Lessons Learned Addressing Dataset Bias in Model-Based Candidate Generation at Twitter
arXiv (Cornell University)(2021)
Abstract
Traditionally, heuristic methods are used to generate candidates for large scale recommender systems. Model-based candidate generation promises multiple potential advantages, primarily that we can explicitly optimize the same objective as the downstream ranking model. However, large scale model-based candidate generation approaches suffer from dataset bias problems caused by the infeasibility of obtaining representative data on very irrelevant candidates. Popular techniques to correct dataset bias, such as inverse propensity scoring, do not work well in the context of candidate generation. We first explore the dynamics of the dataset bias problem and then demonstrate how to use random sampling techniques to mitigate it. Finally, in a novel application of fine-tuning, we show performance gains when applying our candidate generation system to Twitter's home timeline.
MoreTranslated text
Key words
candidate generation,dataset bias,twitter,model-based
AI Read Science
Must-Reading Tree
Example
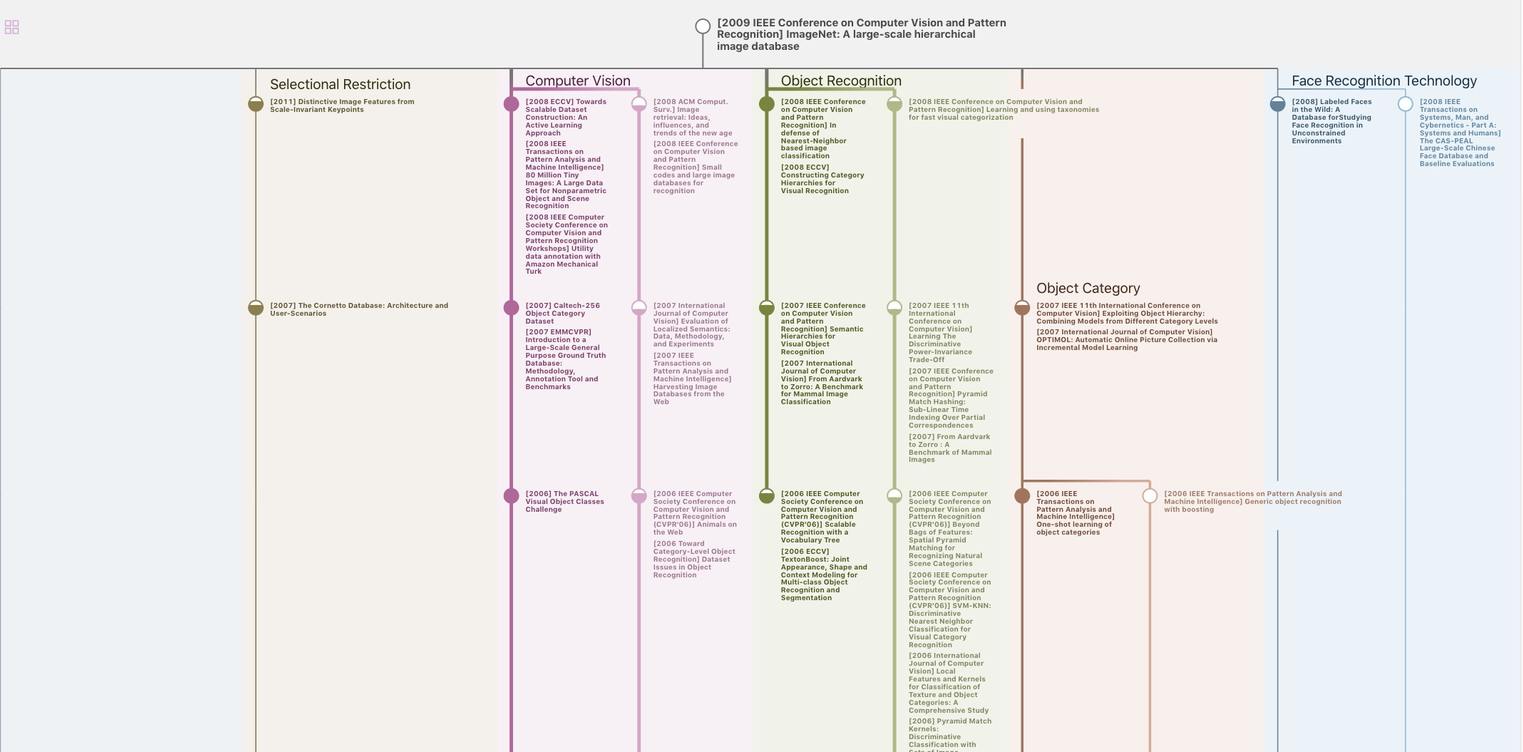
Generate MRT to find the research sequence of this paper
Chat Paper
Summary is being generated by the instructions you defined