Provable Guarantees on the Robustness of Decision Rules to Causal Interventions.
IJCAI(2021)
摘要
Robustness of decision rules to shifts in the data-generating process is crucial to the successful deployment of decision-making systems. Such shifts can be viewed as interventions on a causal graph, which capture (possibly hypothetical) changes in the data-generating process, whether due to natural reasons or by the action of an adversary. We consider causal Bayesian networks and formally define the interventional robustness problem, a novel model-based notion of robustness for decision functions that measures worst-case performance with respect to a set of interventions that denote changes to parameters and/or causal influences. By relying on a tractable representation of Bayesian networks as arithmetic circuits, we provide efficient algorithms for computing guaranteed upper and lower bounds on the interventional robustness probabilities. Experimental results demonstrate that the methods yield useful and interpretable bounds for a range of practical networks, paving the way towards provably causally robust decision-making systems.
更多查看译文
关键词
provable guarantees,robustness,causal
AI 理解论文
溯源树
样例
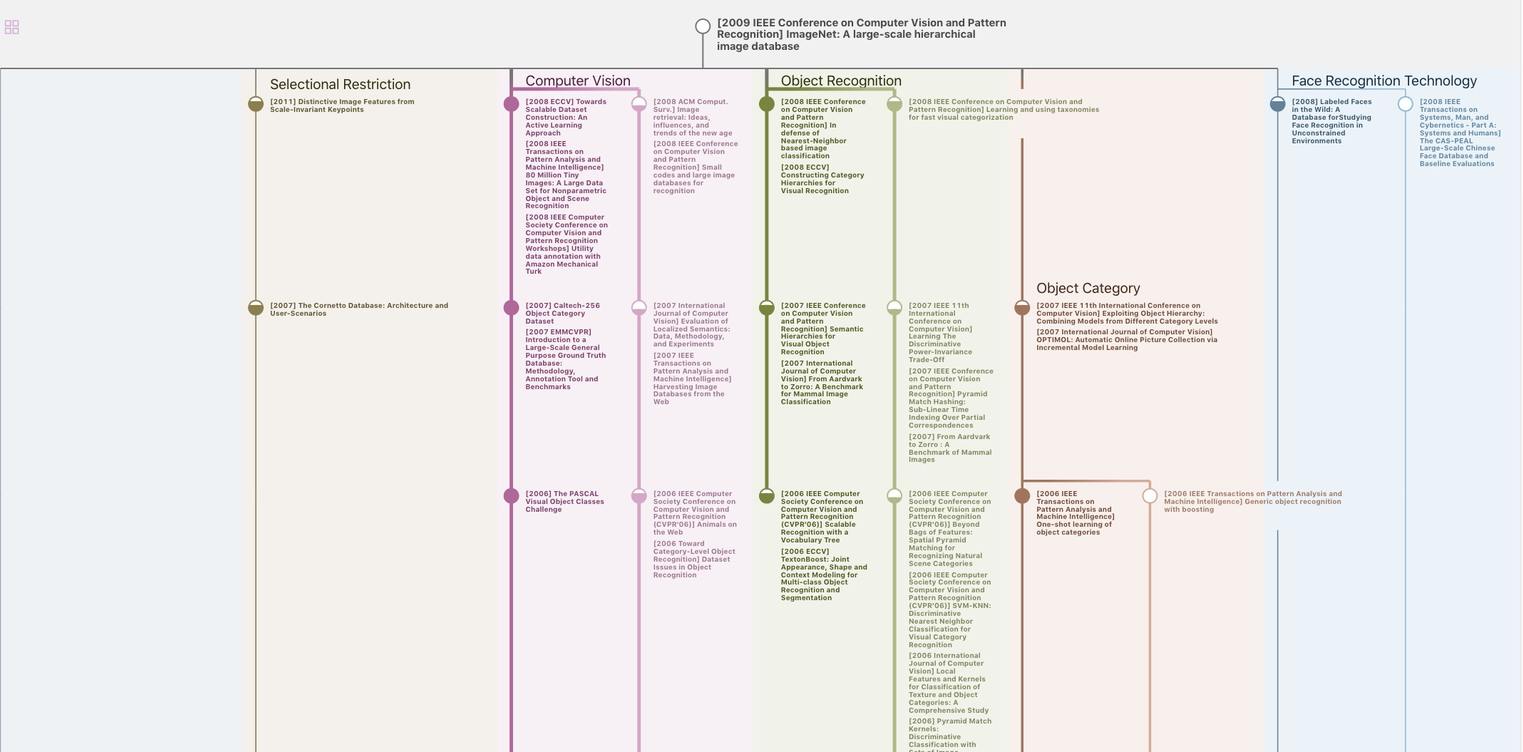
生成溯源树,研究论文发展脉络
Chat Paper
正在生成论文摘要