Robust Physics-Based Manipulation by Interleaving Open and Closed-Loop Execution
arxiv(2021)
摘要
We present a planning and control framework for physics-based manipulation under uncertainty. The key idea is to interleave robust open-loop execution with closed-loop control. We derive robustness metrics through contraction theory. We use these metrics to plan trajectories that are robust to both state uncertainty and model inaccuracies. However, fully robust trajectories are extremely difficult to find or may not exist for many multi-contact manipulation problems. We separate a trajectory into robust and non-robust segments through a minimum cost path search on a robustness graph. Robust segments are executed open-loop and non-robust segments are executed with model-predictive control. We conduct experiments on a real robotic system for reaching in clutter. Our results suggest that the open and closed-loop approach results in up to 35% more real-world success compared to open-loop baselines and a 40% reduction in execution time compared to model-predictive control. We show for the first time that partially open-loop manipulation plans generated with our approach reach similar success rates to model-predictive control, while achieving a more fluent/real-time execution. A video showing real-robot executions can be found at https://youtu.be/rPOPCwHfV4g.
更多查看译文
关键词
manipulation,interleaving open,physics-based,closed-loop
AI 理解论文
溯源树
样例
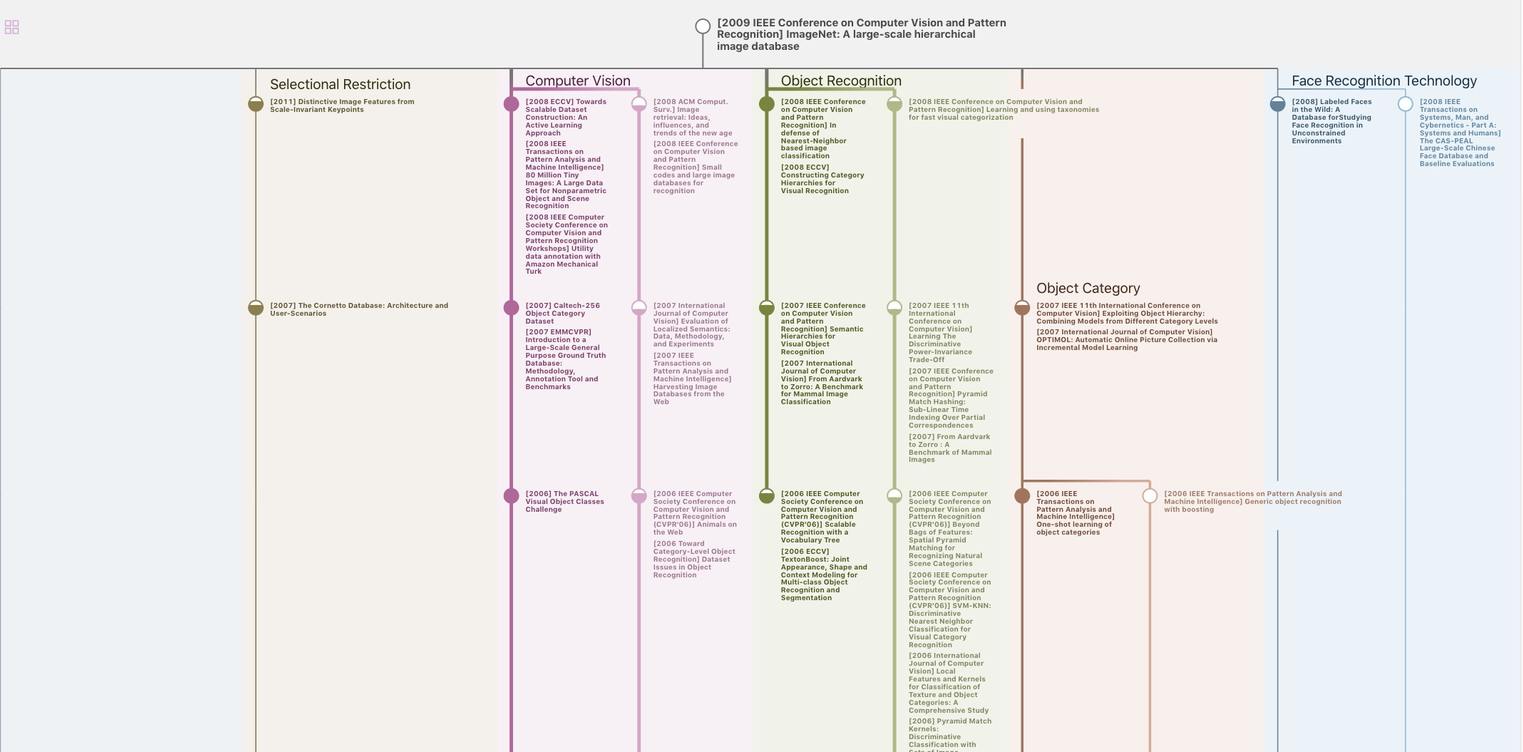
生成溯源树,研究论文发展脉络
Chat Paper
正在生成论文摘要