Attention-based spatial-temporal graph transformer for traffic flow forecasting
Neural Comput. Appl.(2023)
Abstract
Traffic forecasting is significant for establishing intelligent traffic systems. However, the complex spatial-temporal relationships of traffic flow data make it challenging. Despite some studies investigating this problem, capturing complex spatial structures and learning the temporal dependence of the long-term prediction are still challenges that need to be addressed. In this paper, we propose a novel attention-based spatial-temporal graph transformer (ASTGT) model to solve the traffic flow forecasting problem. ASTGT primarily consists of two independent components: temporal encoder and spatial-temporal decoder. Precisely, in temporal encoder, we capture the temporal dynamics of traffic data through the self-attention mechanism. The traffic flow data with long-term periodic dependence are also integrated. Moreover, spatial-temporal decoder superimposes the gated spatial graph convolution block to acquire both temporal and spatial relationships at the same time. Extensive experiments are conducted on PEMS04 and PEMS08 datasets from the Caltrans Performance Measurement System. The results show that our proposed ASTGT model outperforms the state-of-the-art baselines.
MoreTranslated text
Key words
forecasting,graph,flow,attention-based,spatial-temporal
AI Read Science
Must-Reading Tree
Example
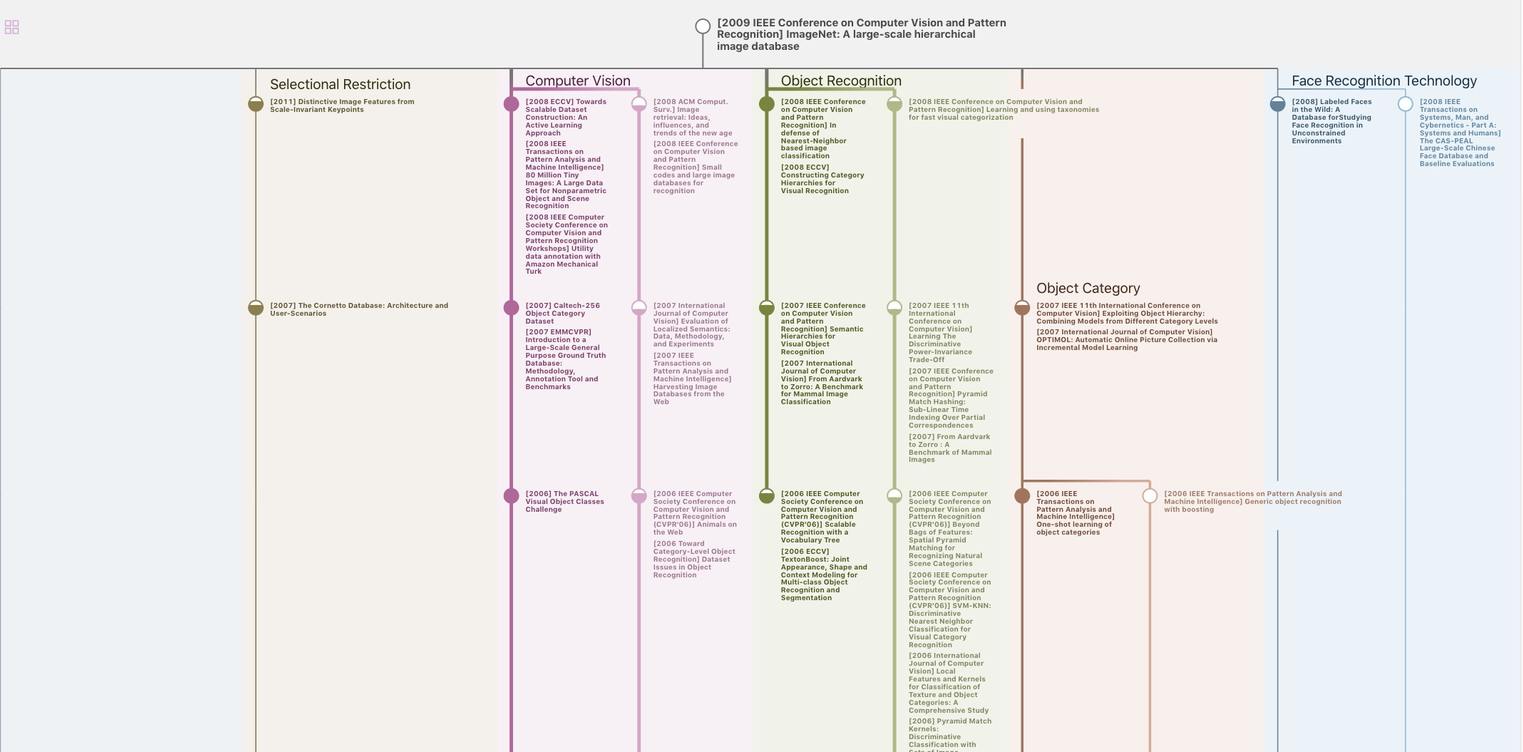
Generate MRT to find the research sequence of this paper
Chat Paper
Summary is being generated by the instructions you defined