Ideal Bayesian Spatial Adaptation
JOURNAL OF THE AMERICAN STATISTICAL ASSOCIATION(2023)
摘要
Many real-life applications involve estimation of curves that exhibit complicated shapes including jumps or varying-frequency oscillations. Practical methods have been devised that can adapt to a locally varying complexity of an unknown function (e.g., variable-knot splines, sparse wavelet reconstructions, kernel methods or trees/forests). However, the overwhelming majority of existing asymptotic minimaxity theory is predicated on homogeneous smoothness assumptions. Focusing on locally H & ouml;lder functions, we provide new locally adaptive posterior concentration rate results under the supremum loss for widely used Bayesian machine learning techniques in white noise and nonparametric regression. In particular, we show that popular spike-and-slab priors and Bayesian CART are uniformly locally adaptive. In addition, we propose a new class of repulsive partitioning priors which relate to variable knot splines and which are exact-rate adaptive. For uncertainty quantification, we construct locally adaptive confidence bands whose width depends on the local smoothness and which achieve uniform asymptotic coverage under local self-similarity. To illustrate that spatial adaptation is not at all automatic, we provide lower-bound results showing that popular hierarchical Gaussian process priors fall short of spatial adaptation. Supplementary materials for this article are available online.
更多查看译文
关键词
Bayesian CART,Partitioning priors,Spatial adaptation,Spike-and-slab,Supremum norm
AI 理解论文
溯源树
样例
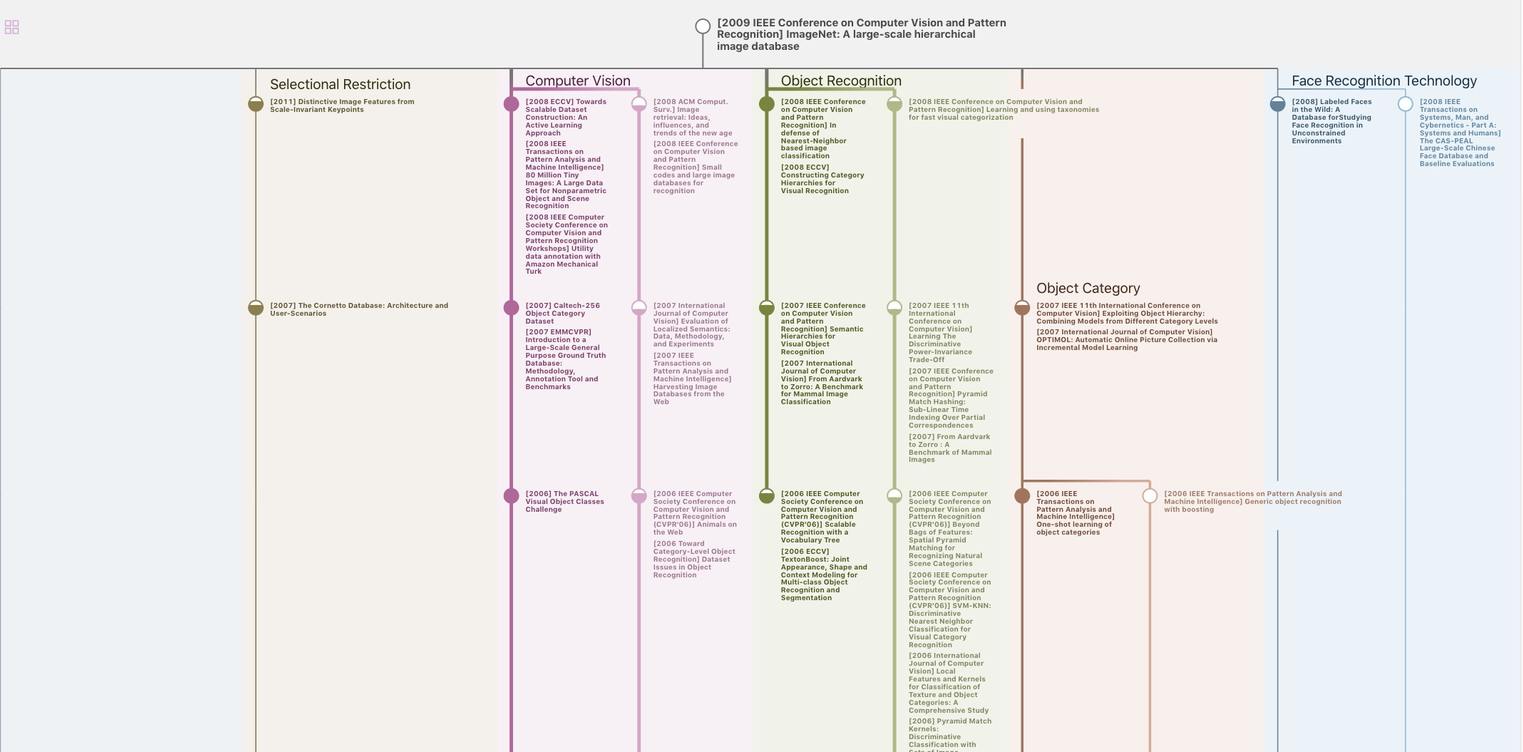
生成溯源树,研究论文发展脉络
Chat Paper
正在生成论文摘要