Local whittle estimation of high-dimensional long-run variance and precision matrices
ANNALS OF STATISTICS(2023)
摘要
This work develops nonasymptotic theory for estimation of the longrun variance matrix and its inverse, the so-called precision matrix, for highdimensional time series under general assumptions on the dependence structure including long-range dependence. The estimation involves shrinkage techniques, which are thresholding and penalizing versions of the classical multivariate local Whittle estimator. The results ensure consistent estimation in a double asymptotic regime where the number of component time series is allowed to grow with the sample size as long as the true model parameters are sparse. The key technical result is a concentration inequality of the local Whittle estimator for the long-run variance matrix around the true model parameters. In particular, it handles simultaneously the estimation of the memory parameters, which enter the underlying model. Novel algorithms for the considered procedures are proposed, and a simulation study and a data application are also provided.
更多查看译文
关键词
High-dimensional time series,frequency domain,short-and long-range dependence,spectral density estimation,shrinkage estimation,local Whittle estimation
AI 理解论文
溯源树
样例
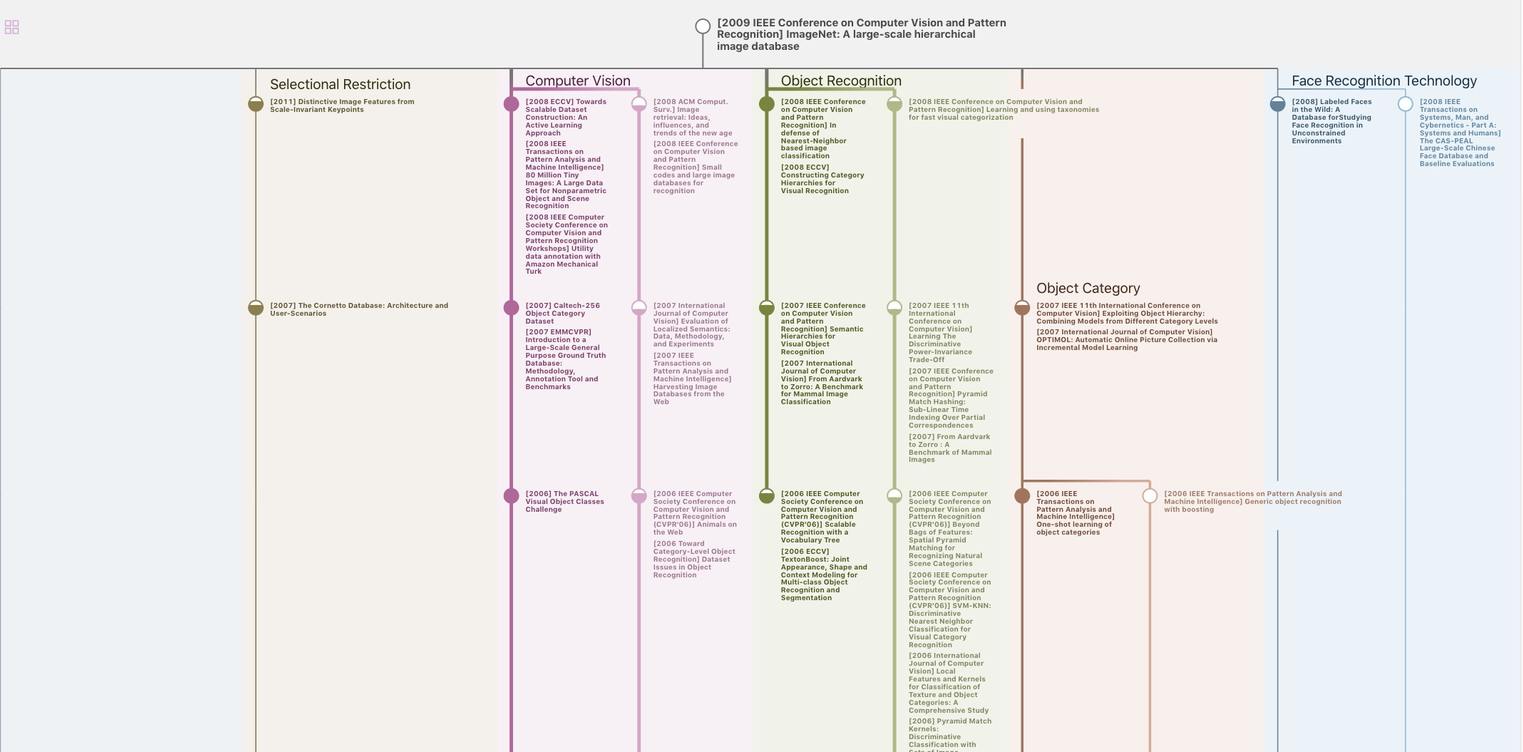
生成溯源树,研究论文发展脉络
Chat Paper
正在生成论文摘要