Posterior Regularization on Bayesian Hierarchical Mixture Clustering
arxiv(2022)
摘要
Bayesian hierarchical mixture clustering (BHMC) improves on the traditional Bayesian hierarchical clustering by, with regard to the parent-to-child diffusion in the generative process, replacing the conventional Gaussian-to-Gaussian (G2G) kernels with a Hierarchical Dirichlet Process Mixture Model (HDPMM). However, the drawback of the BHMC lies in the possibility of obtaining trees with comparatively high nodal variance in the higher levels (i.e., those closer to the root node). This can be interpreted as that the separation between the nodes, particularly those in the higher levels, might be weak. We attempt to overcome this drawback through a recent inferential framework named posterior regularization, which facilitates a simple manner to impose extra constraints on a Bayesian model to address its weakness. To enhance the separation of clusters, we apply posterior regularization to impose max-margin constraints on the nodes at every level of the hierarchy. In this paper, we illustrate the modeling detail of applying the PR on BHMC and show that this solution achieves the desired improvements over the BHMC model.
更多查看译文
关键词
hierarchical mixture clustering,posterior regularization,bayesian
AI 理解论文
溯源树
样例
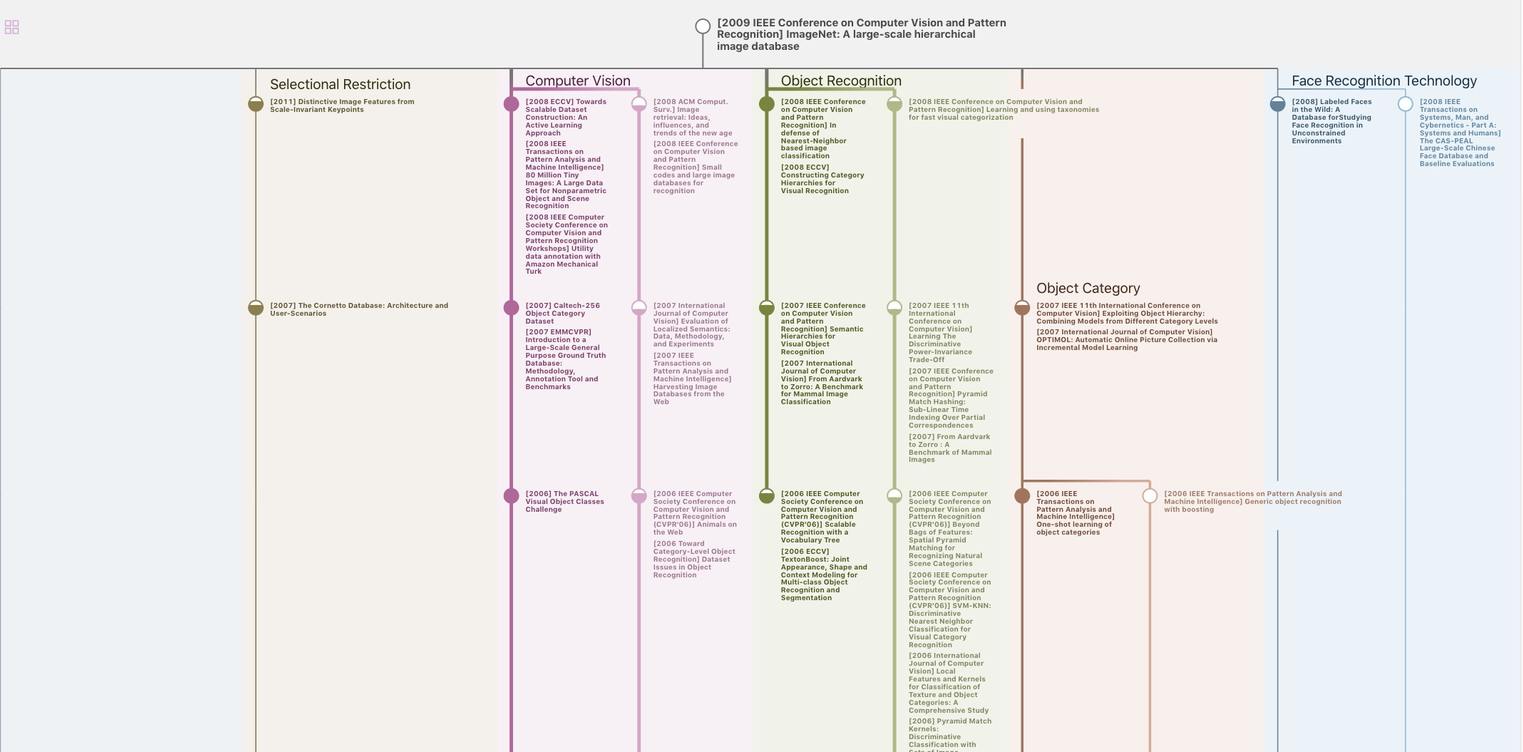
生成溯源树,研究论文发展脉络
Chat Paper
正在生成论文摘要