OpReg-Boost: Learning to Accelerate Online Algorithms with Operator Regression.
Conference on Learning for Dynamics & Control (L4DC)(2022)
摘要
This paper presents a new regularization approach -- termed OpReg-Boost -- to boost the convergence and lessen the asymptotic error of online optimization and learning algorithms. In particular, the paper considers online algorithms for optimization problems with a time-varying (weakly) convex composite cost. For a given online algorithm, OpReg-Boost learns the closest algorithmic map that yields linear convergence; to this end, the learning procedure hinges on the concept of operator regression. We show how to formalize the operator regression problem and propose a computationally-efficient Peaceman-Rachford solver that exploits a closed-form solution of simple quadratically-constrained quadratic programs (QCQPs). Simulation results showcase the superior properties of OpReg-Boost w.r.t. the more classical forward-backward algorithm, FISTA, and Anderson acceleration, and with respect to its close relative convex-regression-boost (CvxReg-Boost) which is also novel but less performing.
更多查看译文
关键词
online algorithms,operator,regression,opreg-boost
AI 理解论文
溯源树
样例
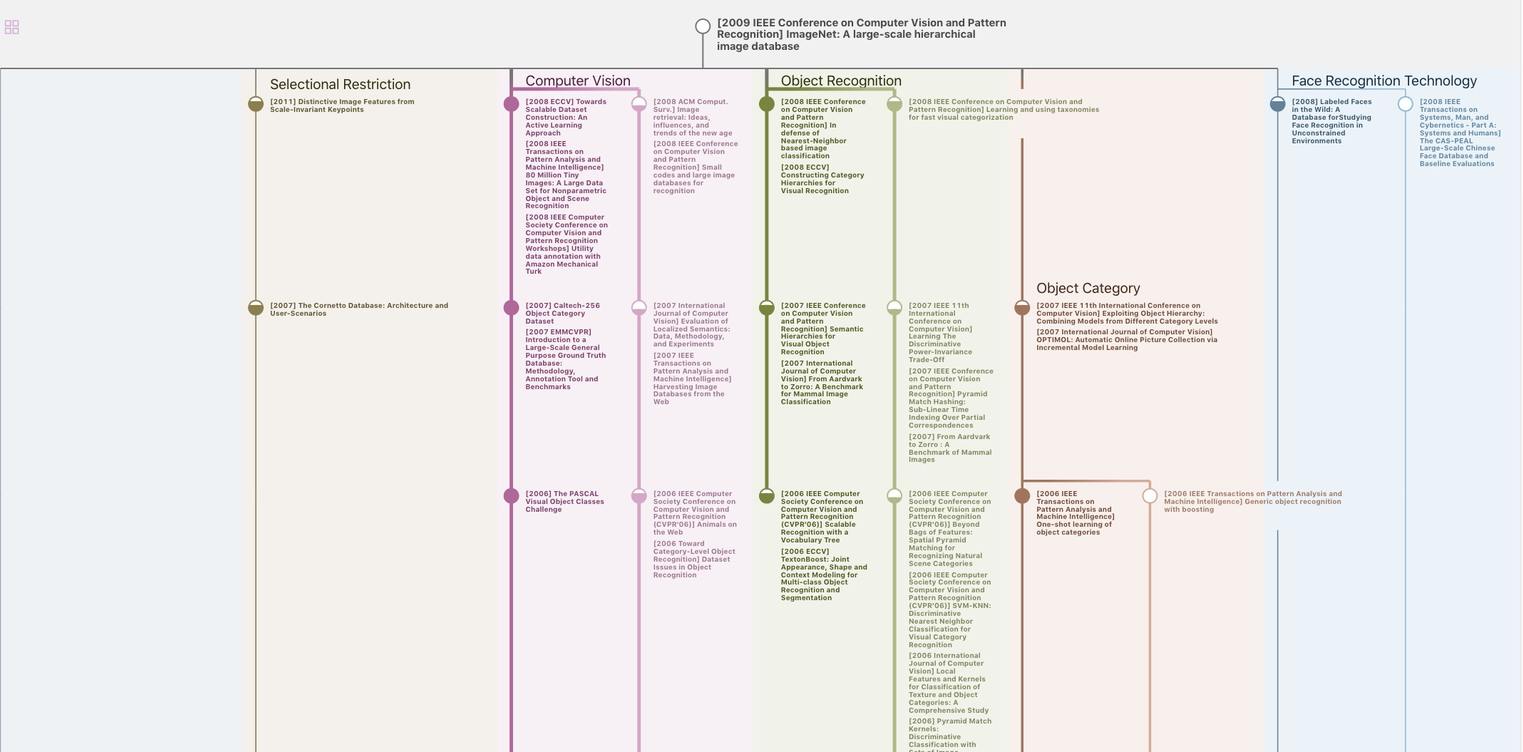
生成溯源树,研究论文发展脉络
Chat Paper
正在生成论文摘要