Evaluating the Robustness of Self-Supervised Learning in Medical Imaging
arxiv(2021)
摘要
Self-supervision has demonstrated to be an effective learning strategy when training target tasks on small annotated data-sets. While current research focuses on creating novel pretext tasks to learn meaningful and reusable representations for the target task, these efforts obtain marginal performance gains compared to fully-supervised learning. Meanwhile, little attention has been given to study the robustness of networks trained in a self-supervised manner. In this work, we demonstrate that networks trained via self-supervised learning have superior robustness and generalizability compared to fully-supervised learning in the context of medical imaging. Our experiments on pneumonia detection in X-rays and multi-organ segmentation in CT yield consistent results exposing the hidden benefits of self-supervision for learning robust feature representations.
更多查看译文
关键词
medical imaging,learning,robustness,self-supervised
AI 理解论文
溯源树
样例
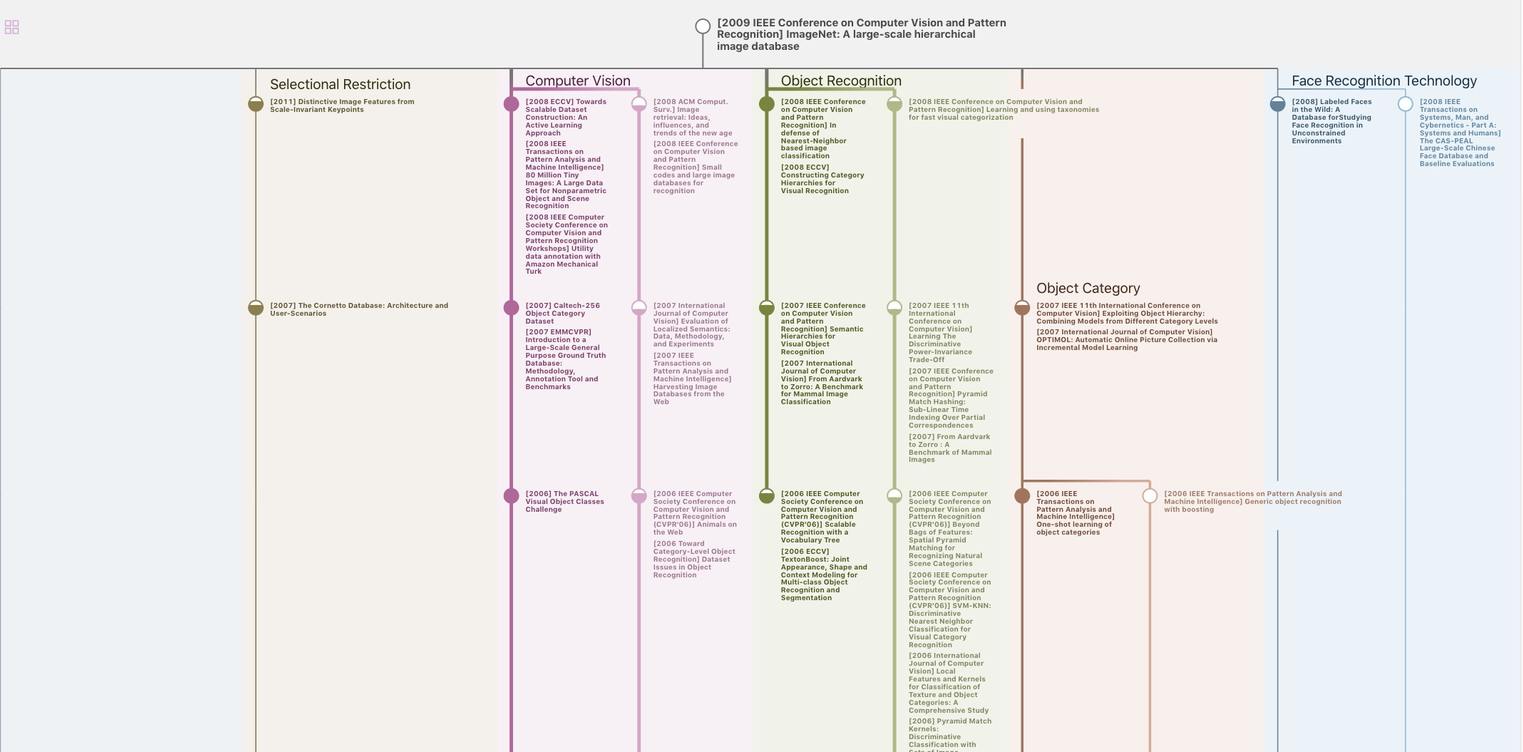
生成溯源树,研究论文发展脉络
Chat Paper
正在生成论文摘要