Training Data Augmentation for Code-Mixed Translation
NAACL-HLT(2021)
摘要
Machine translation of user-generated code-mixed inputs to English is of crucial importance in applications like web search and targeted advertising. We address the scarcity of parallel training data for training such models by designing a strategy of converting existing non-code-mixed parallel data sources to code-mixed parallel data. We present an m-BERT based procedure whose core learnable component is a ternary sequence labeling model, that can be trained with a limited code-mixed corpus alone. We show a 5.8 point increase in BLEU on heavily code-mixed sentences by training a translation model using our data augmentation strategy on an Hindi-English code-mixed translation task.
更多查看译文
AI 理解论文
溯源树
样例
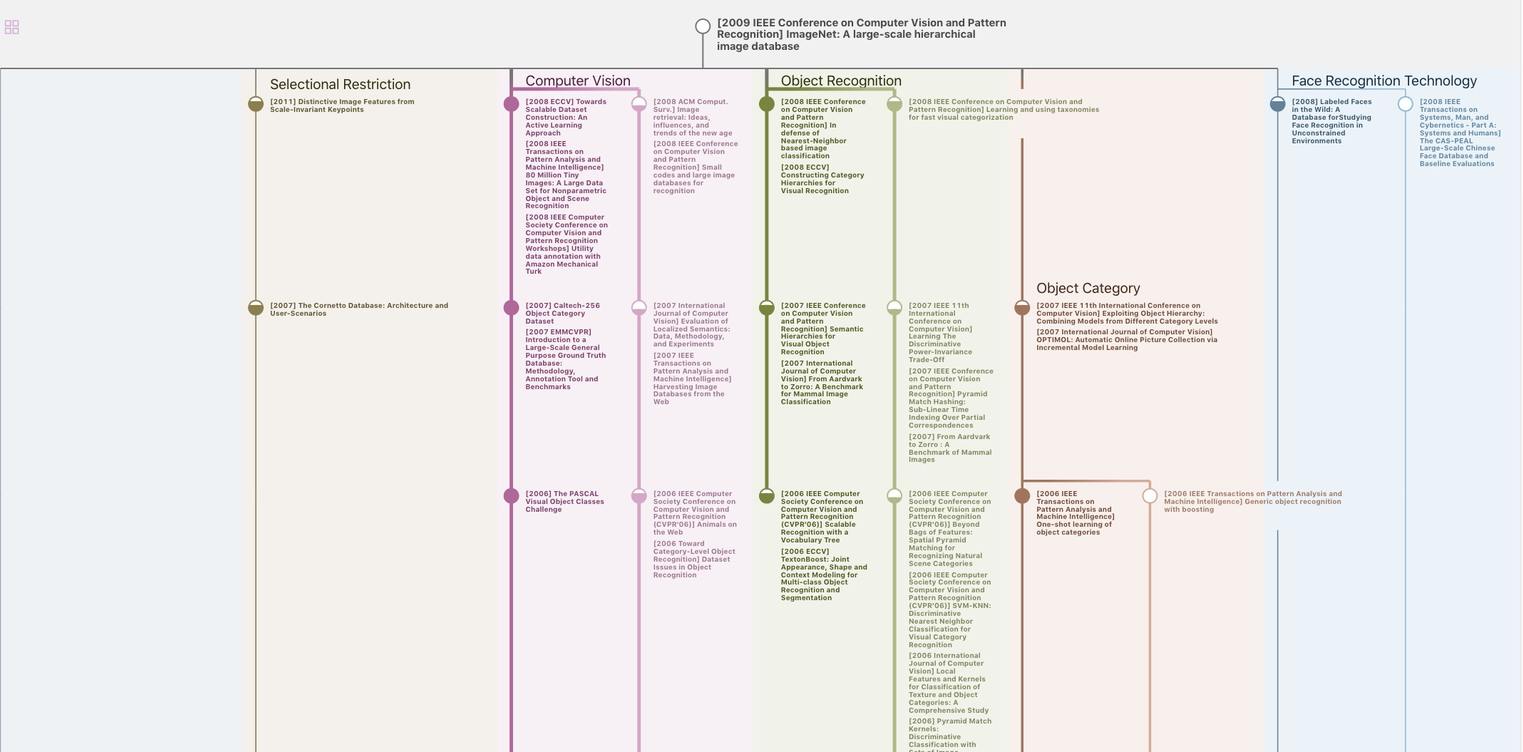
生成溯源树,研究论文发展脉络
Chat Paper
正在生成论文摘要