Towards Demystifying Serverless Machine Learning Training
International Conference on Management of Data(2021)
摘要
ABSTRACTThe appeal of serverless (FaaS) has triggered a growing interest on how to use it in data-intensive applications such as ETL, query processing, or machine learning (ML). Several systems exist for training large-scale ML models on top of serverless infrastructures (e.g., AWS Lambda) but with inconclusive results in terms of their performance and relative advantage over "serverful" infrastructures (IaaS). In this paper we present a systematic, comparative study of distributed ML training over FaaS and IaaS. We present a design space covering design choices such as optimization algorithms and synchronization protocols, and implement a platform, LambdaML, that enables a fair comparison between FaaS and IaaS. We present experimental results using LambdaML, and further develop an analytic model to capture cost/performance tradeoffs that must be considered when opting for a serverless infrastructure. Our results indicate that ML training pays off in serverless only for models with efficient (i.e., reduced) communication and that quickly converge. In general, FaaS can be much faster but it is never significantly cheaper than IaaS.
更多查看译文
关键词
Serverless Computing, Machine Learning
AI 理解论文
溯源树
样例
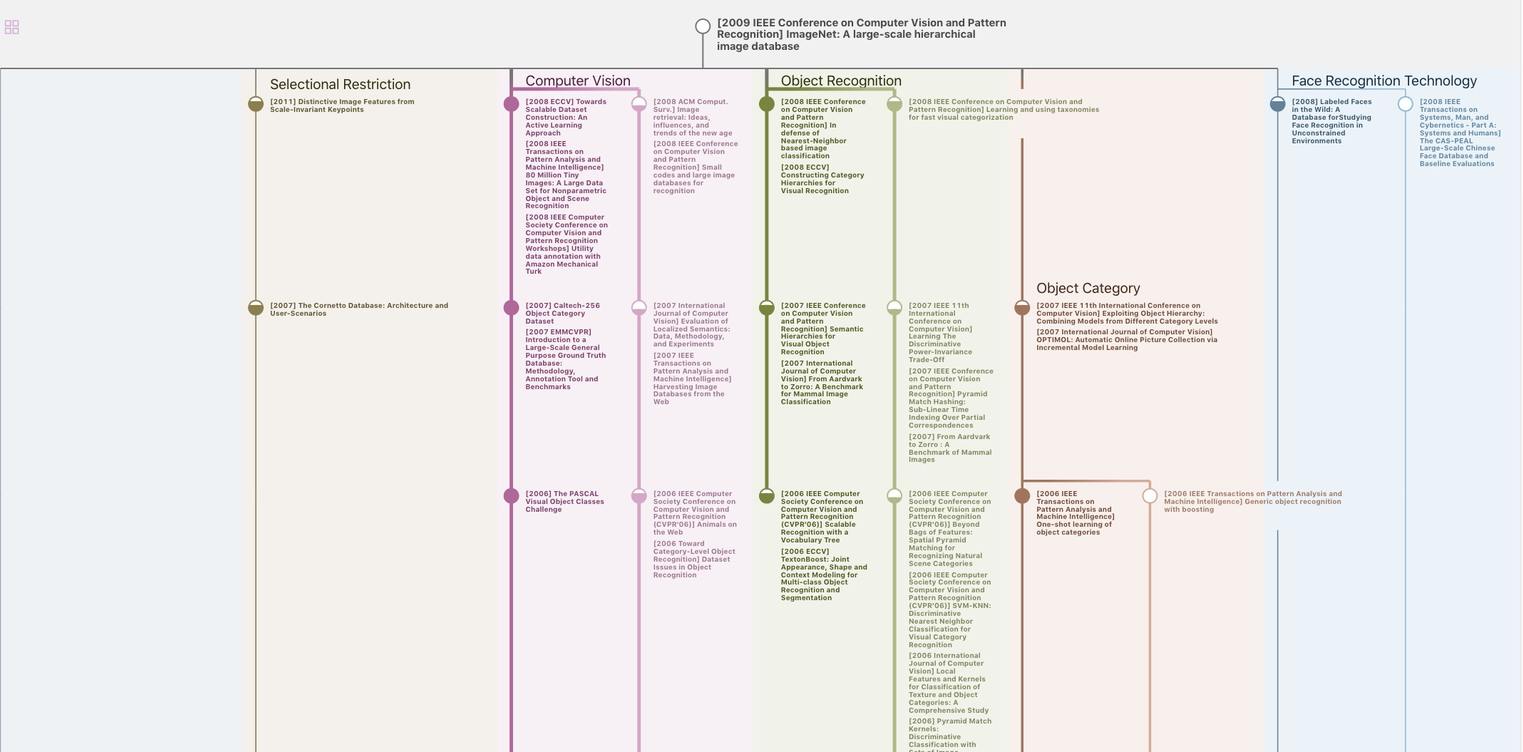
生成溯源树,研究论文发展脉络
Chat Paper
正在生成论文摘要