Autonomous Experiments for Neutron Three-Axis Spectrometers (TAS) with Log-Gaussian Processes
arxiv(2021)
摘要
Autonomous experiments are excellent tools to increase the efficiency of material discovery. Indeed, AI and ML methods can help optimizing valuable experimental resources as, for example, beam time in neutron scattering experiments, in addition to scientists' knowledge and experience. Active learning methods form a particular class of techniques that acquire knowledge on a specific quantity of interest by autonomous decisions on what or where to investigate next based on previous measurements. For instance, Gaussian Process Regression (GPR) is a well-known technique that can be exploited to accomplish active learning tasks for scattering experiments as was recently demonstrated. Gaussian processes are not only capable to approximate functions by their posterior mean function, but can also quantify uncertainty about the approximation itself. Hence, if we perform function evaluations at locations of highest uncertainty, the function can be "optimally" learned in an iterative manner. We suggest the use of log-Gaussian processes, being a natural approach to successfully conduct autonomous neutron scattering experiments in general and TAS experiments with the instrument PANDA at MLZ in particular.
更多查看译文
AI 理解论文
溯源树
样例
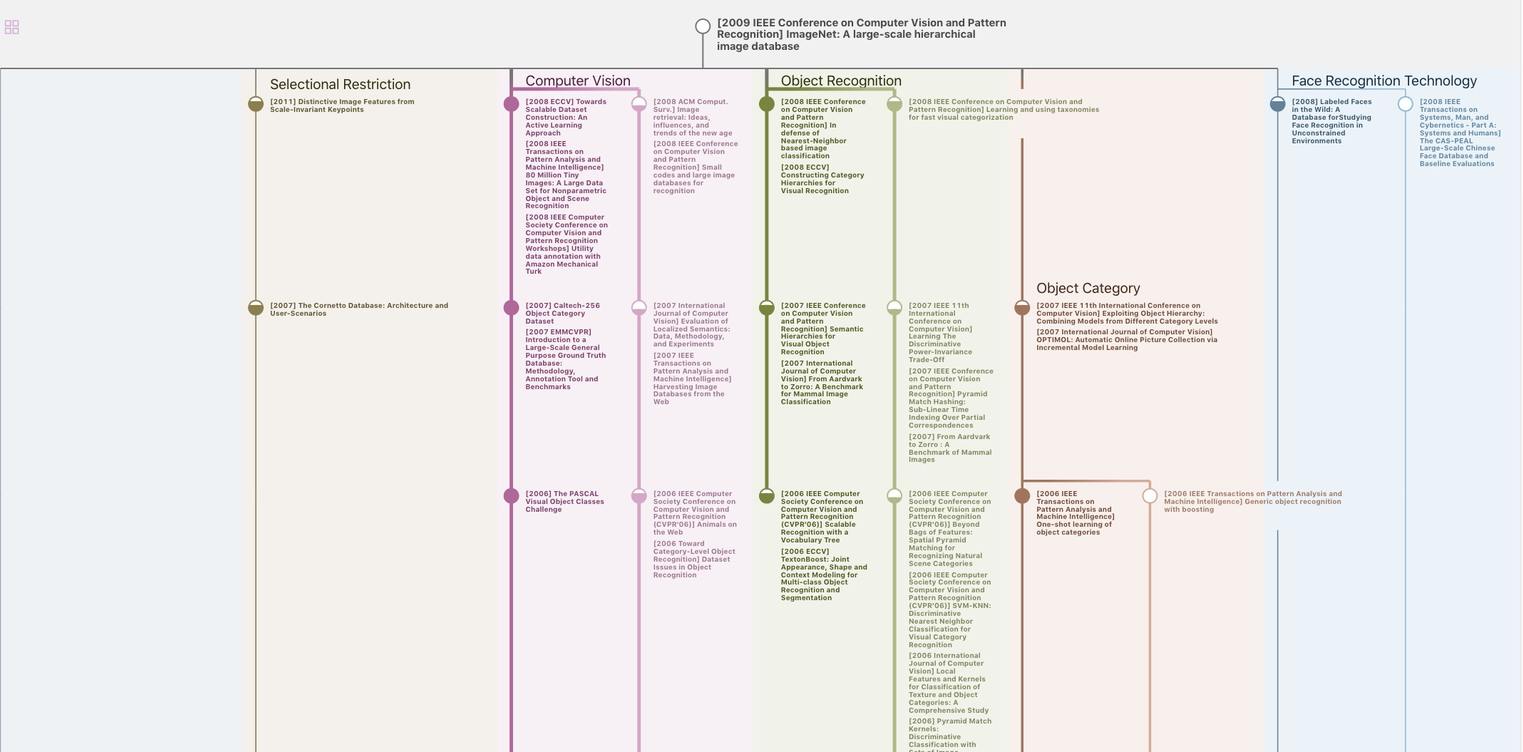
生成溯源树,研究论文发展脉络
Chat Paper
正在生成论文摘要