Dynamic Class Queue for Large Scale Face Recognition In the Wild
2021 IEEE/CVF CONFERENCE ON COMPUTER VISION AND PATTERN RECOGNITION, CVPR 2021(2021)
摘要
Learning discriminative representation using large-scale face datasets in the wild is crucial for real-world applications, yet it remains challenging. The difficulties lie in many aspects and this work focus on computing resource constraint and long-tailed class distribution. Recently, classification-based representation learning with deep neural networks and well-designed losses have demonstrated good recognition performance. However, the computing and memory cost linearly scales up to the number of identities (classes) in the training set, and the learning process suffers from unbalanced classes. In this work, we propose a dynamic class queue (DCQ) to tackle these two problems. Specifically, for each iteration during training, a subset of classes for recognition are dynamically selected and their class weights are dynamically generated on-the-fly which are stored in a queue. Since only a subset of classes is selected for each iteration, the computing requirement is reduced. By using a single server without model parallel, we empirically verify in large-scale datasets that 10% of classes are sufficient to achieve similar performance as using all classes. Moreover, the class weights are dynamically generated in a few-shot manner and therefore suitable for tail classes with only a few instances. We show clear improvement over a strong baseline in the largest public dataset Megaface Challenge2 (MF2) which has 672K identities and over 88% of them have less than 10 instances. Code is available at https ://github.com/bilylee/DCQ
更多查看译文
关键词
dynamic class queue,large scale face recognition,discriminative representation,long-tailed class distribution,classification-based representation,deep neural networks,learning process,unbalanced classes,largest public dataset,computing resource constraint,Megaface Challenge2
AI 理解论文
溯源树
样例
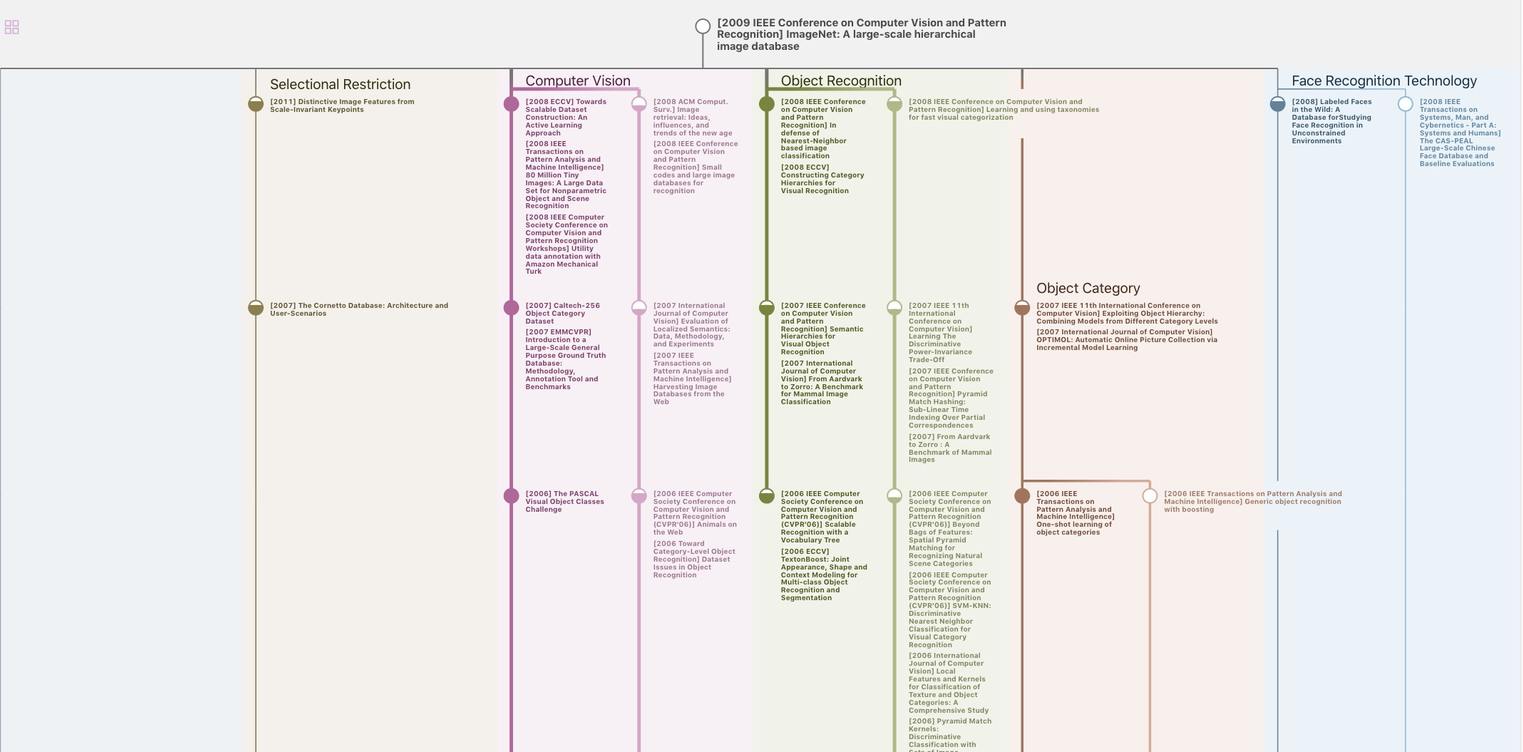
生成溯源树,研究论文发展脉络
Chat Paper
正在生成论文摘要