A Model-Based Validation Study Of Postoperative Complications With Considerations On Operative Timing
ANNALS OF TRANSLATIONAL MEDICINE(2021)
摘要
Background: Surgery is a highly technical procedure relying on high mental acuity and manual dexterity. The possibility that surgical outcomes and post-operative complications could be subject to influence by fatigue and/or circadian rhythms in surgeons has been investigated with inconsistent results.Methods: We conducted a retrospective study to assess the significance of operative timing on classifying surgical complications using an interpretable machine learning approach. We trained various linear, generative as well as tree models on the surgical record data collected from a university-affiliated, tertiary teaching hospital in China by performing parameter tuning using grid search cross-validation for optimizing the F1 score.Results: The results indicated that XGBoost was the best-performing model overall and its feature importance was shown to provide insight into possible timing-related associations with postoperative complications. We observed that the duration of surgery acted as the strongest indicator, and while surgery initiated at night (between 9 pm and 7 am) also ranked higher on the feature importance scale, it bore less significance than other factors such as the patient's age, gender, and type of surgery performed.Conclusions: We showed that surgical records could be used to demonstrate that operative timing might affect the occurrence of postoperative complications, but only in a relatively mild way while potentially entangling with multiple factors.
更多查看译文
关键词
Postoperative complication, circadian rhythm, machine learning, cross validation, XGBoost, feature importance
AI 理解论文
溯源树
样例
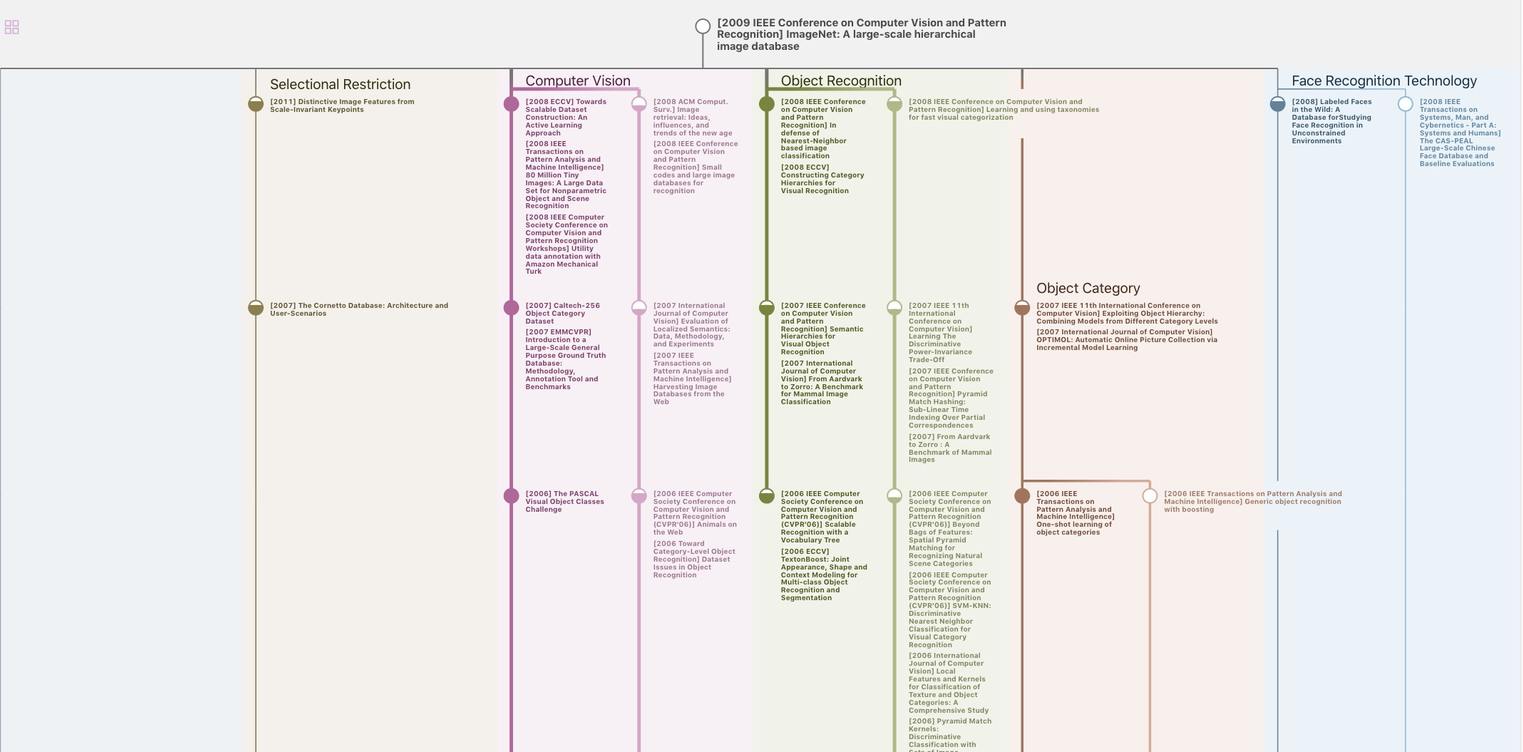
生成溯源树,研究论文发展脉络
Chat Paper
正在生成论文摘要