Probabilistic robustness estimates for feed-forward neural networks
Neural Networks(2021)
摘要
Robustness of deep neural networks is a critical issue in practical applications. In the general case of feed-forward neural networks (including convolutional deep neural network architectures), under random noise attacks, we propose to study the probability that the output of the network deviates from its nominal value by a given threshold. We derive a simple concentration inequality for the propagation of the input uncertainty through the network using the Cramer–Chernoff method and estimates of the local variation of the neural network mapping computed at the training points. We further discuss and exploit the resulting condition on the network to regularize the loss function during training. Finally, we assess the proposed tail probability estimates empirically on various public datasets and show that the observed robustness is very well estimated by the proposed method.
更多查看译文
关键词
Deep neural network,Neural network robustness,Random noise attacks,Regularization
AI 理解论文
溯源树
样例
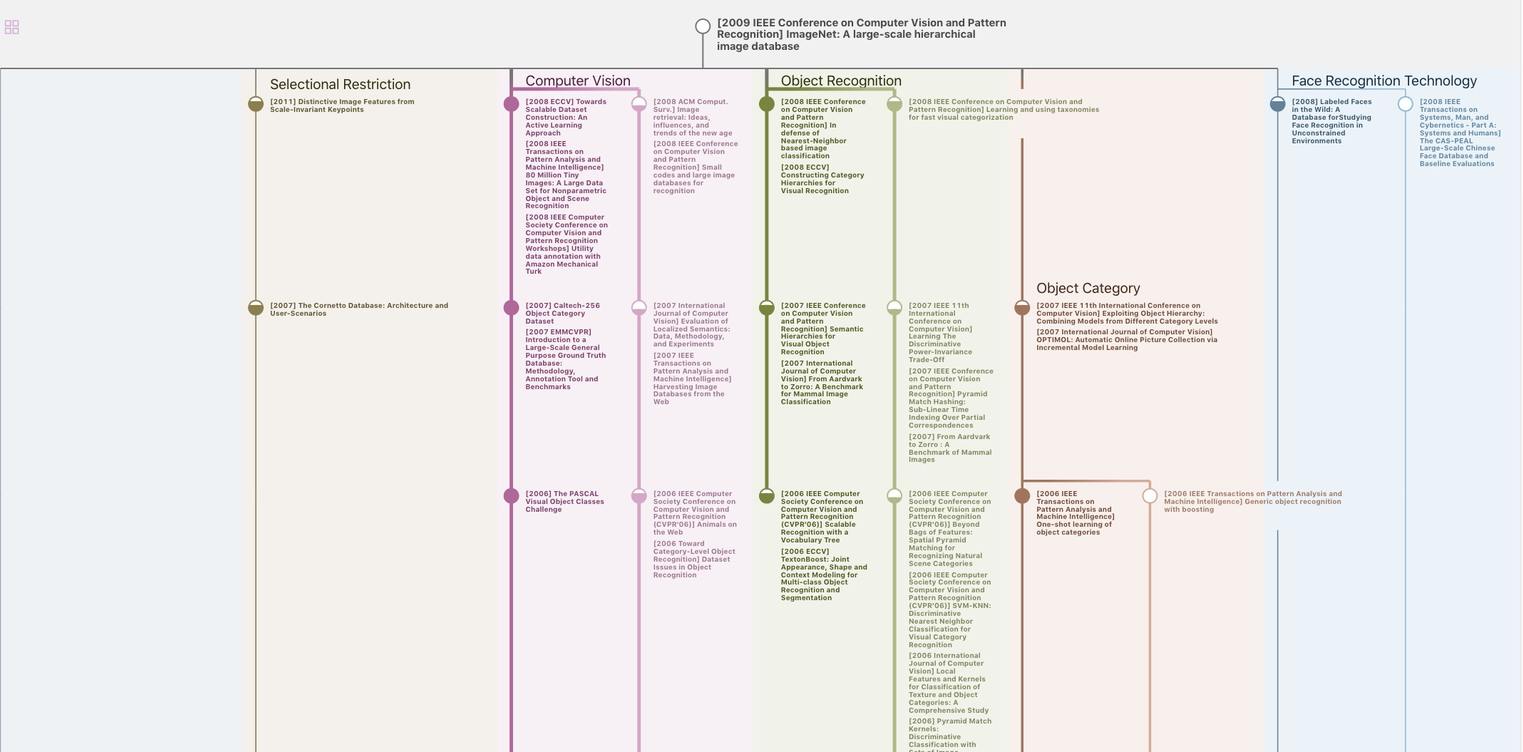
生成溯源树,研究论文发展脉络
Chat Paper
正在生成论文摘要