Robot Action Diagnosis and Experience Correction by Falsifying Parameterised Execution Models
2021 IEEE INTERNATIONAL CONFERENCE ON ROBOTICS AND AUTOMATION (ICRA 2021)(2021)
摘要
When faced with an execution failure, an intelligent robot should be able to identify the likely reasons for the failure and adapt its execution policy accordingly. This paper addresses the question of how to utilise knowledge about the execution process, expressed in terms of learned constraints, in order to direct the diagnosis and experience acquisition process. In particular, we present two methods for creating a synergy between failure diagnosis and execution model learning. We first propose a method for diagnosing execution failures of parameterised action execution models, which searches for action parameters that violate a learned precondition model. We then develop a strategy that uses the results of the diagnosis process for generating synthetic data that are more likely to lead to successful execution, thereby increasing the set of available experiences to learn from. The diagnosis and experience correction methods are evaluated for the problem of handle grasping, such that we experimentally demonstrate the effectiveness of the diagnosis algorithm and show that corrected failed experiences can contribute towards improving the execution success of a robot.
更多查看译文
关键词
robot action diagnosis,experience correction,execution failure,intelligent robot,execution policy,execution process,constraint learning,experience acquisition process,failure diagnosis,parameterised action execution models,diagnosis process,diagnosis algorithm,parameterised execution model falsification,precondition model learning,handle grasping
AI 理解论文
溯源树
样例
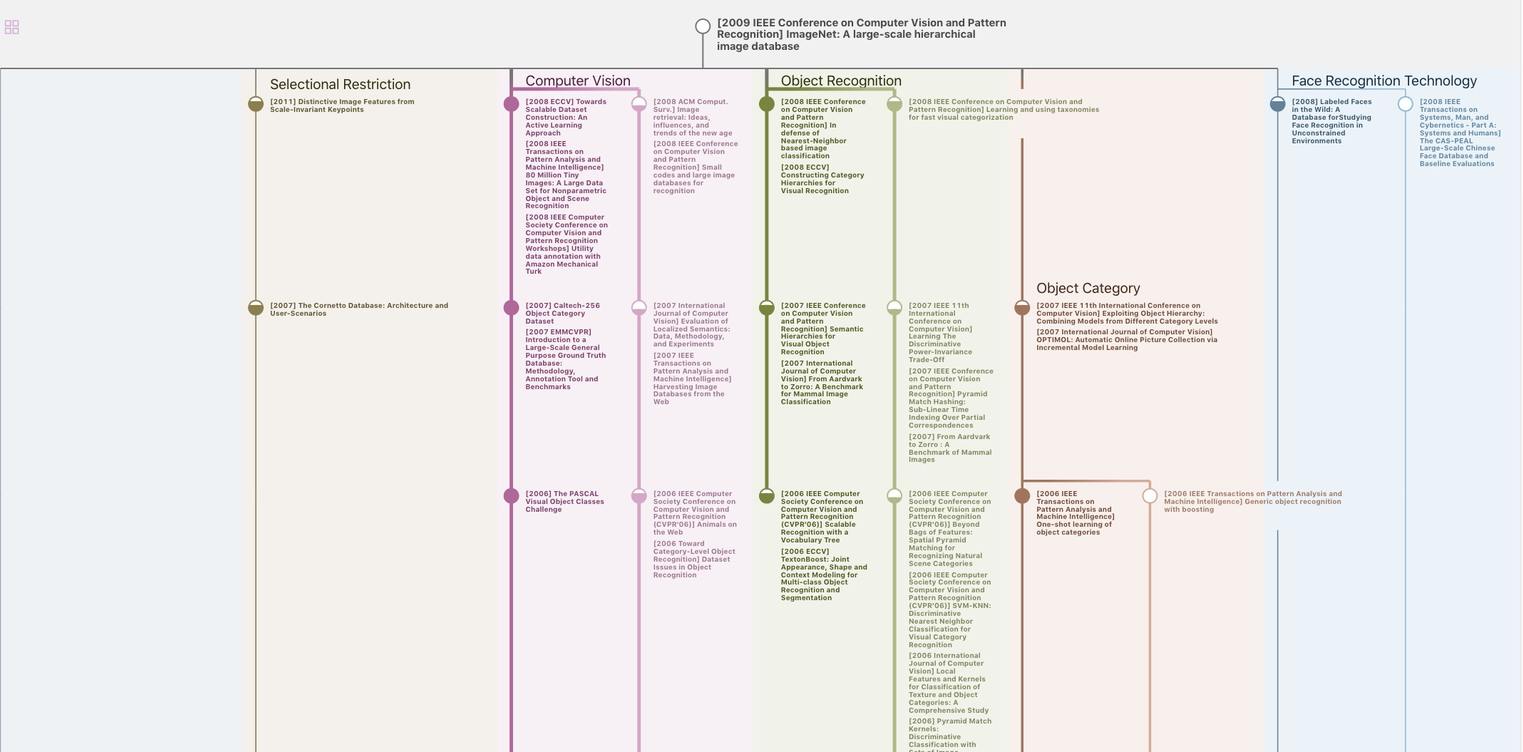
生成溯源树,研究论文发展脉络
Chat Paper
正在生成论文摘要