Can We Faithfully Represent Absence States to Compute Shapley Values on a DNN?
ArXiv(2023)
摘要
Although many methods have been proposed to estimate attributions of input variables, there still exists a significant theoretical flaw in masking-based attribution methods, i.e., it is hard to examine whether the masking method faithfully represents the absence of input variables. Specifically, for masking-based attributions, setting an input variable to the baseline value is a typical way of representing the absence of the variable. However, there are no studies investigating how to represent the absence of input variables and verify the faithfulness of baseline values. Therefore, we revisit the feature representation of a DNN in terms of causality, and propose to use causal patterns to examine whether the masking method faithfully removes information encoded in input variables. More crucially, it is proven that the causality can be explained as the elementary rationale of the Shapley value. Furthermore, we define the optimal baseline value from the perspective of causality, and we propose a method to learn the optimal baseline value. Experimental results have demonstrated the effectiveness of our method.
更多查看译文
关键词
explainable AI,attribution methods,deep neural networks
AI 理解论文
溯源树
样例
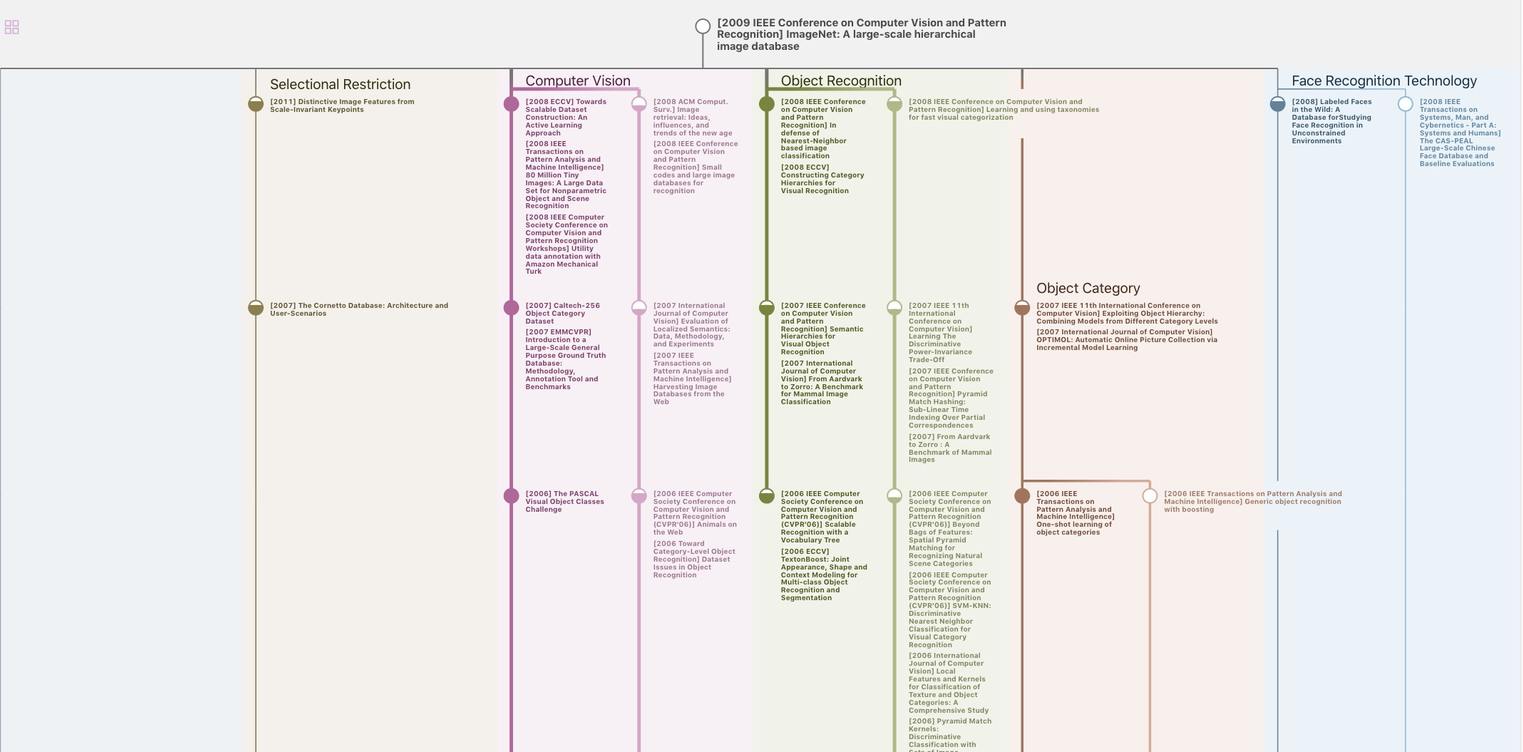
生成溯源树,研究论文发展脉络
Chat Paper
正在生成论文摘要