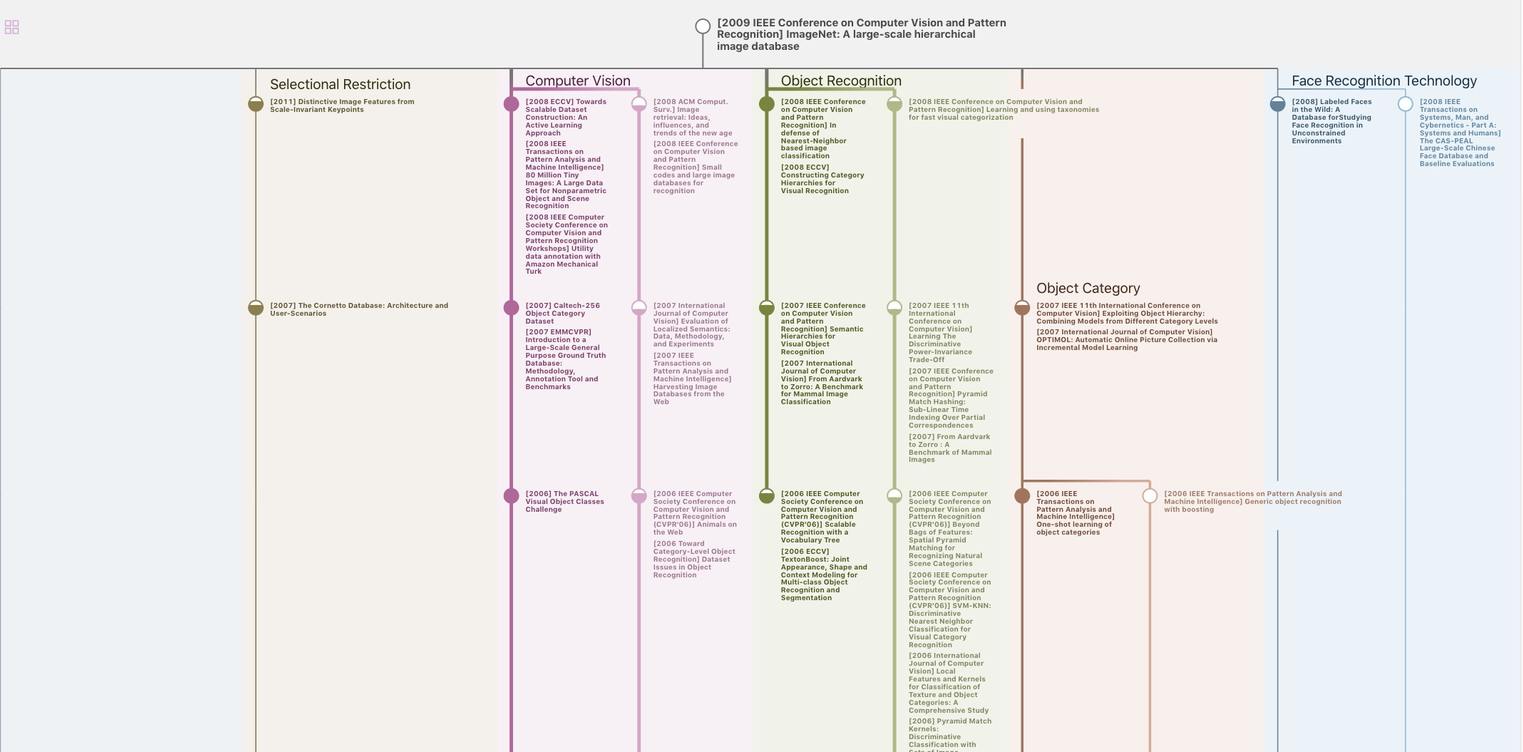
Generate MRT to find the research sequence of this paper
Chat Paper
Summary is being generated by the instructions you defined