Deep Neural Networks and End-to-End Learning for Audio Compression
Journal of KIISE(2021)
摘要
Recent achievements in end-to-end deep learning have encouraged the exploration of tasks dealing with highly structured data with unified deep network models. Having such models for compressing audio signals has been challenging since it requires discrete representations that are not easy to train with end-to-end backpropagation. In this paper, we present an end-to-end deep learning approach that combines recurrent neural networks (RNNs) within the training strategy of variational autoencoders (VAEs) with a binary representation of the latent space. We apply a reparametrization trick for the Bernoulli distribution for the discrete representations, which allows smooth backpropagation. In addition, our approach allows the separation of the encoder and decoder, which is necessary for compression tasks. To our best knowledge, this is the first end-to-end learning for a single audio compression model with RNNs, and our model achieves a Signal to Distortion Ratio (SDR) of 20.54.
更多查看译文
关键词
neural networks,learning,end-to-end
AI 理解论文
溯源树
样例
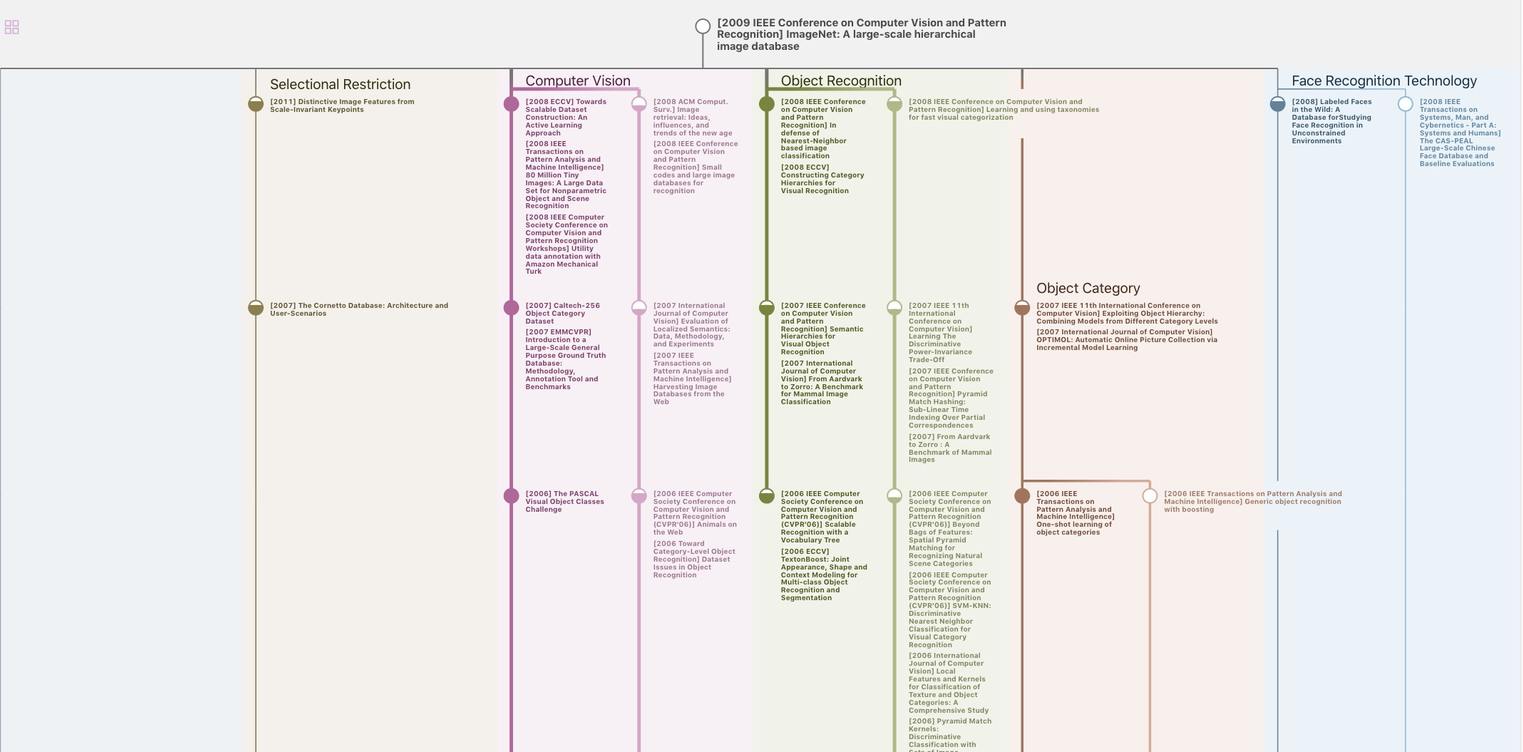
生成溯源树,研究论文发展脉络
Chat Paper
正在生成论文摘要