Bootstrapping a Music Voice Assistant with Weak Supervision.
NAACL-HLT (Industry Papers)(2021)
摘要
One of the first building blocks to create a voice assistant relates to the task of tagging entities or attributes in user queries. This can be particularly challenging when entities are in the tenth of millions, as is the case of e.g. music catalogs. Training slot tagging models at an industrial scale requires large quantities of accurately labeled user queries, which are often hard and costly to gather. On the other hand, voice assistants typically collect plenty of unlabeled queries that often remain unexploited. This paper presents a weakly-supervised methodology to label large amounts of voice query logs, enhanced with a manual filtering step. Our experimental evaluations show that slot tagging models trained on weakly-supervised data outperform models trained on hand-annotated or synthetic data, at a lower cost. Further, manual filtering of weakly-supervised data leads to a very significant reduction in Sentence Error Rate, while allowing us to drastically reduce human curation efforts from weeks to hours, with respect to hand-annotation of queries. The method is applied to successfully bootstrap a slot tagging system for a major music streaming service that currently serves several tens of thousands of daily voice queries.
更多查看译文
关键词
music voice assistant
AI 理解论文
溯源树
样例
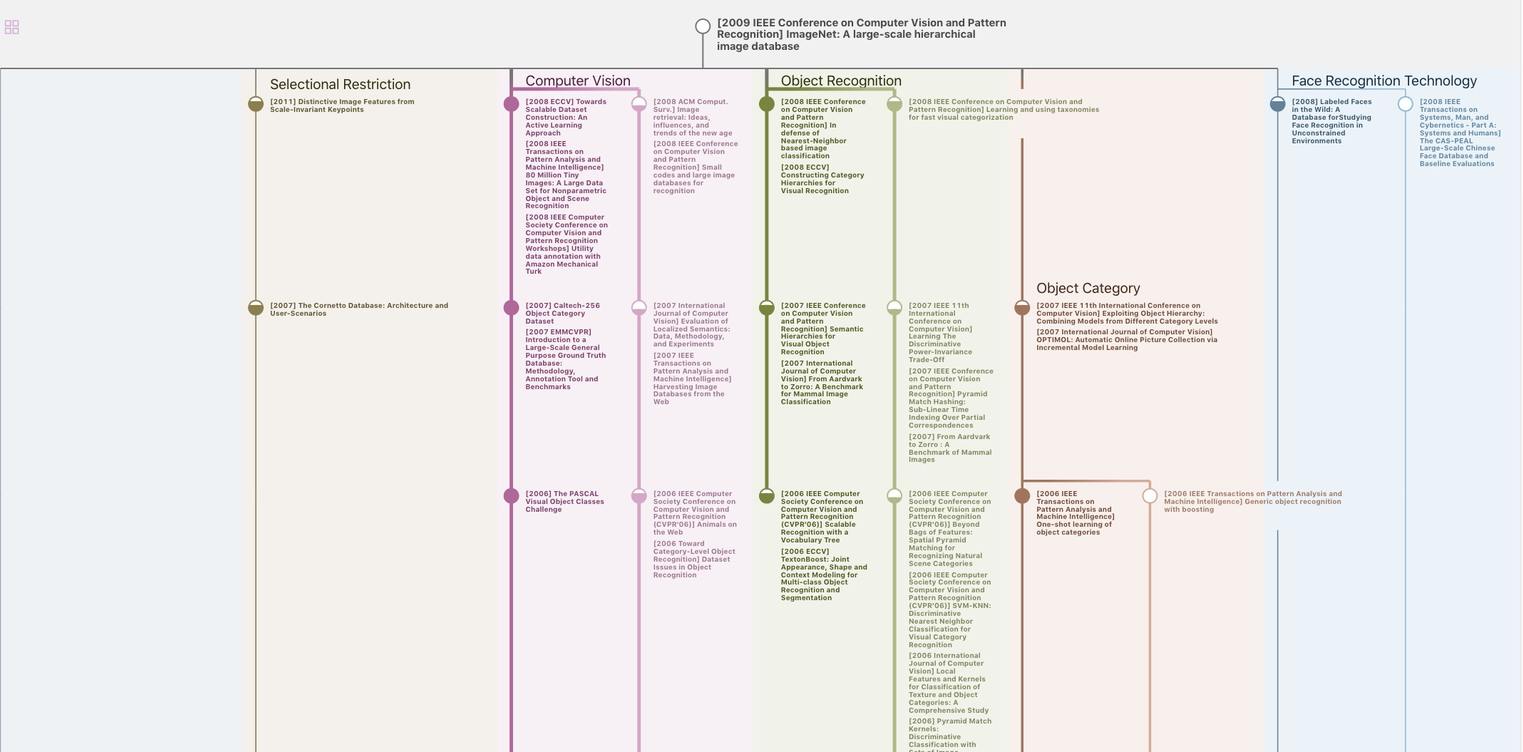
生成溯源树,研究论文发展脉络
Chat Paper
正在生成论文摘要