Power Grid Reliability Estimation Via Adaptive Importance Sampling
IEEE CONTROL SYSTEMS LETTERS(2022)
摘要
Electricity production currently generates approximately 25% of greenhouse gas emissions in the USA. Thus, increasing the amount of renewable energy is a key step to carbon neutrality. However, integrating a large amount of fluctuating renewable generation is a significant challenge for power grid operating and planning. Grid reliability, i.e., an ability to meet operational constraints under power fluctuations, is probably the most important of them. In this letter, we propose computationally efficient and accurate methods to estimate the probability of line overflow, i.e., reliability constraints violation, under a known distribution of renewable energy generation. To this end, we investigate an importance sampling approach, a flexible extension of Monte-Carlo methods, which adaptively changes the sampling distribution to generate more samples near the reliability boundary. The approach allows to estimate overload probability in real-time based only on a few dozens of random samples, compared to thousands required by the plain Monte-Carlo. Our study focuses on high voltage direct current power transmission grids with linear reliability constraints on power injections and line currents. We propose a novel theoretically justified physics-informed adaptive importance sampling algorithm and compare its performance to state-of-the-art methods on multiple IEEE power grid test cases.
更多查看译文
关键词
Power system reliability, Monte Carlo methods, Power grids, Estimation, Uncertainty, Reliability theory, Probability density function, Power system security, power system control, sampling methods, fluctuations
AI 理解论文
溯源树
样例
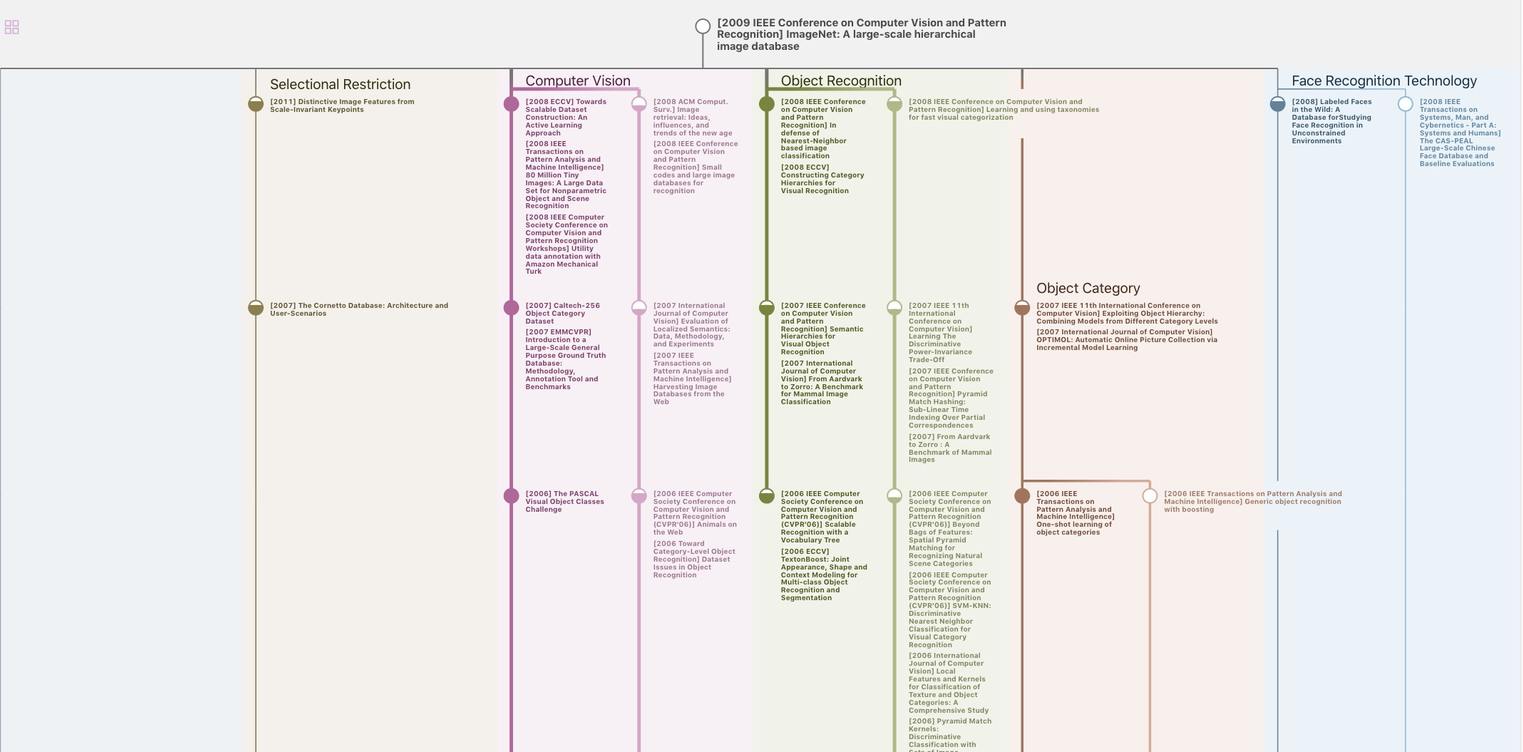
生成溯源树,研究论文发展脉络
Chat Paper
正在生成论文摘要