Mill.jl and JsonGrinder.jl: automated differentiable feature extraction for learning from raw JSON data
arXiv (Cornell University)(2021)
摘要
Learning from raw data input, thus limiting the need for manual feature engineering, is one of the key components of many successful applications of machine learning methods. While machine learning problems are often formulated on data that naturally translate into a vector representation suitable for classifiers, there are data sources, for example in cybersecurity, that are naturally represented in diverse files with a unifying hierarchical structure, such as XML, JSON, and Protocol Buffers. Converting this data to vector (tensor) representation is generally done by manual feature engineering, which is laborious, lossy, and prone to human bias about the importance of particular features. Mill and JsonGrinder is a tandem of libraries, which fully automates the conversion. Starting with an arbitrary set of JSON samples, they create a differentiable machine learning model capable of infer from further JSON samples in their raw form.
更多查看译文
关键词
raw jsongrinderjl data,differentiable feature extraction
AI 理解论文
溯源树
样例
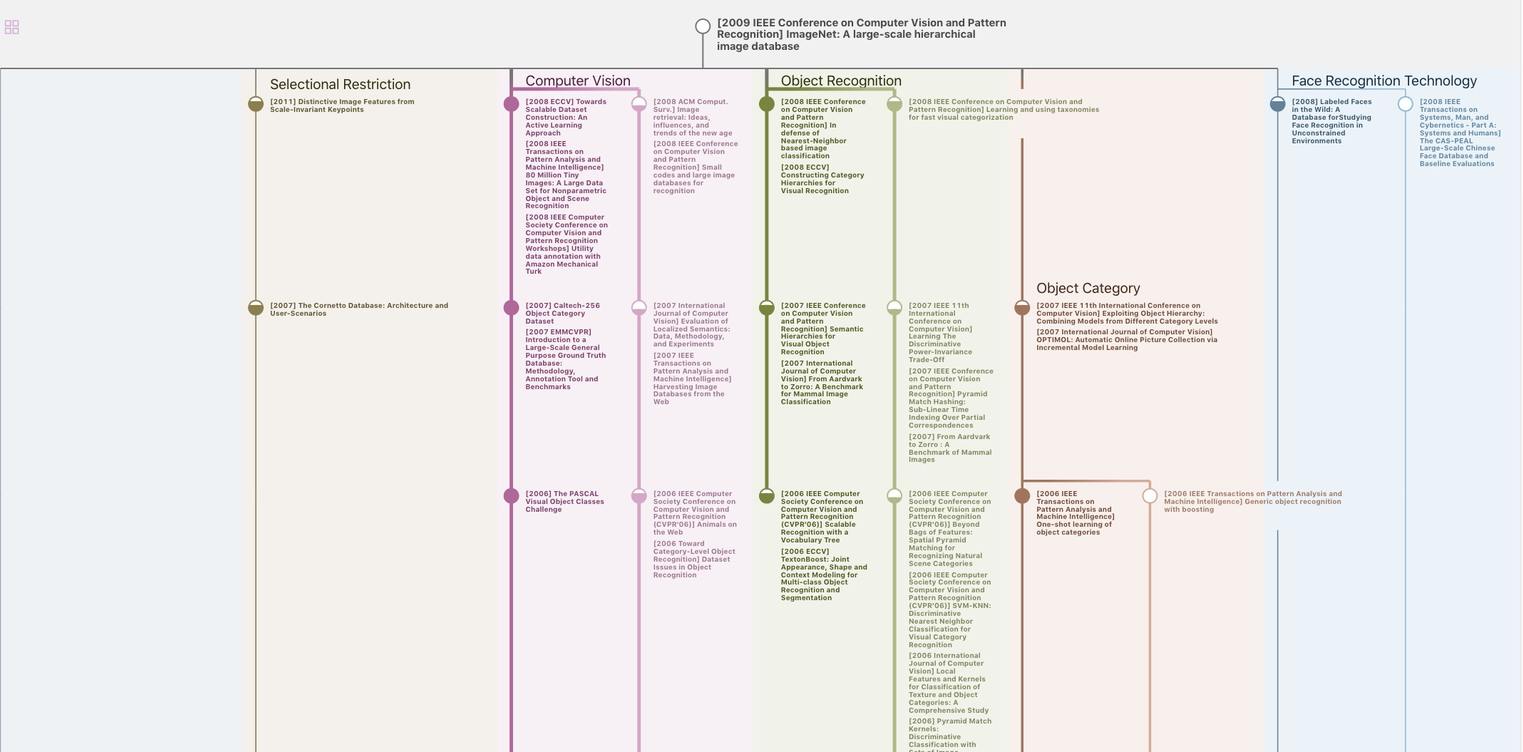
生成溯源树,研究论文发展脉络
Chat Paper
正在生成论文摘要