Cross-Modal and Multimodal Data Analysis Based on Functional Mapping of Spectral Descriptors and Manifold Regularization
arxiv(2021)
摘要
Multimodal manifold modeling methods extend the spectral geometry-aware data analysis to learning from several related and complementary modalities. Most of these methods work based on two major assumptions: 1) there are the same number of homogeneous data samples in each modality, and 2) at least partial correspondences between modalities are given in advance as prior knowledge. This work proposes two new multimodal modeling methods. The first method establishes a general analyzing framework to deal with the multimodal information problem for heterogeneous data without any specific prior knowledge. For this purpose, first, we identify the localities of each manifold by extracting local descriptors via spectral graph wavelet signatures (SGWS). Then, we propose a manifold regularization framework based on the functional mapping between SGWS descriptors (FMBSD) for finding the pointwise correspondences. The second method is a manifold regularized multimodal classification based on pointwise correspondences (M$^2$CPC) used for the problem of multiclass classification of multimodal heterogeneous, which the correspondences between modalities are determined based on the FMBSD method. The experimental results of evaluating the FMBSD method on three common cross-modal retrieval datasets and evaluating the (M$^2$CPC) method on three benchmark multimodal multiclass classification datasets indicate their effectiveness and superiority over state-of-the-art methods.
更多查看译文
关键词
multimodal data analysis,spectral descriptors,manifold regularization,functional mapping,cross-modal
AI 理解论文
溯源树
样例
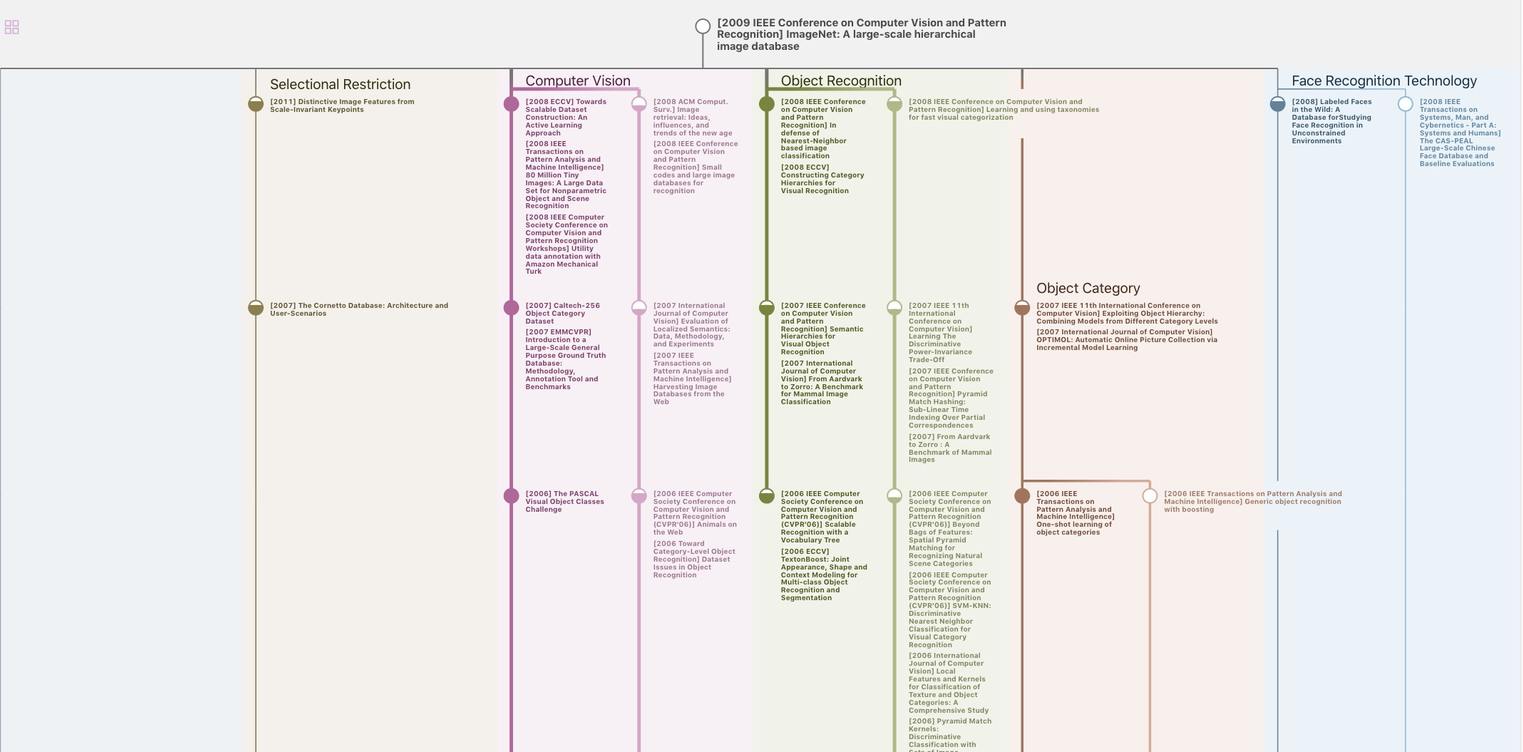
生成溯源树,研究论文发展脉络
Chat Paper
正在生成论文摘要