Quantum imaginary time evolution steered by reinforcement learning
Communications Physics(2022)
Abstract
The quantum imaginary time evolution is a powerful algorithm for preparing the ground and thermal states on near-term quantum devices. However, algorithmic errors induced by Trotterization and local approximation severely hinder its performance. Here we propose a deep reinforcement learning-based method to steer the evolution and mitigate these errors. In our scheme, the well-trained agent can find the subtle evolution path where most algorithmic errors cancel out, enhancing the fidelity significantly. We verified the method’s validity with the transverse-field Ising model and the Sherrington-Kirkpatrick model. Numerical calculations and experiments on a nuclear magnetic resonance quantum computer illustrate the efficacy. The philosophy of our method, eliminating errors with errors, sheds light on error reduction on near-term quantum devices.
MoreTranslated text
Key words
Information theory and computation,Quantum information,Physics,general
AI Read Science
Must-Reading Tree
Example
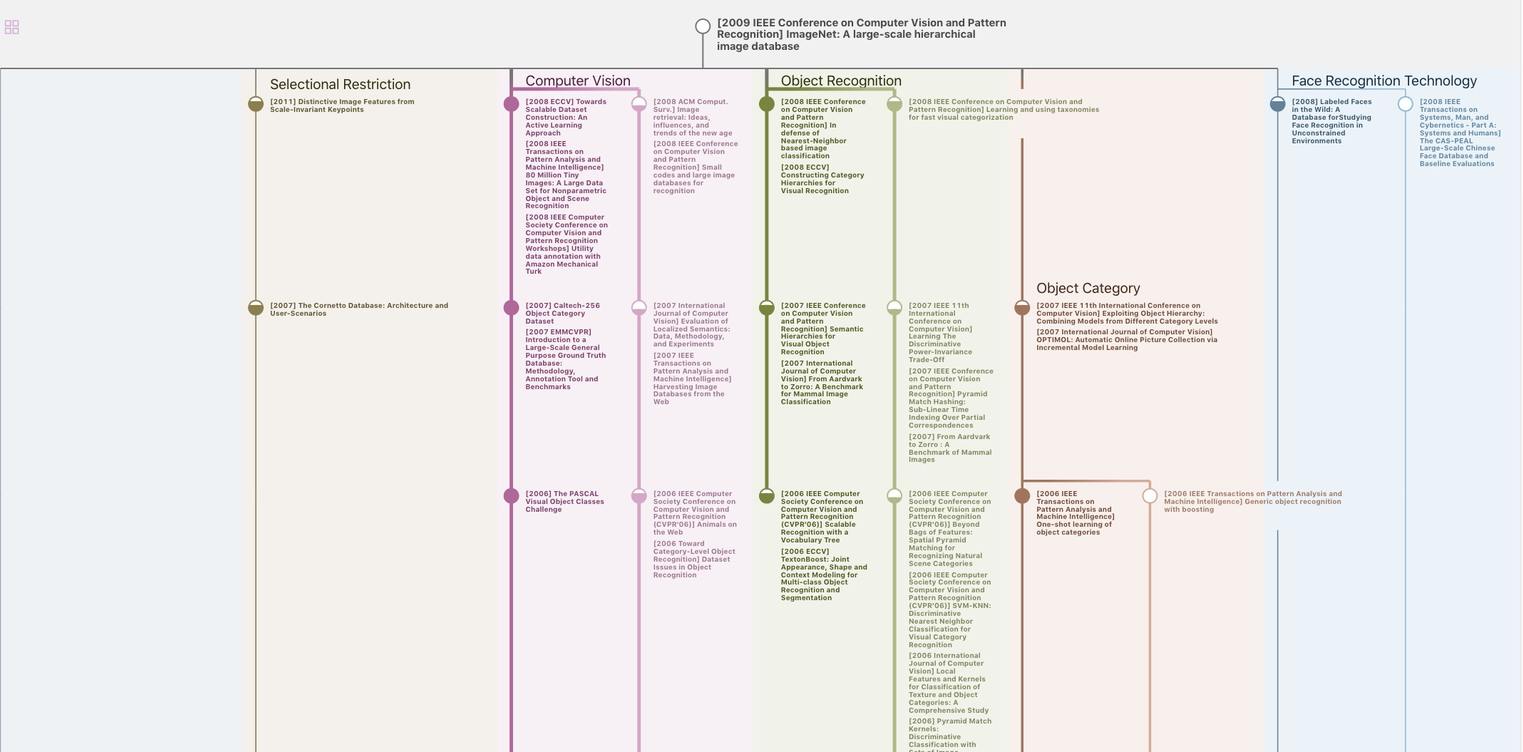
Generate MRT to find the research sequence of this paper
Chat Paper
Summary is being generated by the instructions you defined