Federated Graph Learning -- A Position Paper
arxiv(2021)
摘要
Graph neural networks (GNN) have been successful in many fields, and derived various researches and applications in real industries. However, in some privacy sensitive scenarios (like finance, healthcare), training a GNN model centrally faces challenges due to the distributed data silos. Federated learning (FL) is a an emerging technique that can collaboratively train a shared model while keeping the data decentralized, which is a rational solution for distributed GNN training. We term it as federated graph learning (FGL). Although FGL has received increasing attention recently, the definition and challenges of FGL is still up in the air. In this position paper, we present a categorization to clarify it. Considering how graph data are distributed among clients, we propose four types of FGL: inter-graph FL, intra-graph FL and graph-structured FL, where intra-graph is further divided into horizontal and vertical FGL. For each type of FGL, we make a detailed discussion about the formulation and applications, and propose some potential challenges.
更多查看译文
关键词
graph,learning
AI 理解论文
溯源树
样例
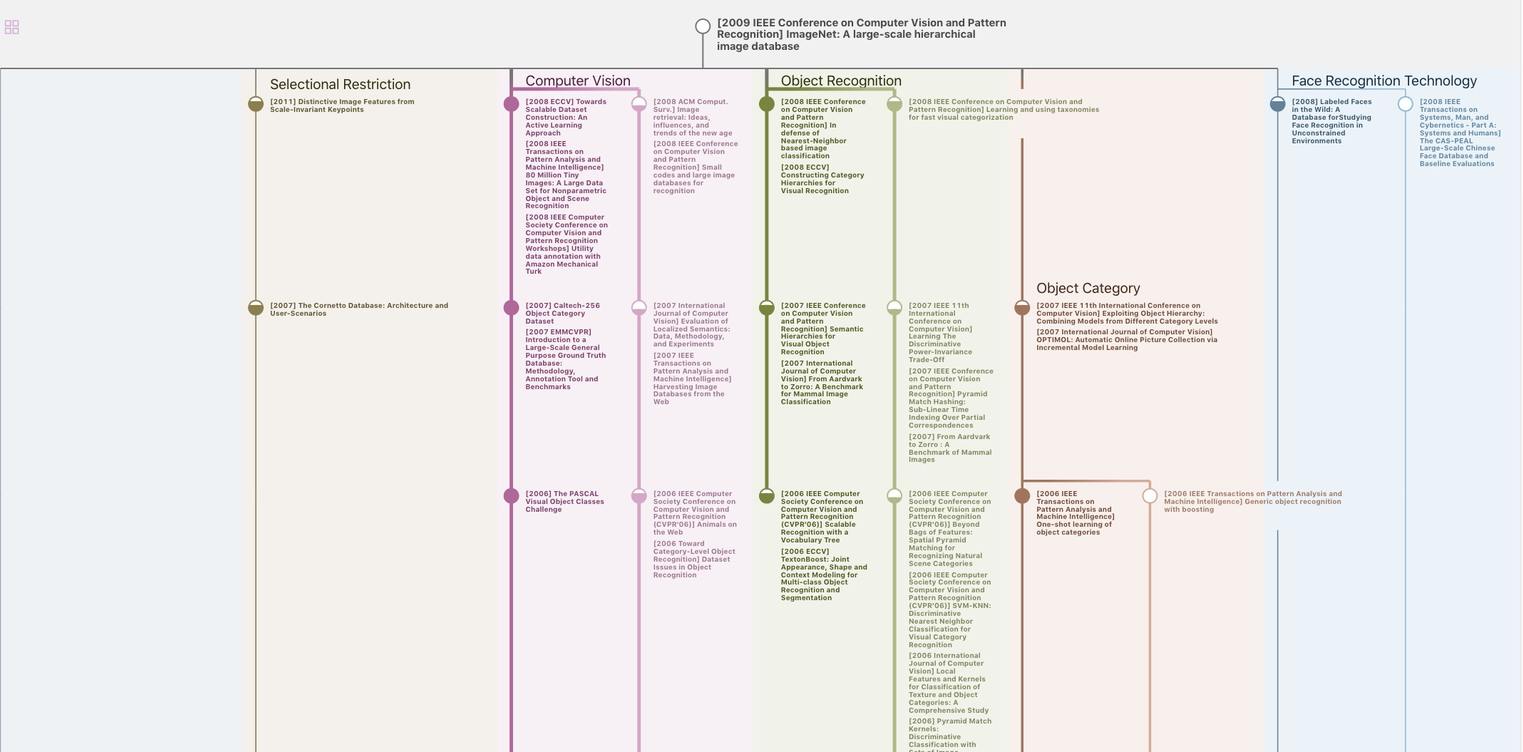
生成溯源树,研究论文发展脉络
Chat Paper
正在生成论文摘要