Ambient Pm2.5 Estimates And Variations During Covid-19 Pandemic In The Yangtze River Delta Using Machine Learning And Big Data
REMOTE SENSING(2021)
摘要
The lockdown of cities in the Yangtze River Delta (YRD) during COVID-19 has provided many natural and typical test sites for estimating the potential of air pollution control and reduction. To evaluate the reduction of PM2.5 concentration in the YRD region by the epidemic lockdown policy, this study employs big data, including PM2.5 observations and 29 independent variables regarding Aerosol Optical Depth (AOD), climate, terrain, population, road density, and Gaode map Point of interesting (POI) data, to build regression models and retrieve spatially continuous distributions of PM2.5 during COVID-19. Simulation accuracy of multiple machine learning regression models, i.e., random forest (RF), support vector regression (SVR), and artificial neural network (ANN) were compared. The results showed that the RF model outperformed the SVR and ANN models in the inversion of PM2.5 in the YRD region, with the model-fitting and cross-validation coefficients of determination R-2 reached 0.917 and 0.691, mean absolute error (MAE) values were 1.026 mu g m(-3) and 2.353 mu g m(-3), and root mean square error (RMSE) values were 1.413 mu g m(-3), and 3.144 mu g m(-3), respectively. PM2.5 concentrations during COVID-19 in 2020 have decreased by 3.61 mu g m(-3) compared to that during the same period of 2019 in the YRD region. The results of this study provide a cost-effective method of air pollution exposure assessment and help provide insight into the atmospheric changes under strong government controlling strategies.
更多查看译文
关键词
PM2 5, machine learning, COVID-19, Yangtze river delta
AI 理解论文
溯源树
样例
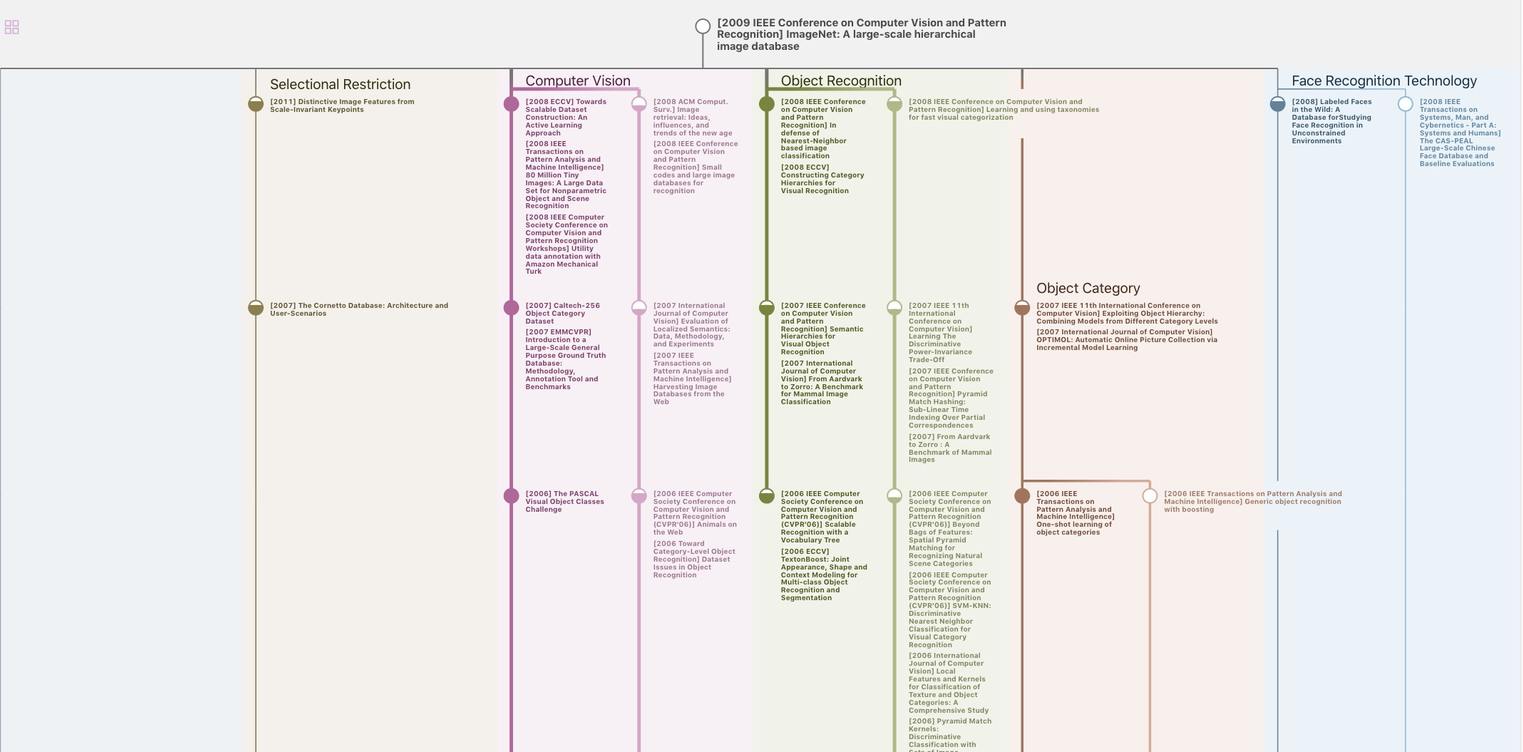
生成溯源树,研究论文发展脉络
Chat Paper
正在生成论文摘要