FlipReID: Closing the Gap Between Training and Inference in Person Re-Identification
2021 9th European Workshop on Visual Information Processing (EUVIP)(2021)
摘要
Since neural networks are data-hungry, incorporating data augmentation in training is a widely adopted technique that enlarges datasets and improves generalization. On the other hand, aggregating predictions of multiple augmented samples (i.e., test-time augmentation) could boost performance even further. In the context of person re-identification models, it is common practice to extract embeddings for both the original images and their horizontally flipped variants. The final representation is the mean of the aforementioned feature vectors. However, such scheme results in a gap between training and inference, i.e., the mean feature vectors calculated in inference are not part of the training pipeline. In this study, we devise the FlipReID structure with the flipping loss to address this issue. More specifically, models using the FlipReID structure are trained on the original images and the flipped images simultaneously, and incorporating the flipping loss minimizes the mean squared error between feature vectors of corresponding image pairs. Extensive experiments show that our method brings consistent improvements. In particular, we set a new record for MSMT17 which is the largest person re-identification dataset. The source code is available at https://github.com/nixingyang/FlipReID.
更多查看译文
关键词
Person re-identification,test-time augmentation
AI 理解论文
溯源树
样例
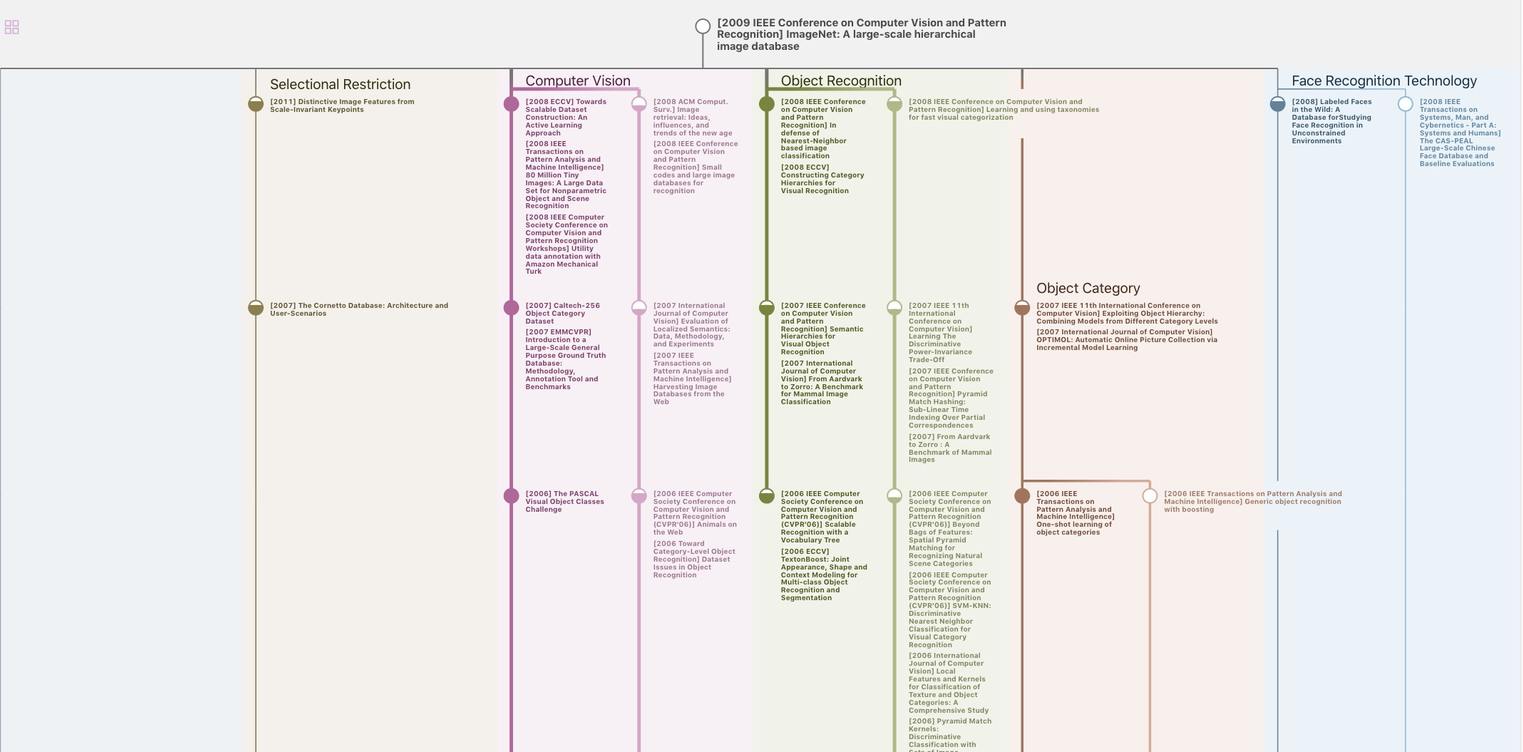
生成溯源树,研究论文发展脉络
Chat Paper
正在生成论文摘要