Land Cover-Specific Local Incidence Angle Correction: A Method For Time-Series Analysis Of Forest Ecosystems
REMOTE SENSING(2021)
摘要
This study deals with a local incidence angle correction method, i.e., the land cover-specific local incidence angle correction (LC-SLIAC), based on the linear relationship between the backscatter values and the local incidence angle (LIA) for a given land cover type in the monitored area. Using the combination of CORINE Land Cover and Hansen et al.'s Global Forest Change databases, a wide range of different LIAs for a specific forest type can be generated for each scene. The algorithm was developed and tested in the cloud-based platform Google Earth Engine (GEE) using Sentinel-1 open access data, Shuttle Radar Topography Mission (SRTM) digital elevation model, and CORINE Land Cover and Hansen et al.'s Global Forest Change databases. The developed method was created primarily for time-series analyses of forests in mountainous areas. LC-SLIAC was tested in 16 study areas over several protected areas in Central Europe. The results after correction by LC-SLIAC showed a reduction of variance and range of backscatter values. Statistically significant reduction in variance (of more than 40%) was achieved in areas with LIA range >50 degrees and LIA interquartile range (IQR) >12 degrees, while in areas with low LIA range and LIA IQR, the decrease in variance was very low and statistically not significant. Six case studies with different LIA ranges were further analyzed in pre- and post-correction time series. Time-series after the correction showed a reduced fluctuation of backscatter values caused by different LIAs in each acquisition path. This reduction was statistically significant (with up to 95% reduction of variance) in areas with a difference in LIA greater than or equal to 27 degrees. LC-SLIAC is freely available on GitHub and GEE, making the method accessible to the wide remote sensing community.
更多查看译文
关键词
local incidence angle, correction, Sentinel-1, time-series, Google Earth Engine, forest monitoring, Central Europe
AI 理解论文
溯源树
样例
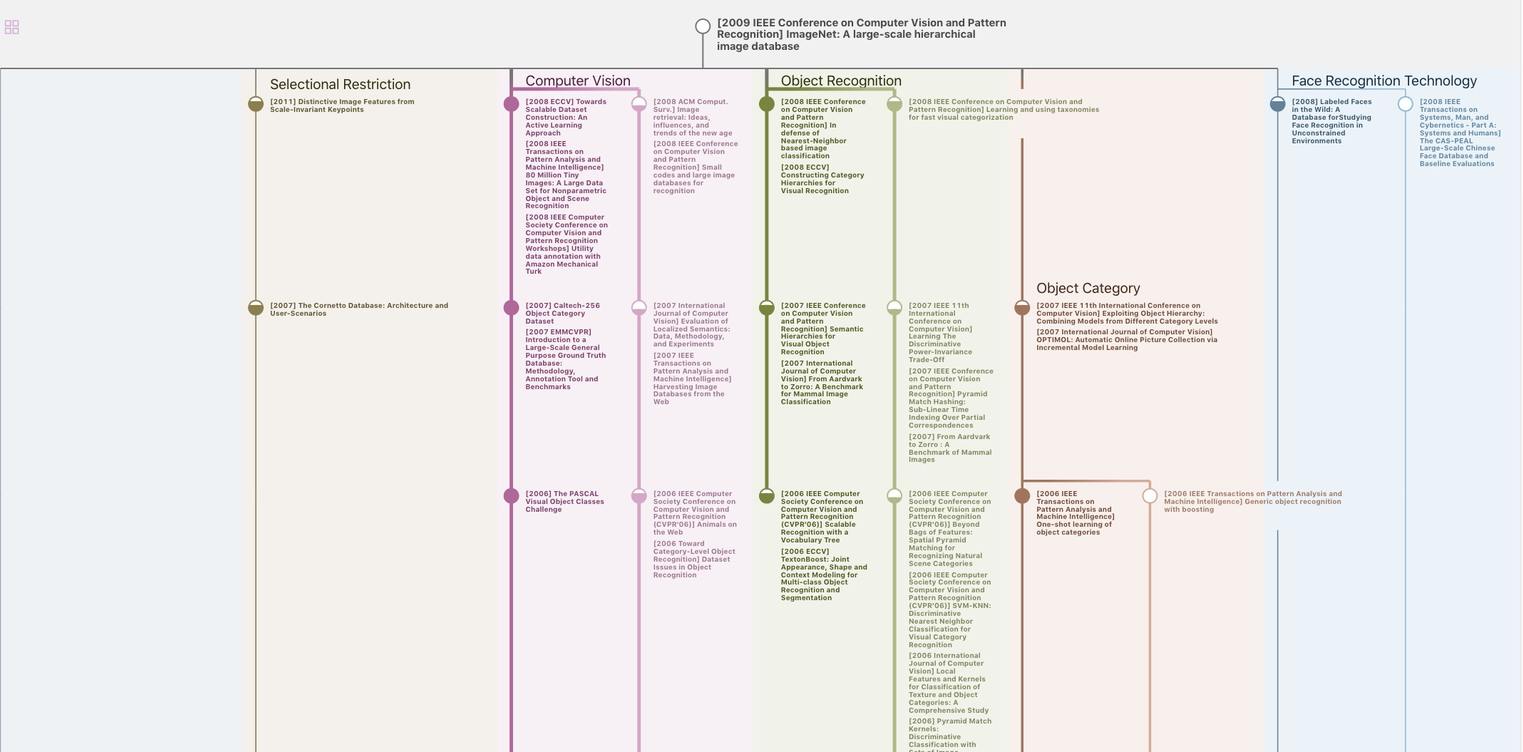
生成溯源树,研究论文发展脉络
Chat Paper
正在生成论文摘要