MeshCNN Fundamentals: Geometric Learning through a Reconstructable Representation
arXiv (Cornell University)(2021)
摘要
Mesh-based learning is one of the popular approaches nowadays to learn shapes. The most established backbone in this field is MeshCNN. In this paper, we propose infusing MeshCNN with geometric reasoning to achieve higher quality learning. Through careful analysis of the way geometry is represented through-out the network, we submit that this representation should be rigid motion invariant, and should allow reconstructing the original geometry. Accordingly, we introduce the first and second fundamental forms as an edge-centric, rotation and translation invariant, reconstructable representation. In addition, we update the originally proposed pooling scheme to be more geometrically driven. We validate our analysis through experimentation, and present consistent improvement upon the MeshCNN baseline, as well as other more elaborate state-of-the-art architectures. Furthermore, we demonstrate this fundamental forms-based representation opens the door to accessible generative machine learning over meshes.
更多查看译文
关键词
geometric learning,representation,fundamentals
AI 理解论文
溯源树
样例
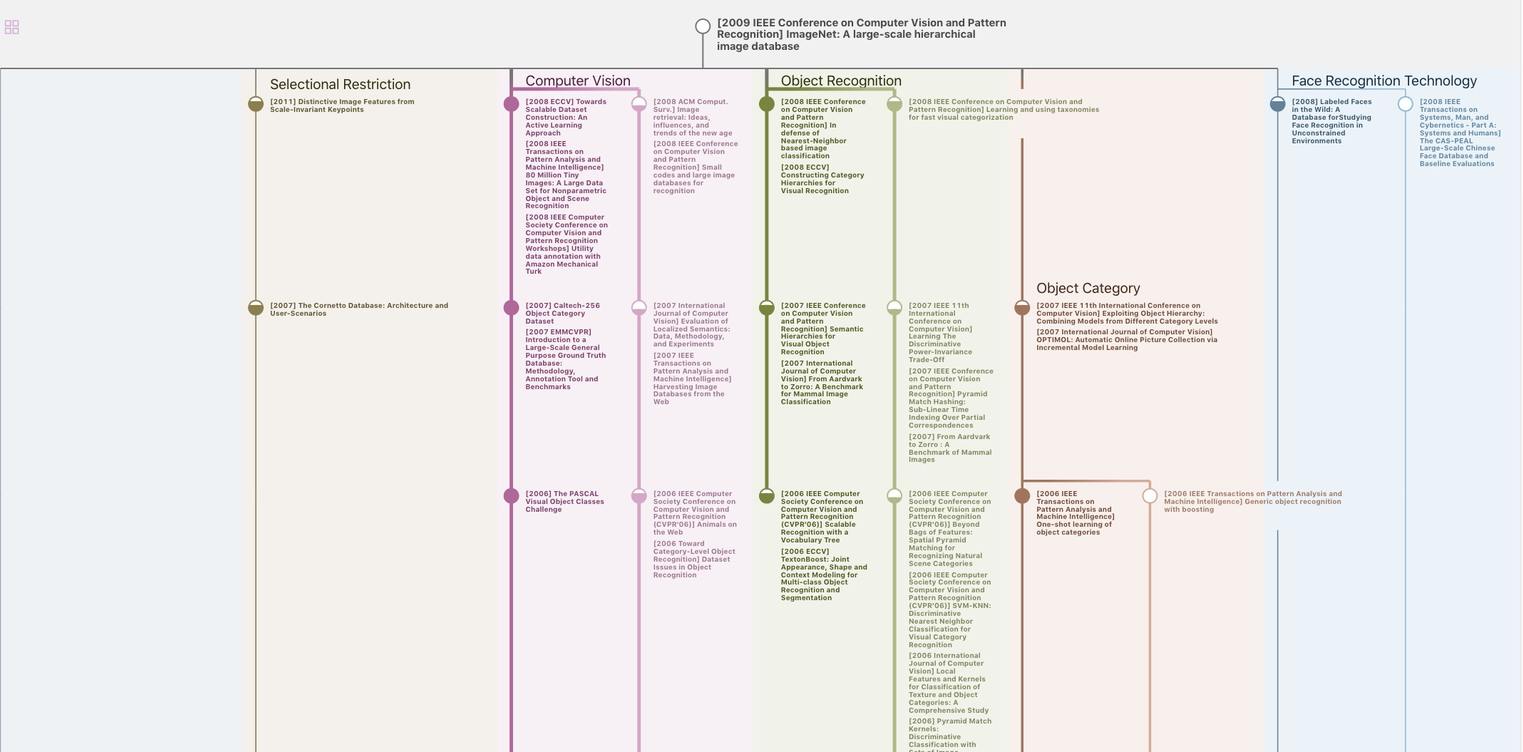
生成溯源树,研究论文发展脉络
Chat Paper
正在生成论文摘要