EntityBERT: Entity-centric Masking Strategy for Model Pretraining for the Clinical Domain
BioNLP@NAACL-HLT(2021)
Abstract
Transformer-based neural language models have led to breakthroughs for a variety of natural language processing (NLP) tasks. However, most models are pretrained on general domain data. We propose a methodology to produce a model focused on the clinical domain: continued pretraining of a model with a broad representation of biomedical terminology (PubMedBERT) on a clinical corpus along with a novel entity-centric masking strategy to infuse domain knowledge in the learning process. We show that such a model achieves superior results on clinical extraction tasks by comparing our entity-centric masking strategy with classic random masking on three clinical NLP tasks: cross-domain negation detection, document time relation (DocTimeRel) classification, and temporal relation extraction. We also evaluate our models on the PubMedQA dataset to measure the models’ performance on a non-entity-centric task in the biomedical domain. The language addressed in this work is English.
MoreTranslated text
Key words
model pretraining,clinical domain,entity-centric
AI Read Science
Must-Reading Tree
Example
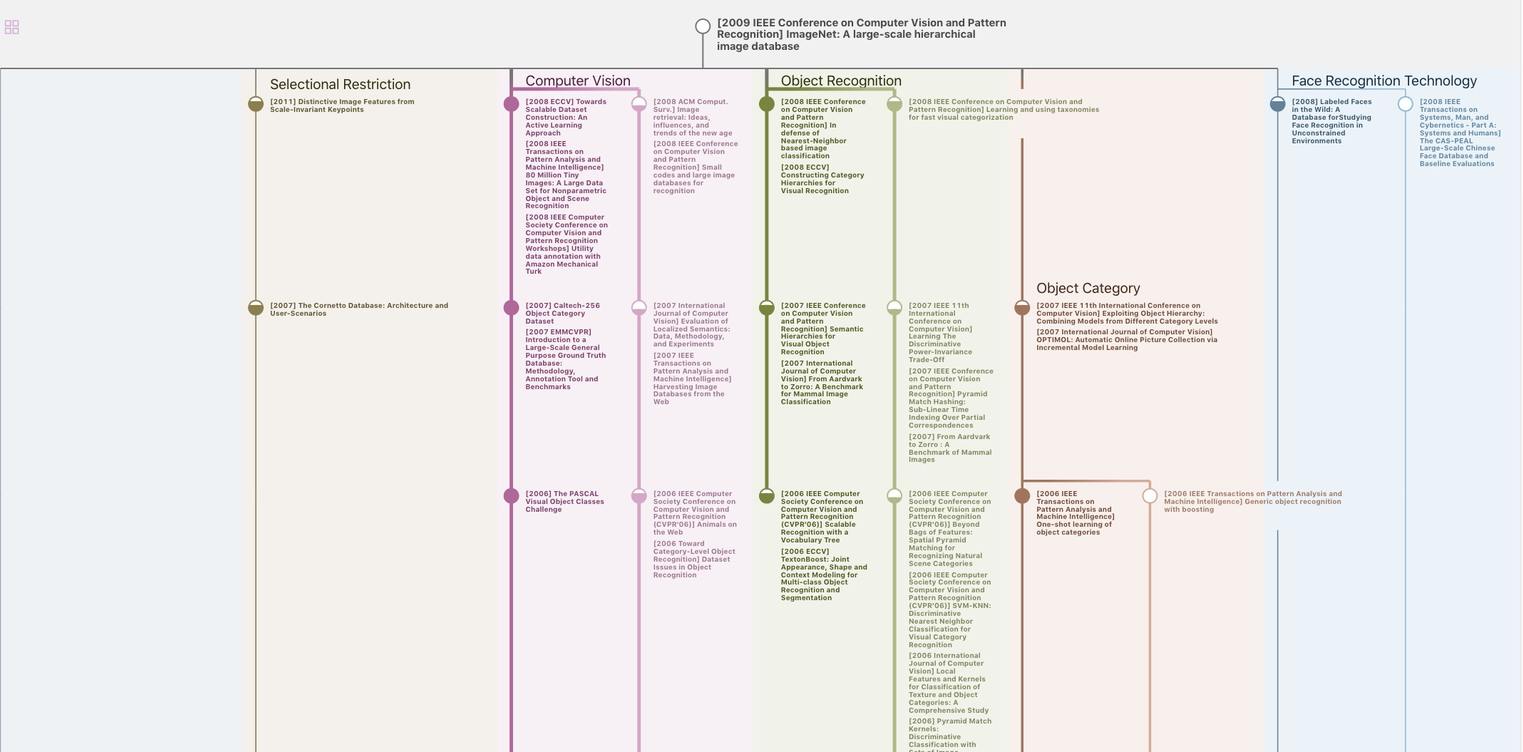
Generate MRT to find the research sequence of this paper
Chat Paper
Summary is being generated by the instructions you defined