Symbiotic Trackers' Ensemble with Trackers' Re-initialization for Face Tracking.
SIMBig(2020)
摘要
Visual object tracking aims to deliver accurate estimates about the state of the target in a sequence of images or video frames. Nevertheless, tracking algorithms are sensitive to different kinds of image perturbations that frequently cause tracking failures. Indeed, tracking failures result from the insertion of imprecise target-related data into the trackers’ appearance models, which leads the trackers to lose the target or drift away from it. Here, we propose a tracking fusion approach, which incorporates feedback and re-initialization mechanisms to improve overall tracking performance. Our fusion technique, called SymTE-TR, enhances trackers’ overall performance by updating their appearances models with reliable information of the target’s states, while resets the imprecise trackers. We evaluated our approach on a facial video dataset, which characterizes a particular challenging tracking application under different imaging conditions. The experimental results indicate that our approach contributes to enhancing individual tracker performances by providing stable results across the video sequences and, consequently, contributes to stable overall tracking fusion performances.
更多查看译文
关键词
Online object tracking,Tracking fusion,Tracking re-initialization,Face tracking
AI 理解论文
溯源树
样例
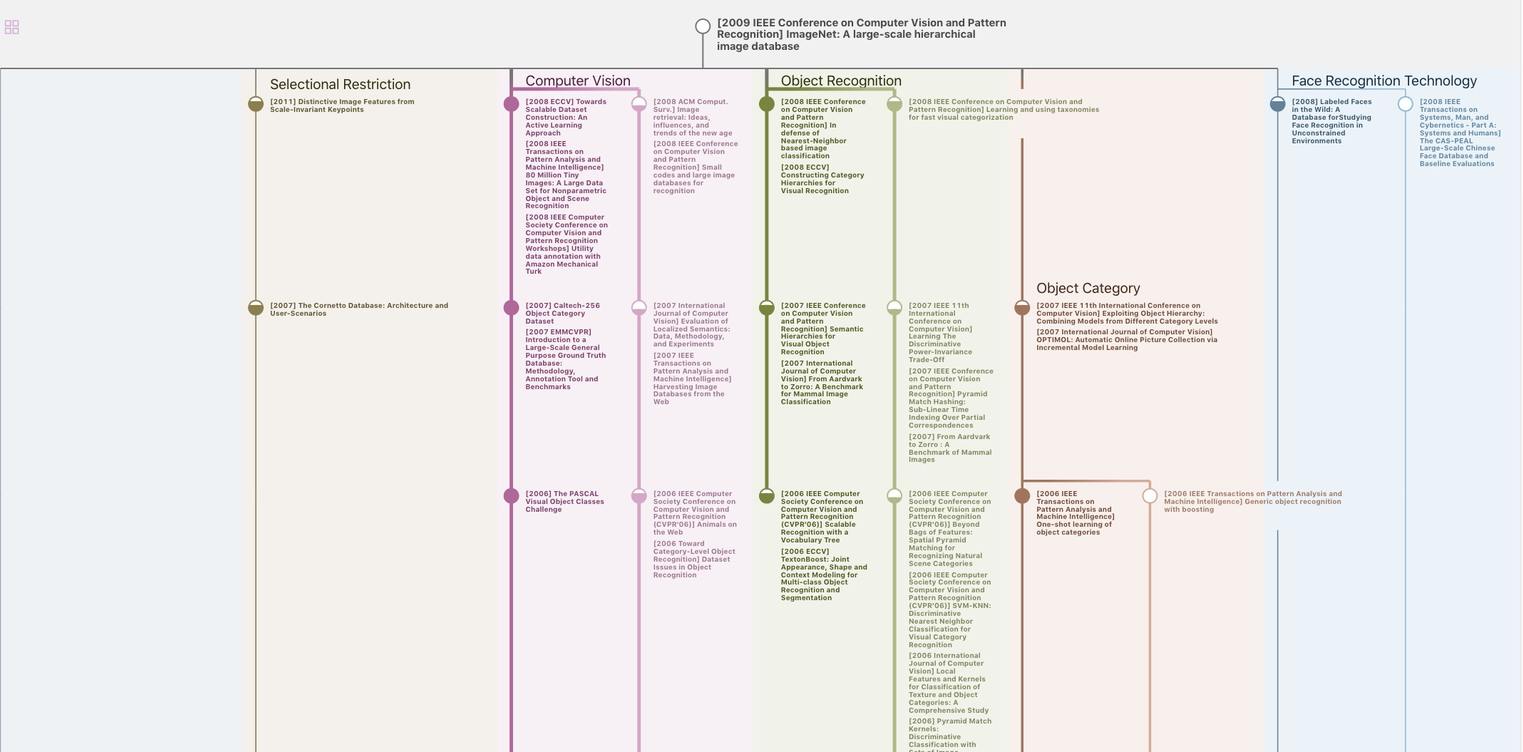
生成溯源树,研究论文发展脉络
Chat Paper
正在生成论文摘要