Towards Improving Privacy of Synthetic DataSets.
APF(2021)
摘要
Recent growth in domain specific applications of machine learning can be attributed to availability of realistic public datasets. Real world datasets may always contain sensitive information about the users, which makes it hard to share freely with other stake holders, and researchers due to regulatory and compliance requirements. Synthesising datasets from real data by leveraging generative techniques is gaining popularity. However, the privacy analysis of these dataset is still a open research. In this work, we fill this gap by investigating the privacy issues of the generated data sets from attacker and auditor point of view. We propose instance level Privacy Score (PS) for each synthetic sample by measuring the memorisation coefficient α m per sample. Leveraging, PS we empirically show that accuracy of membership inference attacks on synthetic data drop significantly. PS is a model agnostic, post training measure, which helps data sharer with guidance about the privacy properties of a given sample but also helps third party data auditors to run privacy checks without sharing model internals. We tested our method on two real world data sets and show that attack accuracy reduced by PS based filtering.
更多查看译文
关键词
Privacy preserving synthetic data, Generative Adversarial Networks, Privacy audit
AI 理解论文
溯源树
样例
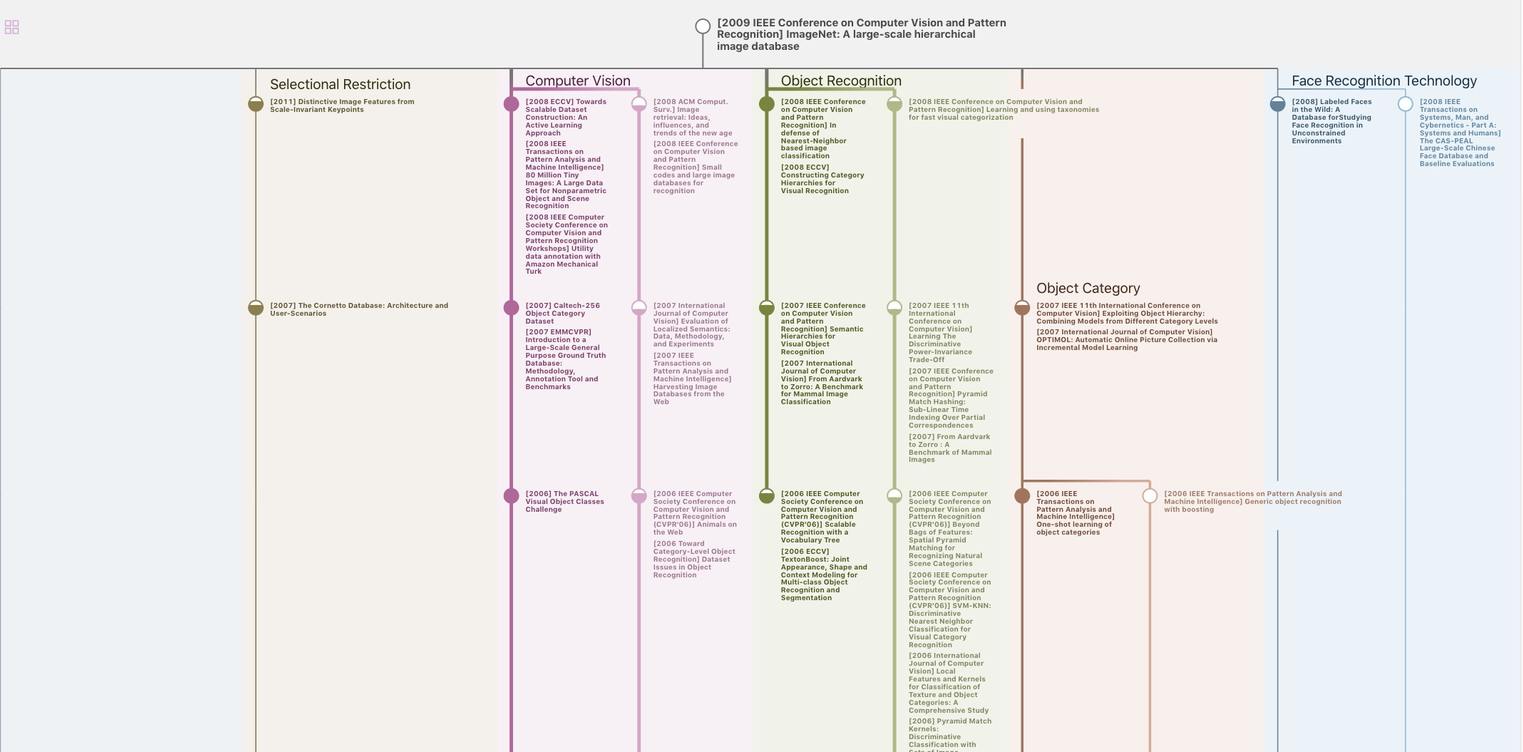
生成溯源树,研究论文发展脉络
Chat Paper
正在生成论文摘要