Policy Optimization in Dynamic Bayesian Network Hybrid Models of Biomanufacturing Processes
arxiv(2022)
摘要
Biopharmaceutical manufacturing is a rapidly growing industry with impact in virtually all branches of medicines. Biomanufacturing processes require close monitoring and control, in the presence of complex bioprocess dynamics with many interdependent factors, as well as extremely limited data due to the high cost of experiments as well as the novelty of personalized bio-drugs. We develop a novel model-based reinforcement learning framework that can achieve human-level control in low-data environments. The model uses a dynamic Bayesian network to capture causal interdependencies between factors and predict how the effects of different inputs propagate through the pathways of the bioprocess mechanisms. This enables the design of process control policies that are both interpretable and robust against model risk. We present a computationally efficient, provably convergence stochastic gradient method for optimizing such policies. Validation is conducted on a realistic application with a multi-dimensional, continuous state variable.
更多查看译文
关键词
biomanufacturing,reinforcement learning,policy optimization,Bayesian networks,bioprocess hybrid model
AI 理解论文
溯源树
样例
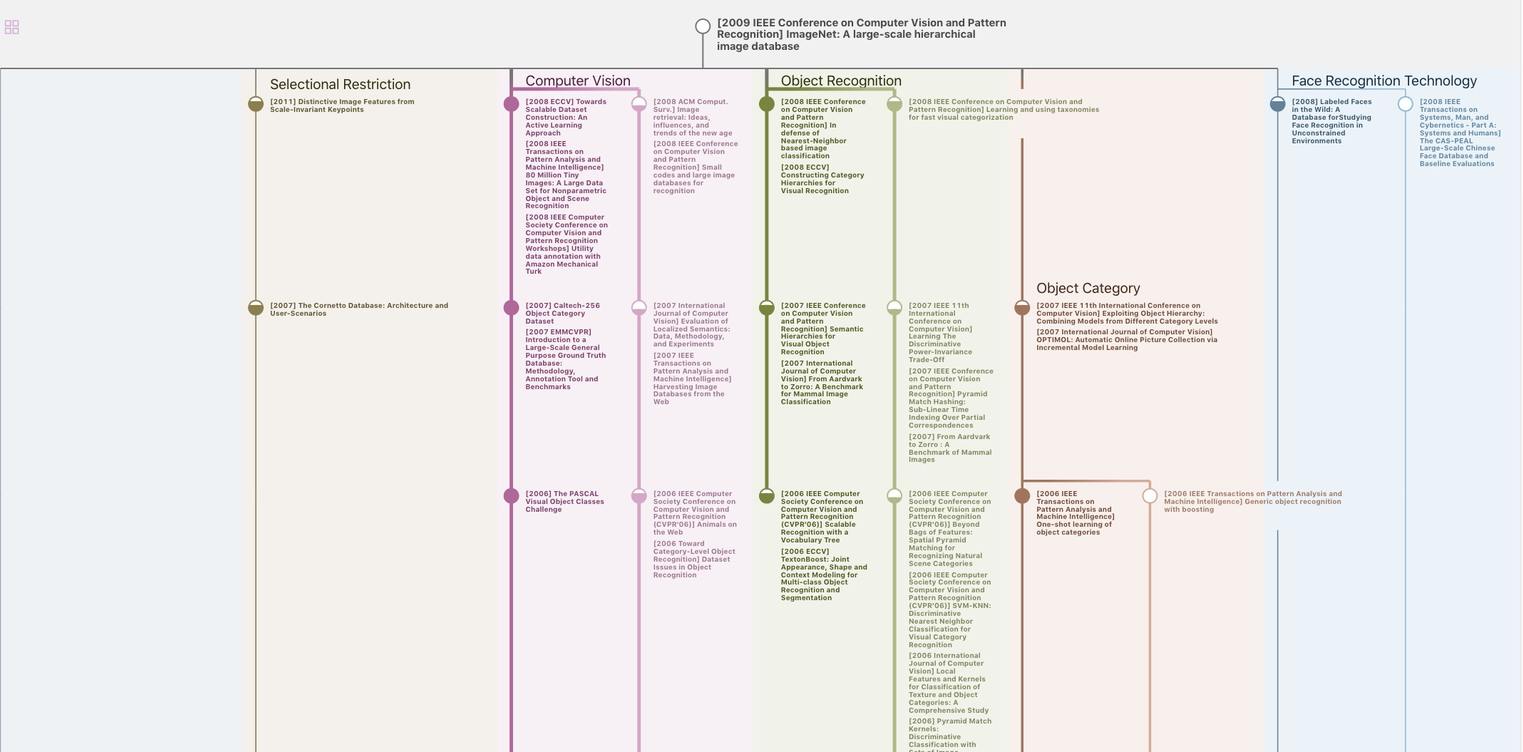
生成溯源树,研究论文发展脉络
Chat Paper
正在生成论文摘要