Guided Hyperparameter Tuning Through Visualization and Inference
CoRR(2021)
摘要
For deep learning practitioners, hyperparameter tuning for optimizing model performance can be a computationally expensive task. Though visualization can help practitioners relate hyperparameter settings to overall model performance, significant manual inspection is still required to guide the hyperparameter settings in the next batch of experiments. In response, we present a streamlined visualization system enabling deep learning practitioners to more efficiently explore, tune, and optimize hyperparameters in a batch of experiments. A key idea is to directly suggest more optimal hyperparameter values using a predictive mechanism. We then integrate this mechanism with current visualization practices for deep learning. Moreover, an analysis on the variance in a selected performance metric in the context of the model hyperparameters shows the impact that certain hyperparameters have on the performance metric. We evaluate the tool with a user study on deep learning model builders, finding that our participants have little issue adopting the tool and working with it as part of their workflow.
更多查看译文
关键词
visualization,inference
AI 理解论文
溯源树
样例
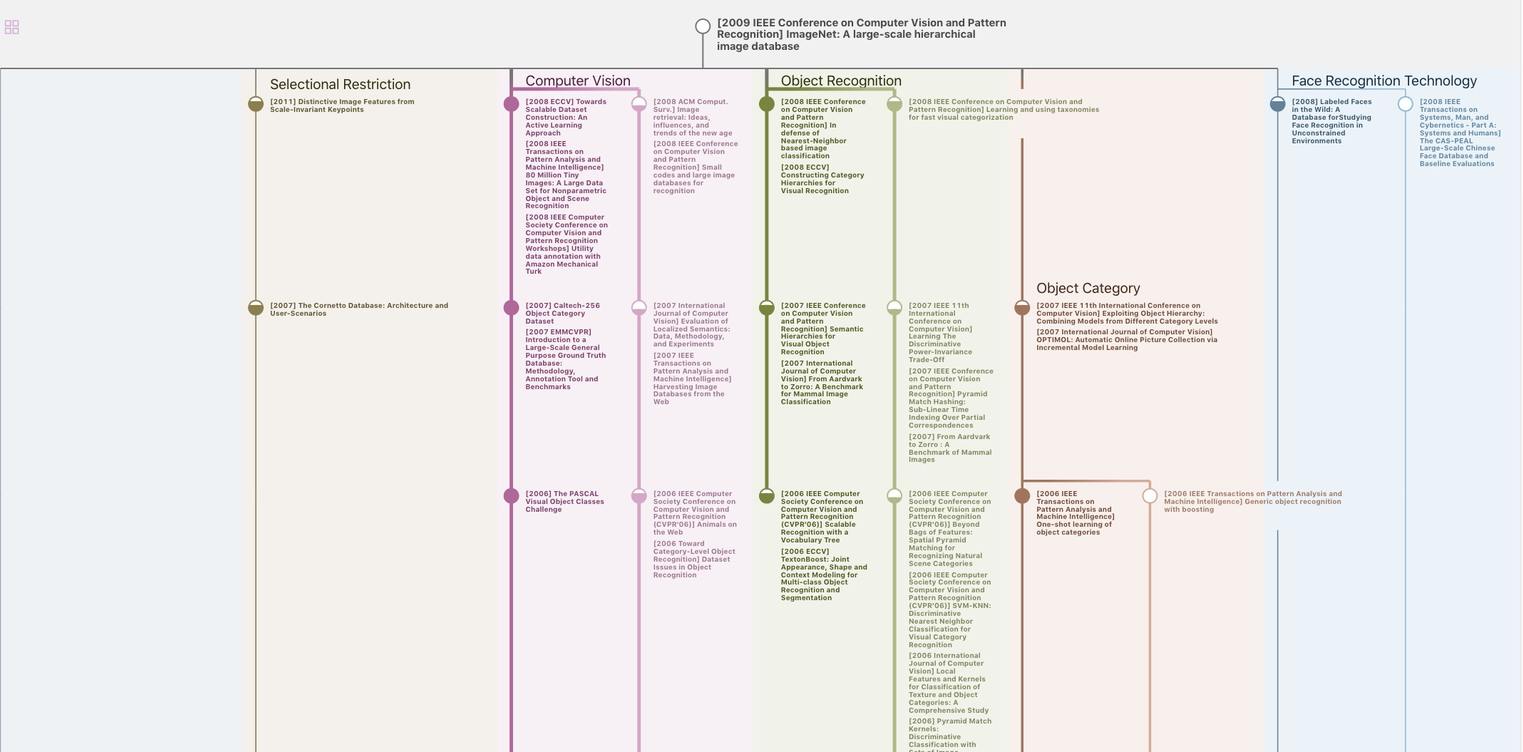
生成溯源树,研究论文发展脉络
Chat Paper
正在生成论文摘要