GoSafe: Globally Optimal Safe Robot Learning
2021 IEEE INTERNATIONAL CONFERENCE ON ROBOTICS AND AUTOMATION (ICRA 2021)(2021)
摘要
When learning policies for robotic systems from data, safety is a major concern, as violation of safety constraints may cause hardware damage. SAFEOPT is an efficient Bayesian optimization (BO) algorithm that can learn policies while guaranteeing safety with high probability. However, its search space is limited to an initially given safe region. We extend this method by exploring outside the initial safe area while still guaranteeing safety with high probability. This is achieved by learning a set of initial conditions from which we can recover safely using a learned backup controller in case of a potential failure. We derive conditions for guaranteed convergence to the global optimum and validate GOSAFE in hardware experiments.
更多查看译文
关键词
GoSafe,globally optimal safe robot learning,learning policies,robotic systems,safety constraints,hardware damage,Bayesian optimization algorithm,search space,learned backup controller
AI 理解论文
溯源树
样例
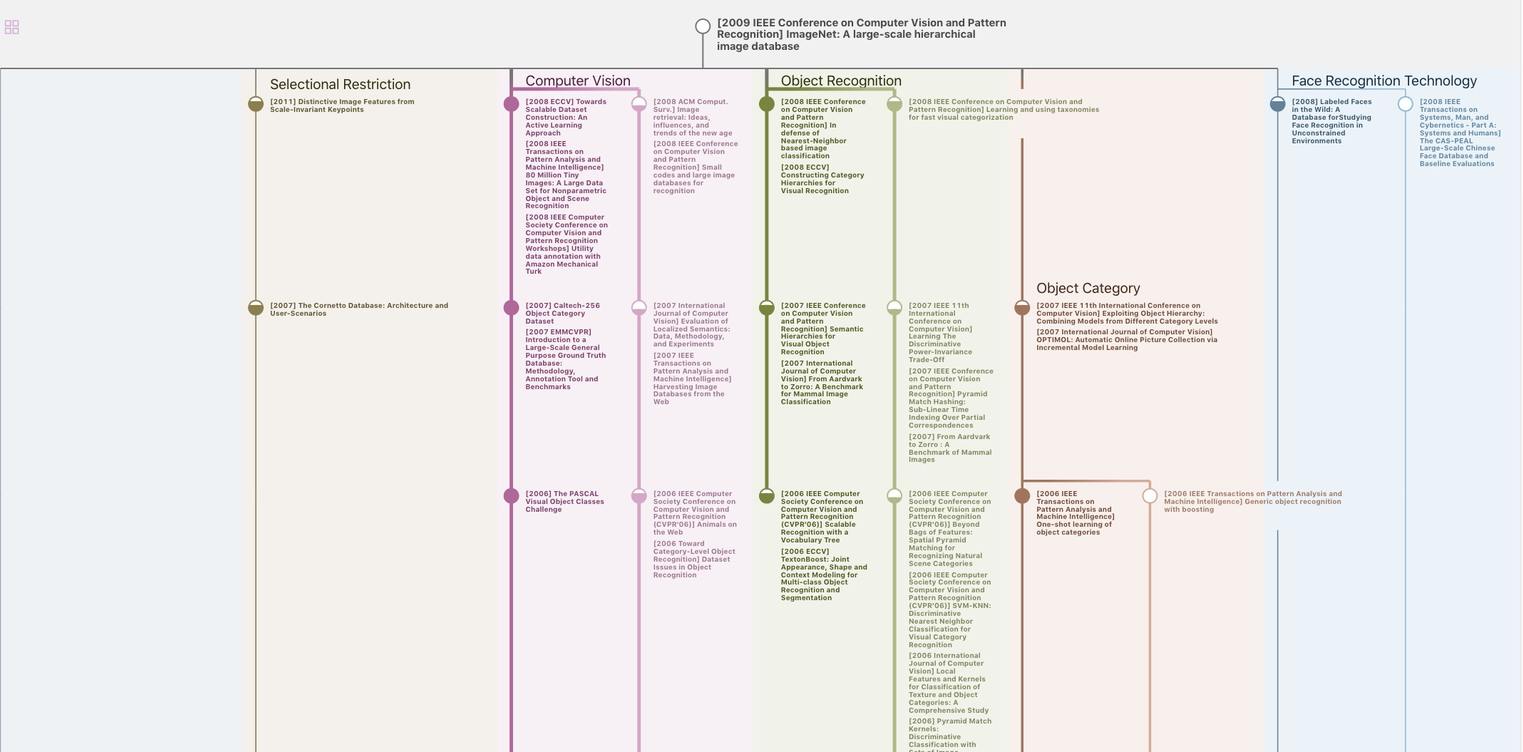
生成溯源树,研究论文发展脉络
Chat Paper
正在生成论文摘要